Deep Learning-Based Network Intrusion Detection Using Multiple Image Transformers
APPLIED SCIENCES-BASEL(2023)
摘要
The development of computer vision-based deep learning models for accurate two-dimensional (2D) image classification has enabled us to surpass existing machine learning-based classifiers and human classification capabilities. Recently, steady efforts have been made to apply these sophisticated vision-based deep learning models as network intrusion detection domains, and various experimental results have confirmed their applicability and limitations. In this paper, we present an optimized method for processing network intrusion detection system (NIDS) datasets using vision-based deep learning models by further expanding existing studies to overcome these limitations. In the proposed method, the NIDS dataset can further enhance the performance of existing deep-learning-based intrusion detection by converting the dataset into 2D images through various image transformers and then integrating into three-channel RGB color images, unlike the existing method. Various performance evaluations confirm that the proposed method can significantly improve intrusion detection performance over the recent method using grayscale images, and existing NIDSs without the use of images. As network intrusion is increasingly evolving in complexity and variety, we anticipate that the intrusion detection algorithm outlined in this study will facilitate network security.
更多查看译文
关键词
network intrusion detection,multiple image transformers,deep learning,three-channel RGB color image
AI 理解论文
溯源树
样例
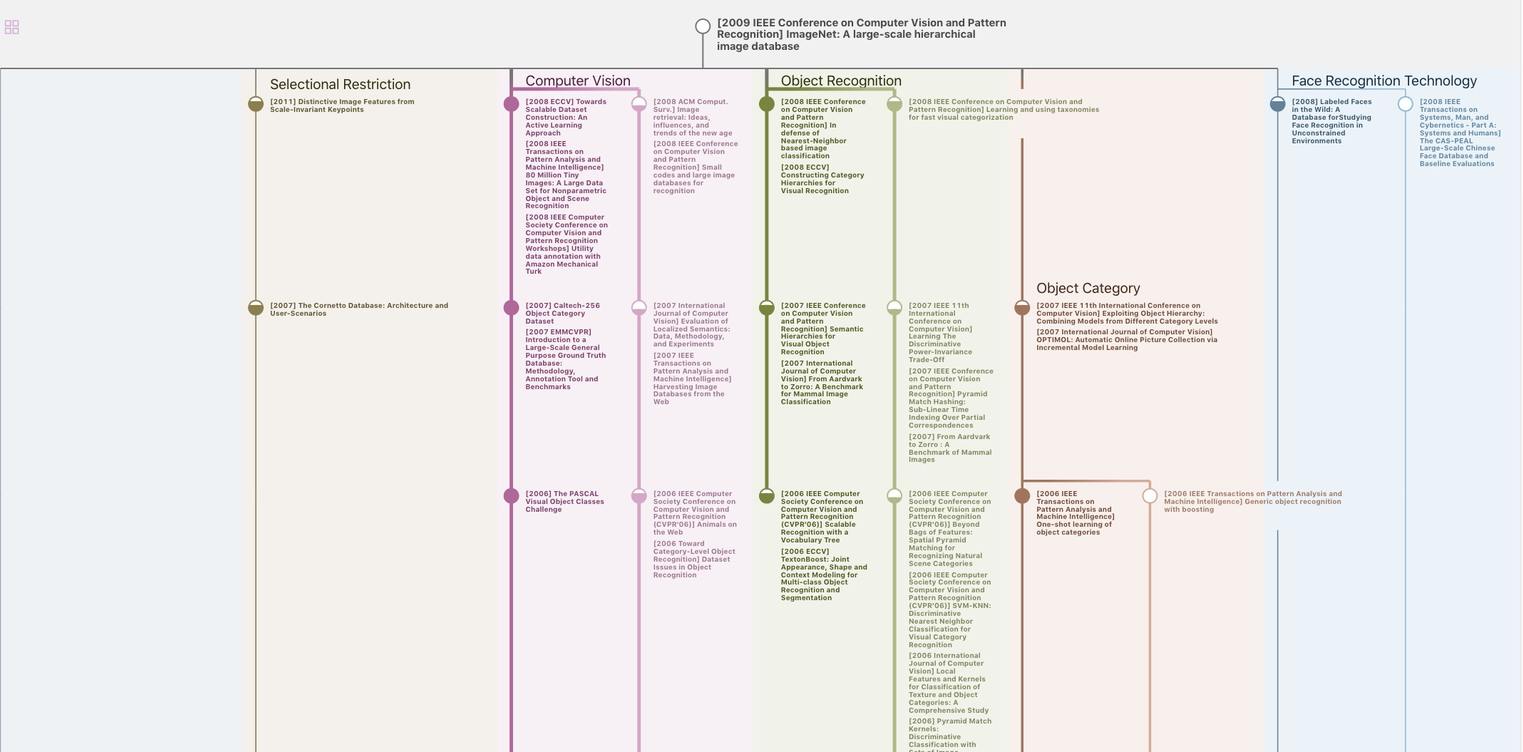
生成溯源树,研究论文发展脉络
Chat Paper
正在生成论文摘要