Mixing predictions for online metric algorithms
CoRR(2023)
摘要
A major technique in learning-augmented online algorithms is combining multiple algorithms or predictors. Since the performance of each predictor may vary over time, it is desirable to use not the single best predictor as a benchmark, but rather a dynamic combination which follows different predictors at different times. We design algorithms that combine predictions and are competitive against such dynamic combinations for a wide class of online problems, namely, metrical task systems. Against the best (in hindsight) unconstrained combination of $\ell$ predictors, we obtain a competitive ratio of $O(\ell^2)$, and show that this is best possible. However, for a benchmark with slightly constrained number of switches between different predictors, we can get a $(1+\epsilon)$-competitive algorithm. Moreover, our algorithms can be adapted to access predictors in a bandit-like fashion, querying only one predictor at a time. An unexpected implication of one of our lower bounds is a new structural insight about covering formulations for the $k$-server problem.
更多查看译文
关键词
online metric algorithms,predictions
AI 理解论文
溯源树
样例
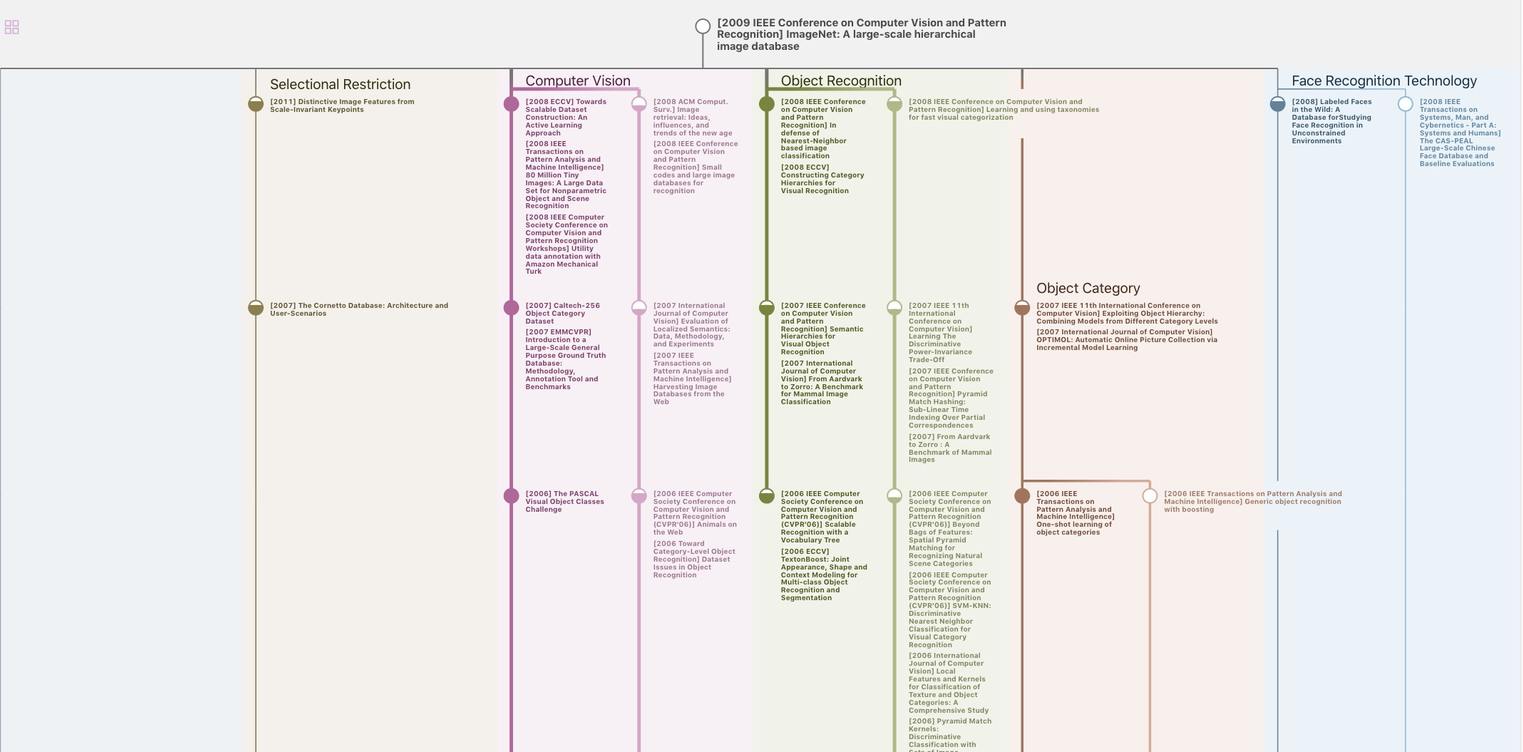
生成溯源树,研究论文发展脉络
Chat Paper
正在生成论文摘要