Enhancing Multimodal Entity and Relation Extraction With Variational Information Bottleneck
IEEE-ACM TRANSACTIONS ON AUDIO SPEECH AND LANGUAGE PROCESSING(2024)
摘要
This article studies the multimodal named entity recognition (MNER) and multimodal relation extraction (MRE), which are important for content analysis and various applications. The core of MNER and MRE lies in incorporating evident visual information to enhance textual semantics, where two issues inherently demand investigations. The first issue is modality-noise, where the task-irrelevant information in each modality may be noises misleading the task prediction. The second issue is modality-gap, where representations from different modalities are inconsistent, preventing from building the semantic alignment between the text and image. To address these issues, we propose a novel method for MNER and MRE by MultiModal representation learning with Information Bottleneck (MMIB). For the first issue, a refinement-regularizer probes the information-bottleneck principle to balance the predictive evidence and noisy information, yielding expressive representations for prediction. For the second issue, an alignment-regularizer is proposed, where a mutual information-based item works in a contrastive manner to regularize the consistent text-image representations. To our best knowledge, we are the first to explore variational IB estimation for MNER and MRE. Experiments show that MMIB achieves the state-of-the-art performances on three public benchmarks.
更多查看译文
关键词
Multimodal named entity recognition,multimodal relation extraction,information bottleneck
AI 理解论文
溯源树
样例
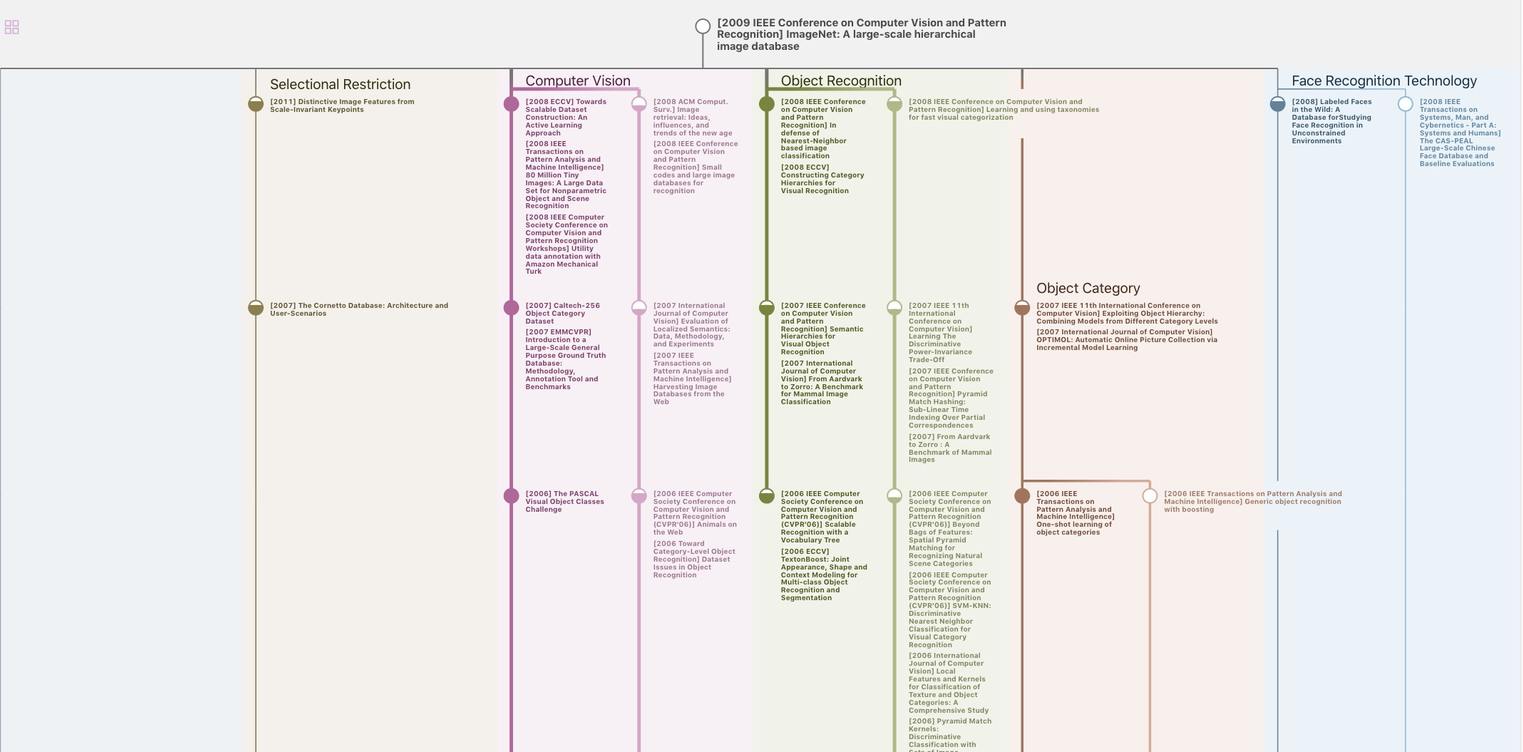
生成溯源树,研究论文发展脉络
Chat Paper
正在生成论文摘要