Gastrointestinal Diseases Classification Using Deep Transfer Learning and Features Optimization
CMC-COMPUTERS MATERIALS & CONTINUA(2023)
摘要
Gastrointestinal diseases like ulcers, polyps', and bleeding are increasing rapidly in the world over the last decade. On average 0.7 million cases are reported worldwide every year. The main cause of gastrointestinal diseases is a Helicobacter Pylori (H. Pylori) bacterium that presents in more than 50% of people around the globe. Many researchers have proposed different methods for gastrointestinal disease using computer vision tech-niques. Few of them focused on the detection process and the rest of them performed classification. The major challenges that they faced are the simi-larity of infected and healthy regions that misleads the correct classification accuracy. In this work, we proposed a technique based on Mask Recurrent -Convolutional Neural Network (R-CNN) and fine-tuned pre-trained ResNet-50 and ResNet-152 networks for feature extraction. Initially, the region of interest is detected using Mask R-CNN which is later utilized for the training of fine-tuned models through transfer learning. Features are extracted from fine-tuned models that are later fused using a serial approach. Moreover, an Improved Ant Colony Optimization (ACO) algorithm has also opted for the best feature selection from the fused feature vector. The best-selected features are finally classified using machine learning techniques. The experimental process was conducted on the publicly available dataset and obtained an improved accuracy of 96.43%. In comparison with state-of-the-art techniques, it is observed that the proposed accuracy is improved.
更多查看译文
关键词
Diseases,deep learning,endoscopy,gastrointestinal tract,transfer learning
AI 理解论文
溯源树
样例
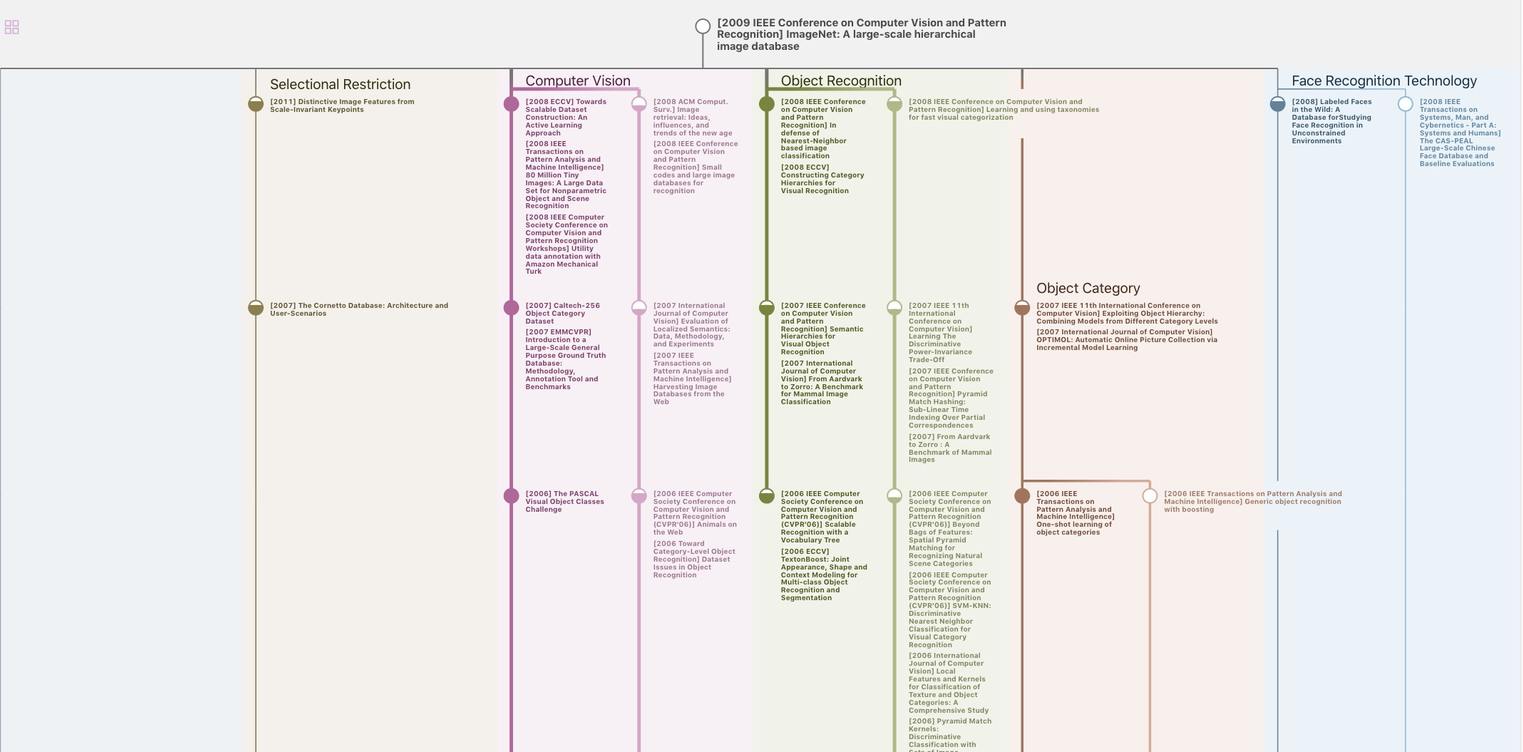
生成溯源树,研究论文发展脉络
Chat Paper
正在生成论文摘要