Constraining Bedrock Groundwater Residence Times in a Mountain System With Environmental Tracer Observations and Bayesian Uncertainty Quantification
WATER RESOURCES RESEARCH(2023)
摘要
Groundwater residence time distributions provide fundamental insights on the hydrological processes within watersheds. Yet, observations that can constrain groundwater residence times over broad timescales remain scarce in mountain catchment studies. We use environmental tracers (CFC-12, SF6, H-3, and He-4) to investigate groundwater residence times along a hillslope in the East River Watershed, Colorado, USA. We develop a Bayesian inference framework that applies a Markov-chain Monte Carlo (MCMC) approach to estimate noble gas recharge temperature, elevation, and excess-air parameters and the resulting environmental tracer concentrations. MCMC is then used to propagate the environmental tracer uncertainties to estimates of groundwater mean residence times inferred with lumped parameter models. All samples contain H-3, CFC-12, and SF6 in addition to terrigenic He-4, suggesting a mixture of water characterized by modern and premodern residence times. He-4 exponential mean residence times range from hundreds of years at the upslope well to thousands of years at the toe-slope well assuming average crustal production rates. We find that binary mixing residence time distributions with separate young and old mixing fractions are needed to predict the He-4, CFC-12, SF6, and H-3 observations, supporting the importance of flow path mixing in this bedrock system. Our findings that the fractured bedrock hosts groundwater with a mixture of residence times ranging from decades to millennia suggest variable recharge dynamics and flow path mixing along the hillslope and highlight the importance of characterizing groundwater systems with observations that are sensitive to transport over a broad range of residence times.
更多查看译文
关键词
groundwater residence times,environmental tracers,uncertainty analysis,catchment hydrology,dissolved noble gases
AI 理解论文
溯源树
样例
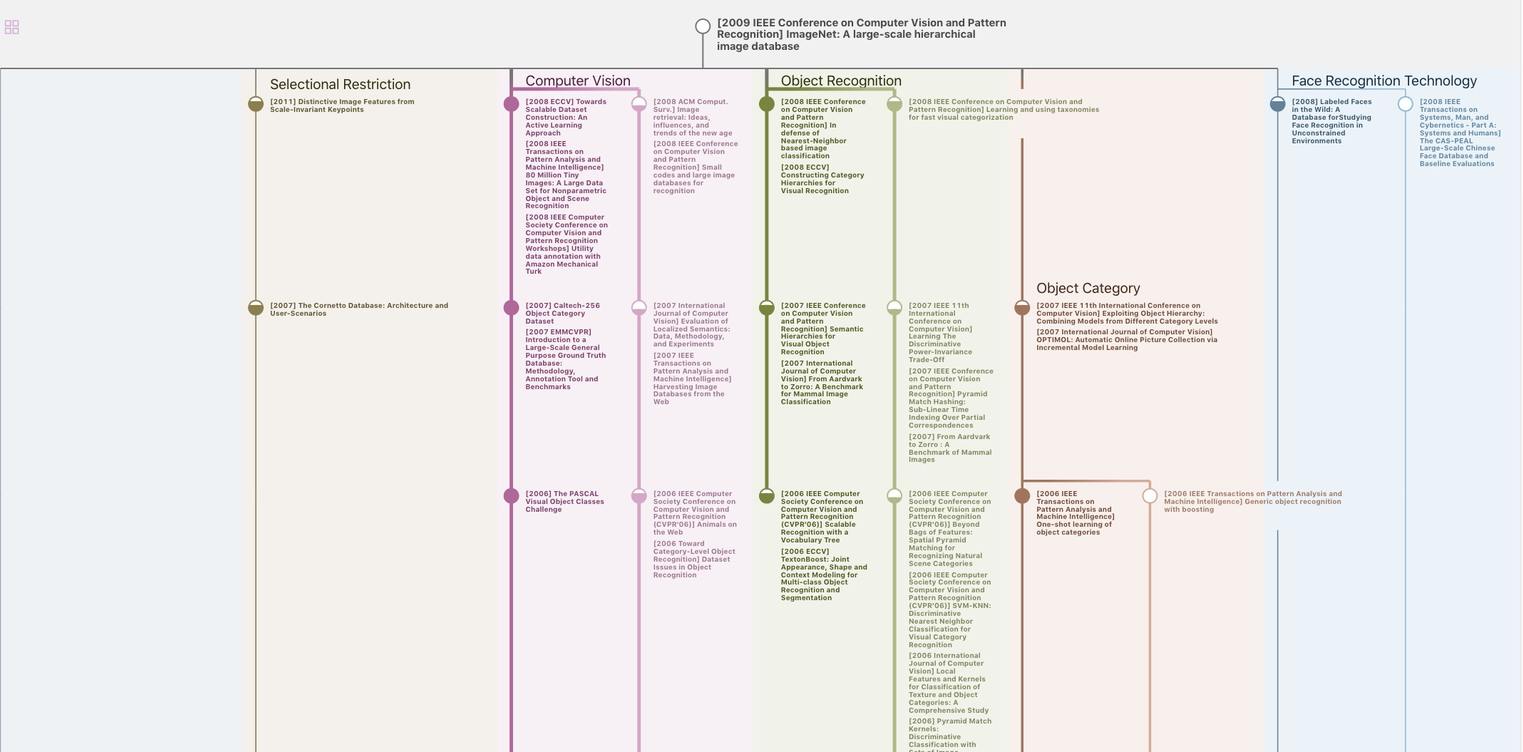
生成溯源树,研究论文发展脉络
Chat Paper
正在生成论文摘要