A Hybrid Deep Learning Model for Multi-Station Classification and Passenger Flow Prediction
APPLIED SCIENCES-BASEL(2023)
摘要
Multiple station passenger flow prediction is crucial but challenging for intelligent transportation systems. Recently, deep learning models have been widely applied in multi-station passenger flow prediction. However, flows at the same station in different periods, or different stations in the same period, always present different characteristics. These indicate that globally extracting spatio-temporal features for multi-station passenger flow prediction may only be powerful enough to achieve the excepted performance for some stations. Therefore, a novel two-step multi-station passenger flow prediction model is proposed. First, an unsupervised clustering method for station classification using pure passenger flow is proposed based on the Transformer encoder and K-Means. Two novel evaluation metrics are introduced to verify the effectiveness of the classification results. Then, based on the classification results, a passenger flow prediction model is proposed for every type of station. Residual network (ResNet) and graph convolution network (GCN) are applied for spatial feature extraction, and attention long short-term memory network (AttLSTM) is used for temporal feature extraction. Integrating results for every type of station creates a prediction model for all stations in the network. Experiments are conducted on two real-world ridership datasets. The proposed model performs better than unclassified results in multi-station passenger flow prediction.
更多查看译文
关键词
station classification,multi-station passenger flow prediction,transformer encoder,K-Means,GCN,attention LSTM
AI 理解论文
溯源树
样例
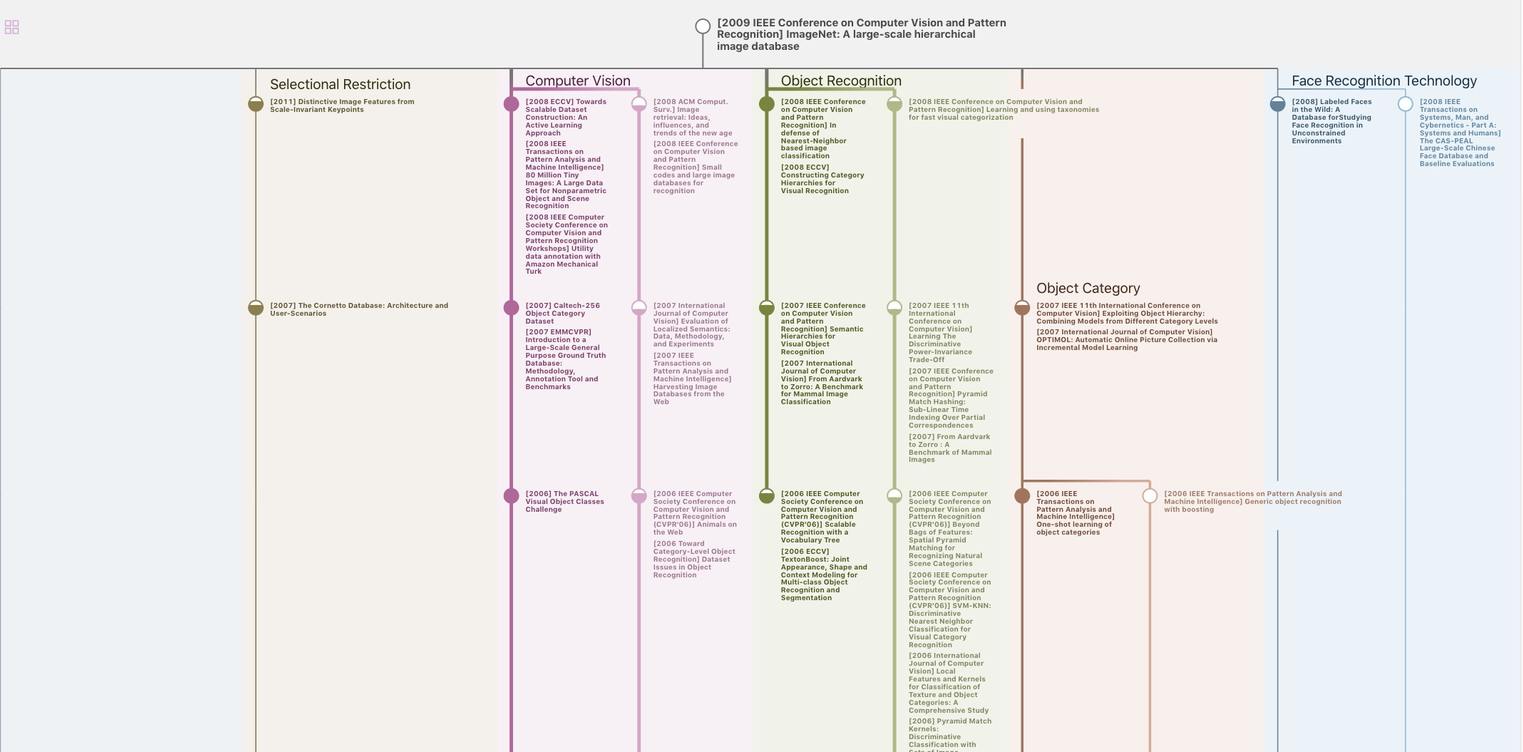
生成溯源树,研究论文发展脉络
Chat Paper
正在生成论文摘要