A Dual System-Level Parameterization for Identification from Closed-Loop Data
2023 62ND IEEE CONFERENCE ON DECISION AND CONTROL, CDC(2023)
摘要
This work presents a dual system-level parameterization (D-SLP) method for closed-loop identification of linear time-invariant systems.The recent system-level synthesis framework parameterizes all stabilizing controllers via linear constraints on closed-loop response functions, known as system-level parameters. It was demonstrated that several structural, locality, and communication constraints on the controller can be posed as convex constraints on these system-level parameters. In the current work, the identification problem is treated as a dual of the system-level synthesis problem. The plant model is identified from the dual system-level parameters associated to the plant. In comparison to existing closed-loop identification approaches (such as the dual-Youla parameterization), the D-SLP framework neither requires the knowledge of a nominal plant that is stabilized by the known controller, nor depends upon the choice of factorization of the nominal plant and the stabilizing controller. Numerical simulations demonstrate the efficacy of the proposed D-SLP method in terms of identification errors, compared to existing closed-loop identification techniques.
更多查看译文
AI 理解论文
溯源树
样例
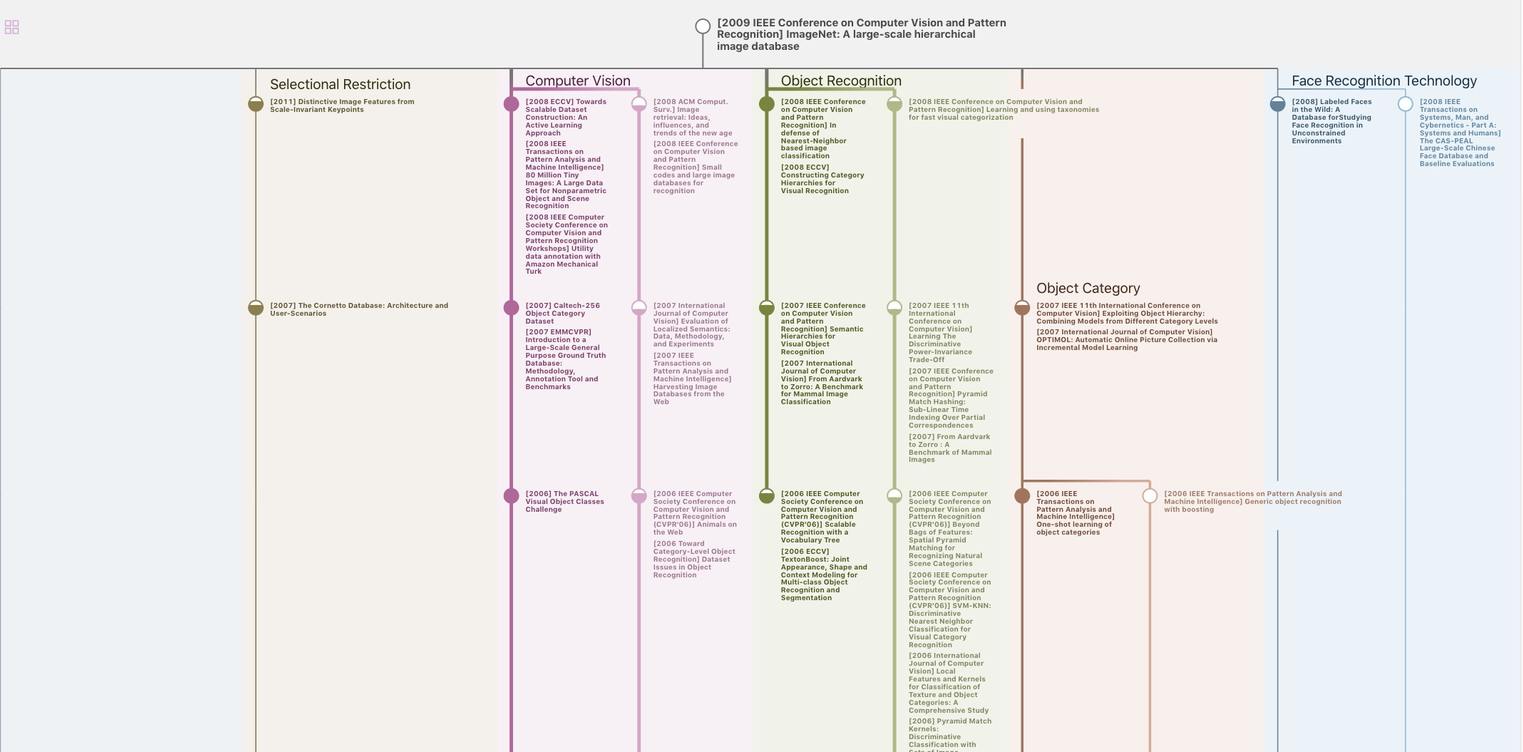
生成溯源树,研究论文发展脉络
Chat Paper
正在生成论文摘要