Model and application of annual river runoff prediction based on complementary set empirical mode decomposition combined with particle swarm optimization adaptive neuro-fuzzy system
WATER SUPPLY(2023)
摘要
Runoff is affected by natural and nonnatural factors in the process of formation, and the runoff series is generally nonstationary time series. How to improve the accuracy of runoff prediction has always been a difficult problem for hydrologists. The key to solve this problem is to reduce the complexity of runoff series and improve the accuracy of runoff prediction model. Based on the aforementioned ideas, this article uses the complementary set empirical mode decomposition to decompose the runoff series into multiple intrinsic components that retain time-frequency information, thus reducing the complexity of the runoff series. The particle swarm optimization (PSO) adaptive neuro-fuzzy system is used to predict each intrinsic component to improve the accuracy of runoff prediction. After that, the trained intrinsic components of the model are reconstructed into the original runoff series. The example shows that the absolute relative error of the runoff forecasting model constructed in this article is 0.039, and the determination coefficient is 0.973. This model can be applied to the annual runoff series forecasting. Comparing the prediction results of this model with empirical mode decomposition algorithm-ANFIS model and ANFIS model, complementary set empirical mode decomposition algorithm-PSO-ANFIS model shows obvious advantages.
更多查看译文
关键词
adaptive neuro-fuzzy system,annual runoff,complementary set empirical mode decomposition,forecast accuracy,runoff forecast
AI 理解论文
溯源树
样例
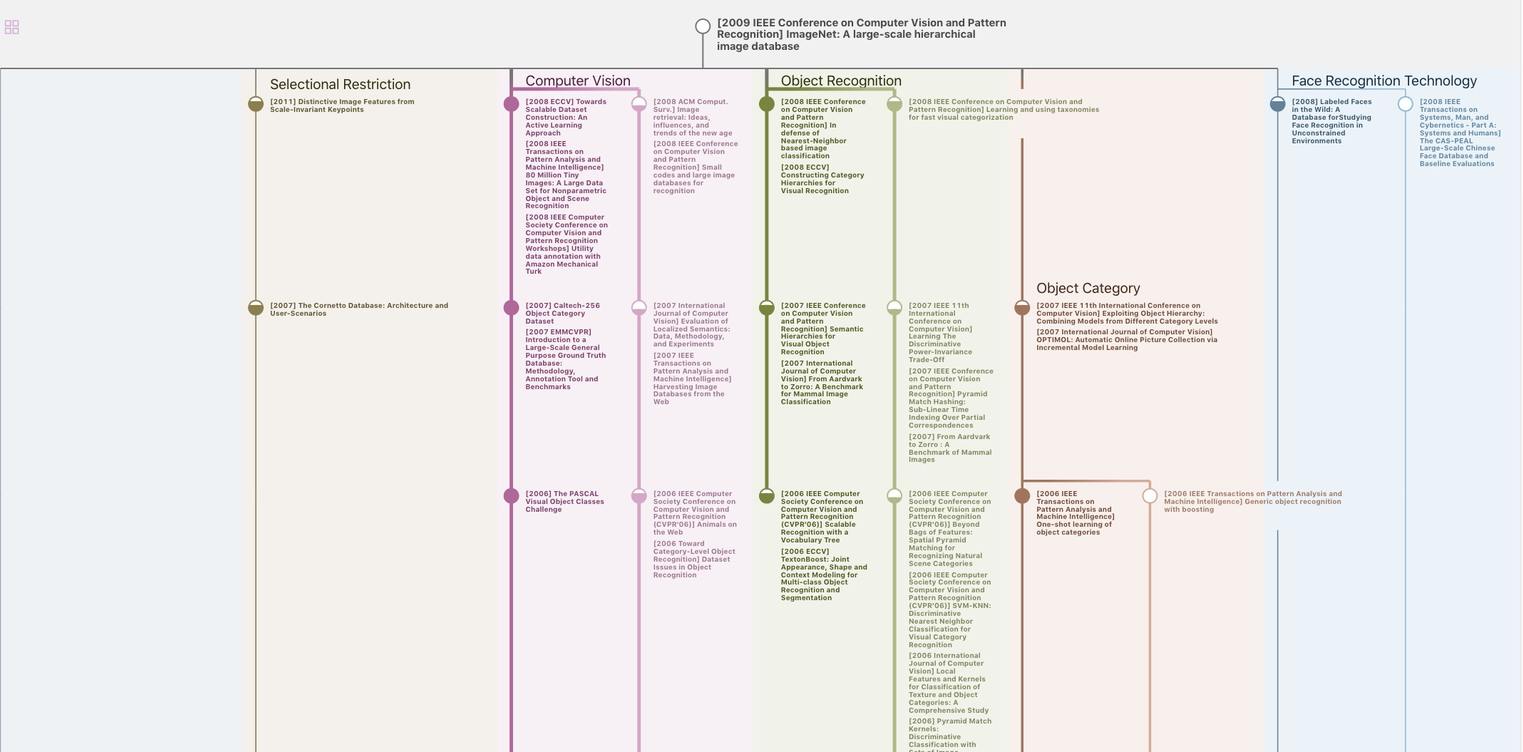
生成溯源树,研究论文发展脉络
Chat Paper
正在生成论文摘要