A CEP-driven framework for real-time news impact prediction on financial markets
SERVICE ORIENTED COMPUTING AND APPLICATIONS(2023)
摘要
Real-time news impact prediction on financial markets is a challenging task for finance experts with limited IT expertise. Many practitioners build machine learning models trained with many low-level features extracted from multiple event-based streams (news and financial market data), which often leads to poor outcomes. State-of-the-art solutions either ignore domain-specific contexts or are customised to merely one type of static datasets rather than real-time data streams. In most cases, the domain expert would have to manually conduct data collection, data cleaning and aggregation, and machine learning step by step with the assistance of IT experts, which is time-consuming and complicated. To address these limitations, we propose a technique that uses real-time data pre-processing in accordance with domain-specific event patterns to generate better-quality datasets. This technique is supported by a systematic framework featuring a data model capturing domain-specific event patterns, an SOA-based architecture and processes that integrate the capabilities of sentiment analysis, complex event processing with automated machine learning (AutoML), facilitating event pattern detection and continual learning with sliding time windows. The benefit of adopting an SOA architecture is to ensure the flexibility of the selection and seamless integration of components. This solution allows domain experts to define domain-specific event patterns via a user-friendly interface and prepare better-quality datasets by pre-processing real-time data streams accordingly via the complex event processing component, aiming to generate meaningful prediction results by the downstream AutoML component. The AutoML component allows for minimal machine learning skills by the domain expert to conduct the prediction tasks. A prototype was implemented to evaluate its feasibility and functionality on a real-life price movement prediction scenario involving 3 years of news and financial market data. The results demonstrate that finance experts are able to complete news impact prediction tasks in real time without the intervention of IT experts, which saves a large amount of time compared with traditional machine learning processes.
更多查看译文
关键词
Complex event processing,Machine learning,Real-time analytics,Data quality,Service-oriented architecture,Finance
AI 理解论文
溯源树
样例
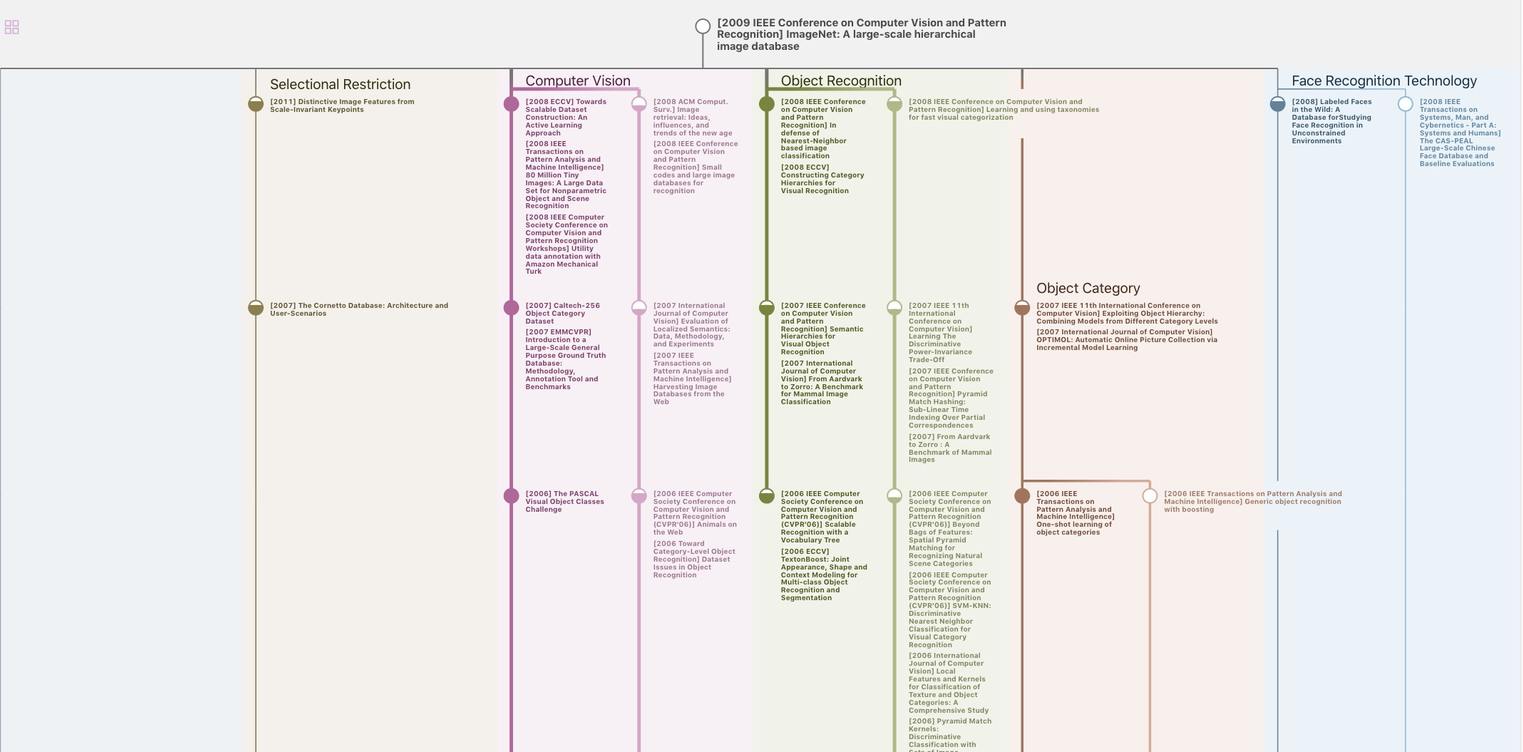
生成溯源树,研究论文发展脉络
Chat Paper
正在生成论文摘要