Exploration of Lightweight Single Image Denoising with Transformers and Truly Fair Training
CoRR(2023)
摘要
As multimedia content often contains noise from intrinsic defects of digital devices, image denoising is an important step for high-level vision recognition tasks. Although several studies have developed the denoising field employing advanced Transformers, these networks are too momory-intensive for real-world applications. Additionally, there is a lack of research on lightweight denosing (LWDN) with Transformers. To handle this, this work provides seven comparative baseline Transformers for LWDN, serving as a foundation for future research. We also demonstrate the parts of randomly cropped patches significantly affect the denoising performances during training. While previous studies have overlooked this aspect, we aim to train our baseline Transformers in a truly fair manner. Furthermore, we conduct empirical analyses of various components to determine the key considerations for constructing LWDN Transformers. Codes are available at https://github.com/rami0205/LWDN.
更多查看译文
关键词
lightweight image denosing baselines, Transformers, fair training, hierarchical network, channel self-attention, spatial self-attention
AI 理解论文
溯源树
样例
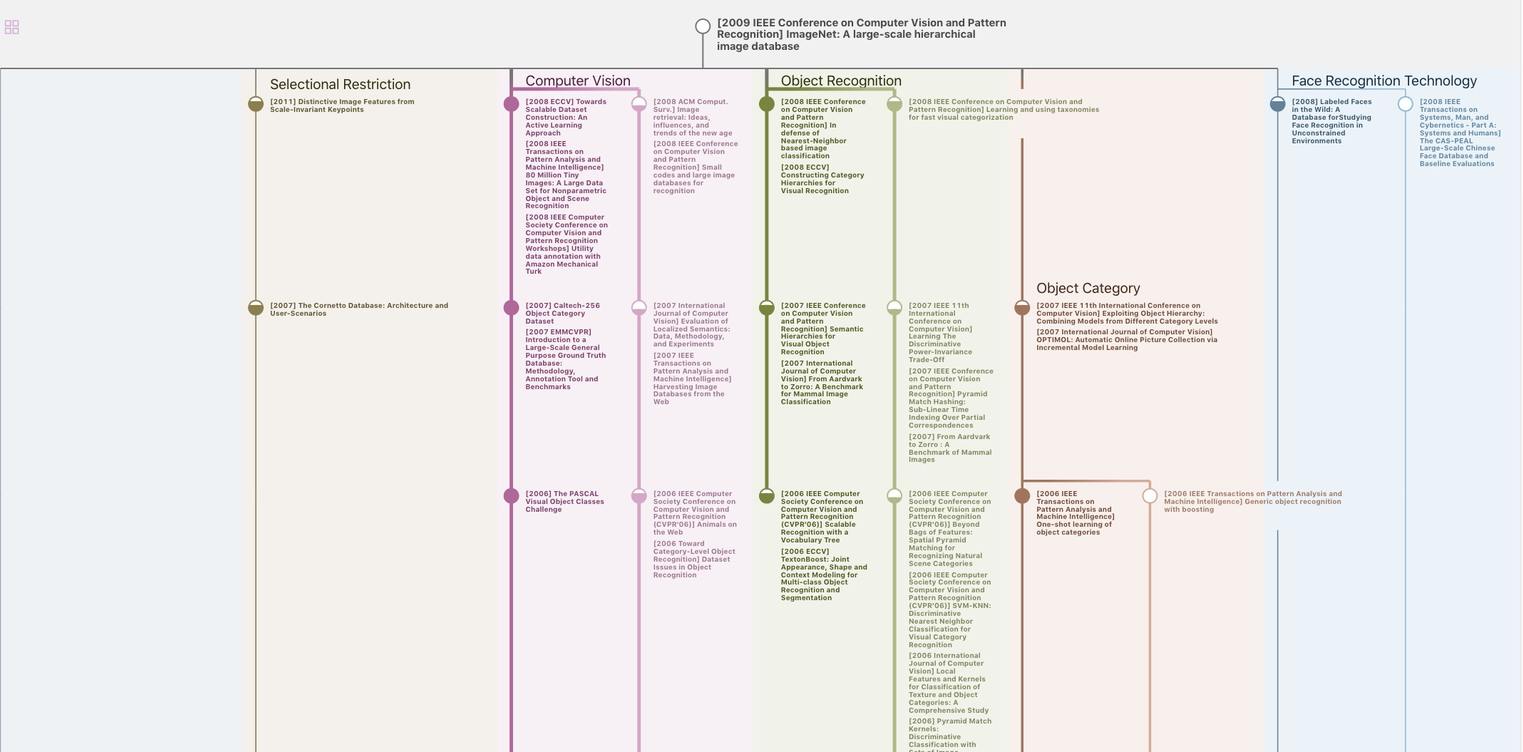
生成溯源树,研究论文发展脉络
Chat Paper
正在生成论文摘要