ENTL: Embodied Navigation Trajectory Learner
2023 IEEE/CVF International Conference on Computer Vision (ICCV)(2023)
Abstract
We propose Embodied Navigation Trajectory Learner (ENTL), a method for extracting long sequence representations for embodied navigation. Our approach unifies world modeling, localization and imitation learning into a single sequence prediction task. We train our model using vector-quantized predictions of future states conditioned on current states and actions. ENTL's generic architecture enables the sharing of the the spatio-temporal sequence encoder for multiple challenging embodied tasks. We achieve competitive performance on navigation tasks using significantly less data than strong baselines while performing auxiliary tasks such as localization and future frame prediction (a proxy for world modeling). A key property of our approach is that the model is pre-trained without any explicit reward signal, which makes the resulting model generalizable to multiple tasks and environments.
MoreTranslated text
Key words
Semantic Reasoning,Action Recognition,Motion Capture
AI Read Science
Must-Reading Tree
Example
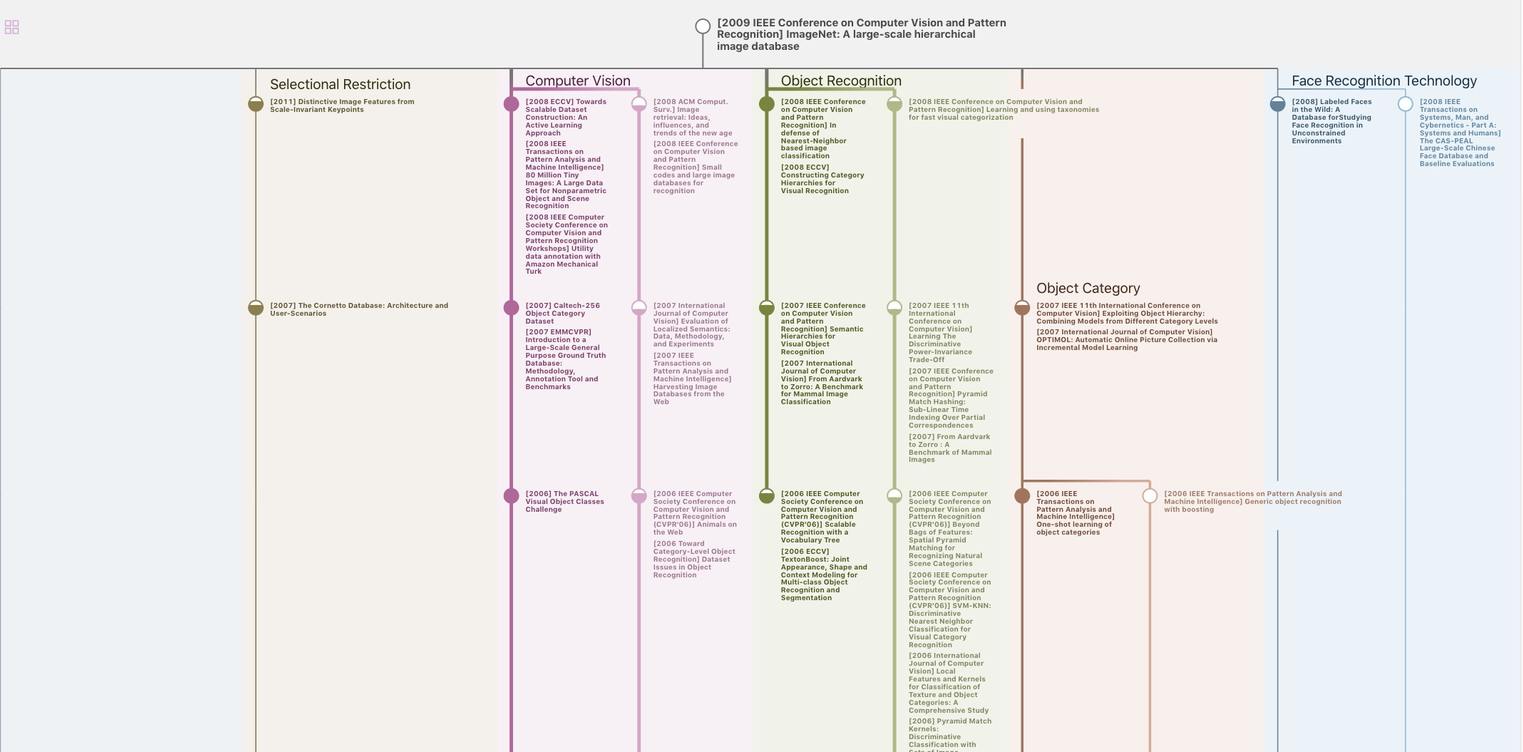
Generate MRT to find the research sequence of this paper
Chat Paper
Summary is being generated by the instructions you defined