Gradient Attention Balance Network: Mitigating Face Recognition Racial Bias via Gradient Attention
CoRR(2023)
摘要
Although face recognition has made impressive progress in recent years, we ignore the racial bias of the recognition system when we pursue a high level of accuracy. Previous work found that for different races, face recognition networks focus on different facial regions, and the sensitive regions of darker-skinned people are much smaller. Based on this discovery, we propose a new de-bias method based on gradient attention, called Gradient Attention Balance Network (GABN). Specifically, we use the gradient attention map (GAM) of the face recognition network to track the sensitive facial regions and make the GAMs of different races tend to be consistent through adversarial learning. This method mitigates the bias by making the network focus on similar facial regions. In addition, we also use masks to erase the Top-N sensitive facial regions, forcing the network to allocate its attention to a larger facial region. This method expands the sensitive region of darker-skinned people and further reduces the gap between GAM of darker-skinned people and GAM of Caucasians. Extensive experiments show that GABN successfully mitigates racial bias in face recognition and learns more balanced performance for people of different races.
更多查看译文
关键词
darker-skinned people,de-bias method,face recognition network,face recognition racial bias,GAM,gradient attention balance network,gradient attention map,network focus,recognition system,sensitive facial regions,sensitive region,similar facial regions
AI 理解论文
溯源树
样例
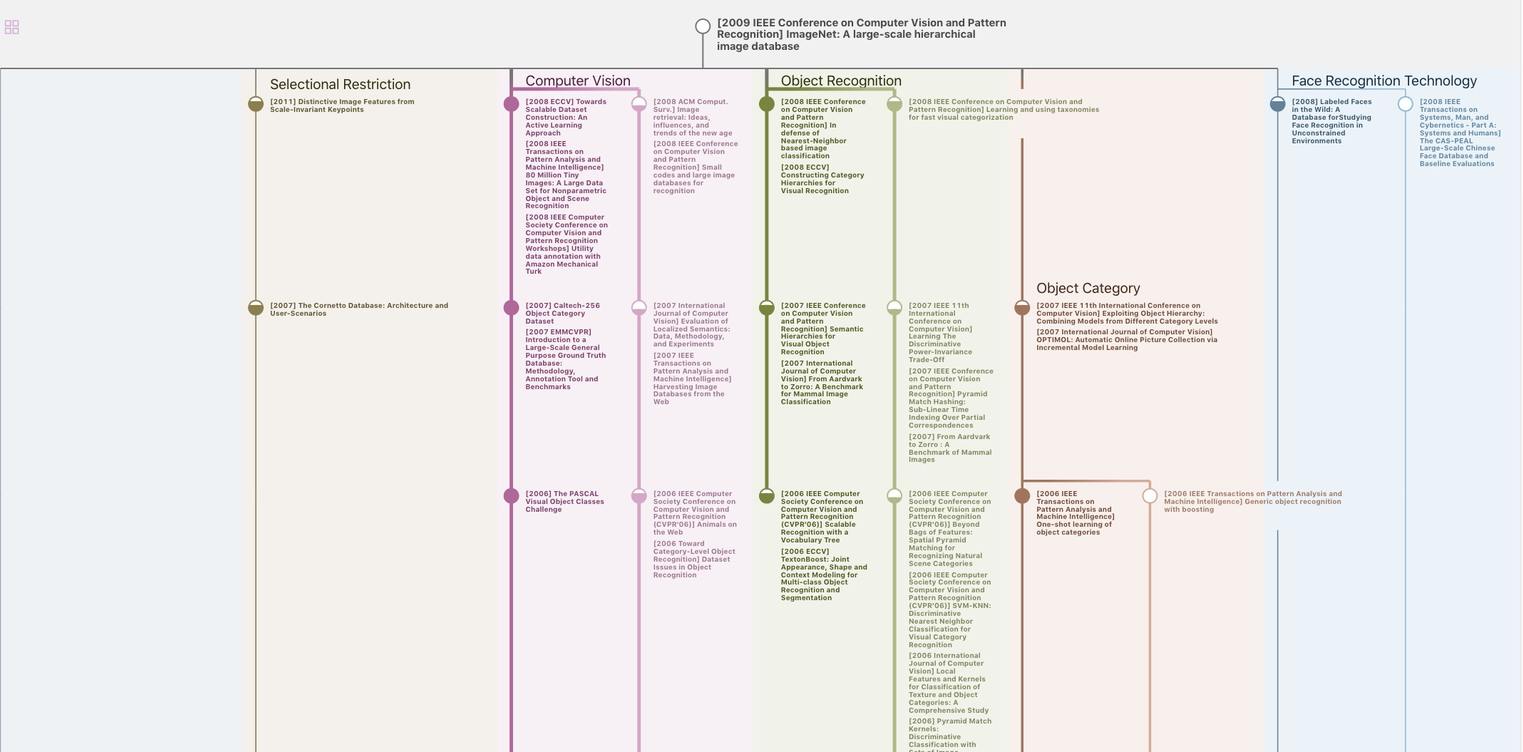
生成溯源树,研究论文发展脉络
Chat Paper
正在生成论文摘要