Subnetwork ensembling and data augmentation: Effects on calibration
EXPERT SYSTEMS(2023)
摘要
Deep Learning models based on convolutional neural networks are known to be uncalibrated, that is, they are either overconfident or underconfident in their predictions. Safety-critical applications of neural networks, however, require models to be well-calibrated, and there are various methods in the literature to increase model performance and calibration. Subnetwork ensembling is based on the over-parametrization of modern neural networks by fitting several subnetworks into a single network to take advantage of ensembling them without additional computational costs. Data augmentation methods have also been shown to enhance model performance in terms of accuracy and calibration. However, ensembling and data augmentation seem orthogonal to each other, and the total effect of combining these two methods is not well-known; the literature in fact is inconsistent. Through an extensive set of empirical experiments, we show that combining subnetwork ensemble methods with data augmentation methods does not degrade model calibration.
更多查看译文
关键词
calibration,data augmentation,ensembles
AI 理解论文
溯源树
样例
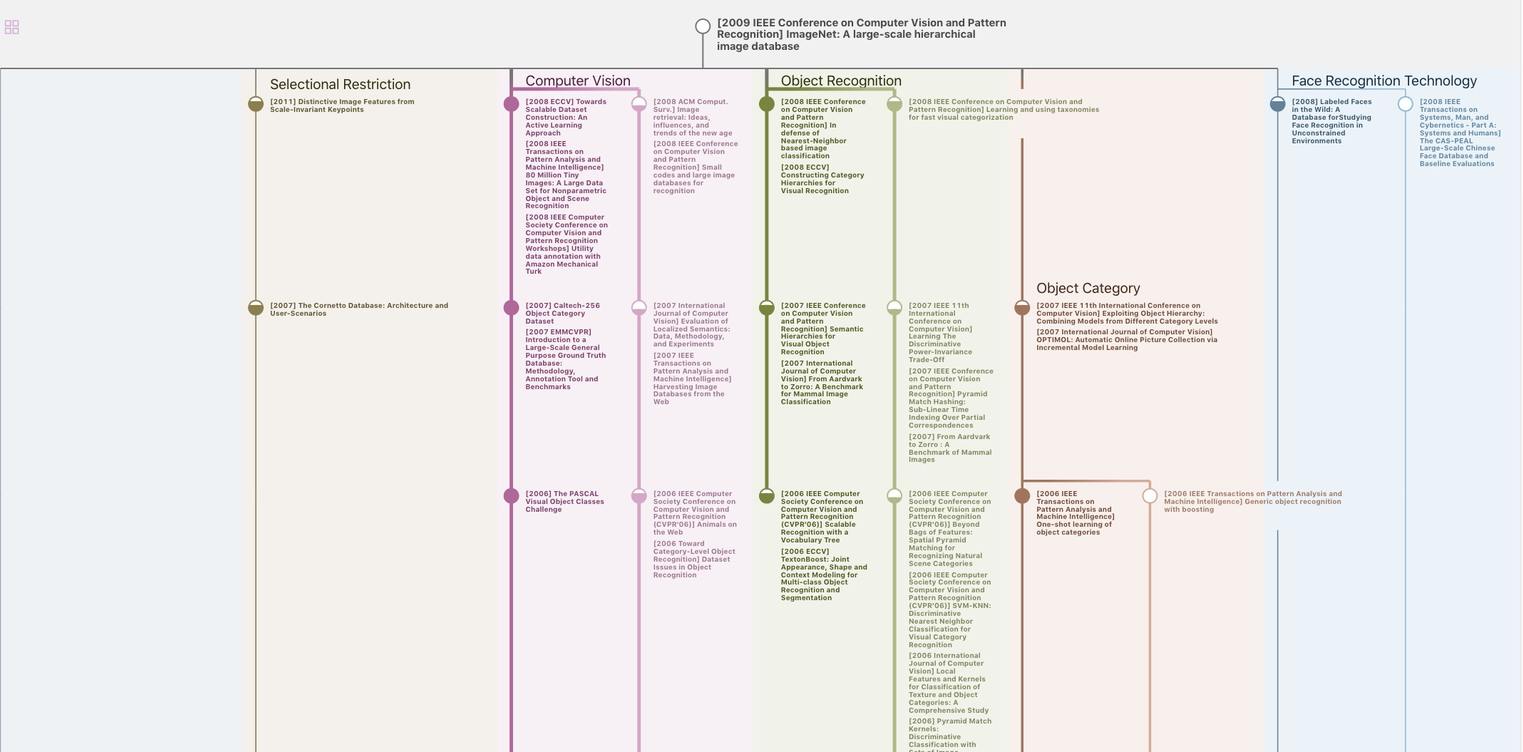
生成溯源树,研究论文发展脉络
Chat Paper
正在生成论文摘要