Graph-Augmentation-Free Self-Supervised Learning for Social Recommendation
APPLIED SCIENCES-BASEL(2023)
Abstract
Social recommendation systems can improve recommendation quality in cases of sparse user-item interaction data, which has attracted the industry's attention. In reality, social recommendation systems mostly mine real user preferences from social networks. However, trust relationships in social networks are complex and it is difficult to extract valuable user preference information, which worsens recommendation performance. To address this problem, this paper proposes a social recommendation algorithm based on multi-graph contrastive learning. To ensure the reliability of user preferences, the algorithm builds multiple enhanced user relationship views of the user's social network and encodes multi-view high-order relationship learning node representations using graph and hypergraph convolutional networks. Considering the effect of the long-tail phenomenon, graph-augmentation-free self-supervised learning is used as an auxiliary task to contrastively enhance node representations by adding uniform noise to each layer of encoder embeddings. Three open datasets were used to evaluate the algorithm, and it was compared to well-known recommendation systems. The experimental studies demonstrated the superiority of the algorithm.
MoreTranslated text
Key words
social recommendation,self-supervised learning,graph convolutional network
AI Read Science
Must-Reading Tree
Example
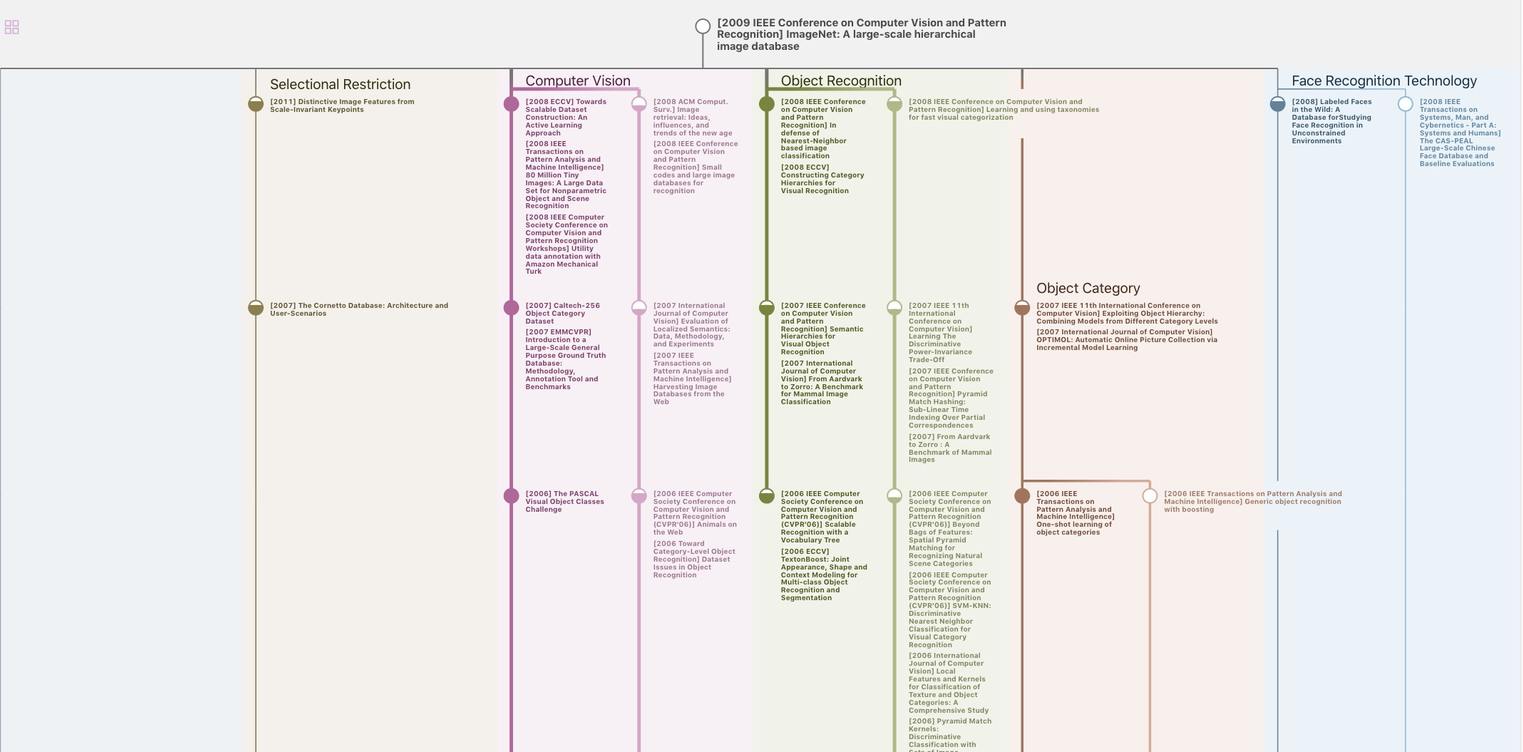
Generate MRT to find the research sequence of this paper
Chat Paper
Summary is being generated by the instructions you defined