I Don’t Care How Popular You Are! Investigating Popularity Bias in Music Recommendations from a User’s Perspective
CHIIR '23: Proceedings of the 2023 Conference on Human Information Interaction and Retrieval(2023)
摘要
Recommender systems are designed to help us navigate through an abundance of online content. Collaborative filtering (CF) approaches are commonly used to leverage behaviors of others with a similar taste to make predictions for the target user. However, CF is prone to introduce or amplify popularity bias in which popular (often consumed or highly ranked) items are prioritized over less popular items. Many computational metrics of popularity biases — and resulting algorithmic (un)fairness — have been presented. However, it is largely unclear whether these metrics reflect human perception of bias and fairness. We conducted a user study with 170 participants to explore how users perceive recommendation lists created by algorithms with different degrees of popularity bias. Our results show — surprisingly — that popularity biases in recommendation lists are barely observed by users, even when corresponding bias/fairness metrics clearly indicate them.
更多查看译文
AI 理解论文
溯源树
样例
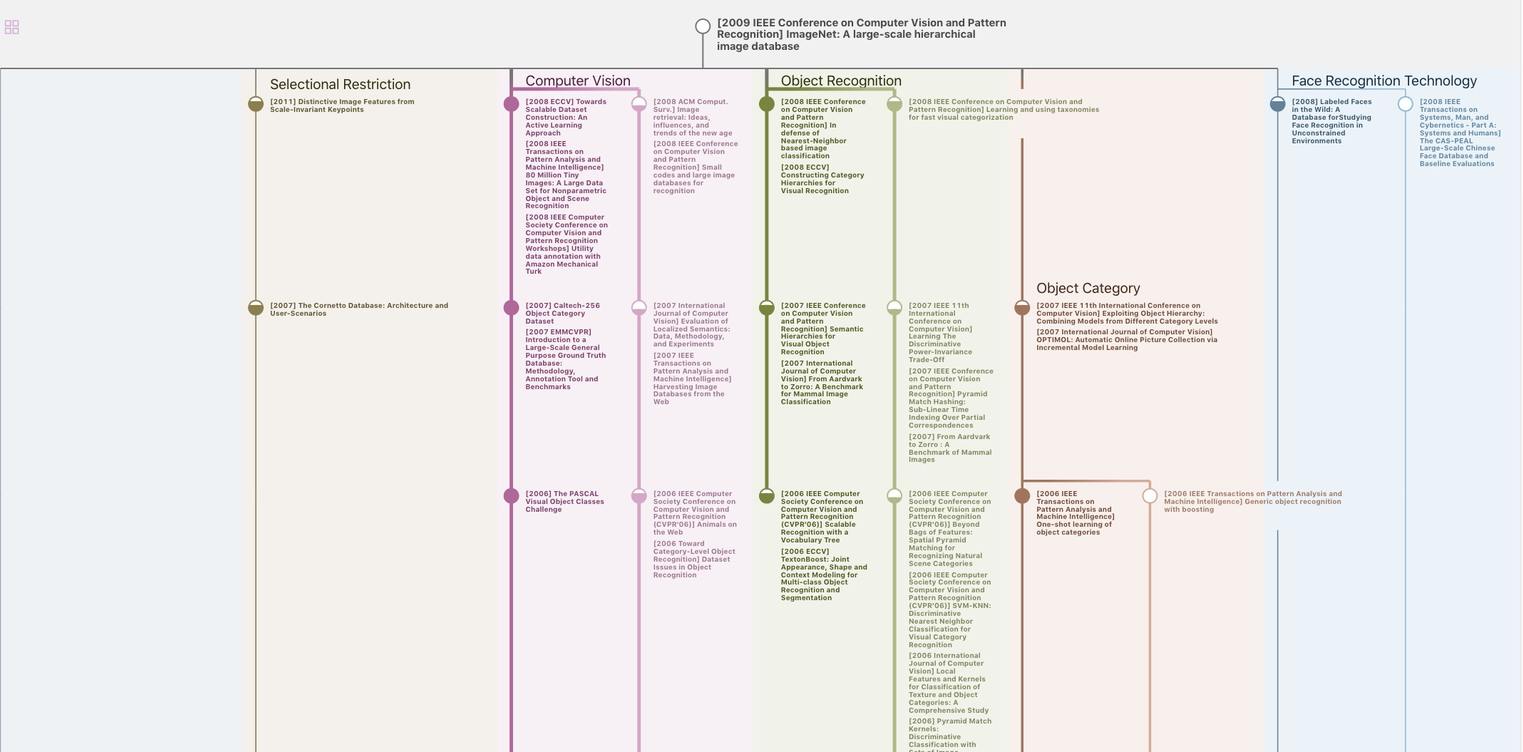
生成溯源树,研究论文发展脉络
Chat Paper
正在生成论文摘要