Multidimensional Perceptron for Efficient and Explainable Long Text Classification
CoRR(2023)
摘要
Because of the inevitable cost and complexity of transformer and pre-trained models, efficiency concerns are raised for long text classification. Meanwhile, in the highly sensitive domains, e.g., healthcare and legal long-text mining, potential model distrust, yet underrated and underexplored, may hatch vital apprehension. Existing methods generally segment the long text, encode each piece with the pre-trained model, and use attention or RNNs to obtain long text representation for classification. In this work, we propose a simple but effective model, Segment-aWare multIdimensional PErceptron (SWIPE), to replace attention/RNNs in the above framework. Unlike prior efforts, SWIPE can effectively learn the label of the entire text with supervised training, while perceive the labels of the segments and estimate their contributions to the long-text labeling in an unsupervised manner. As a general classifier, SWIPE can endorse different encoders, and it outperforms SOTA models in terms of classification accuracy and model efficiency. It is noteworthy that SWIPE achieves superior interpretability to transparentize long text classification results.
更多查看译文
关键词
explainable long text,text
classification,perceptron
AI 理解论文
溯源树
样例
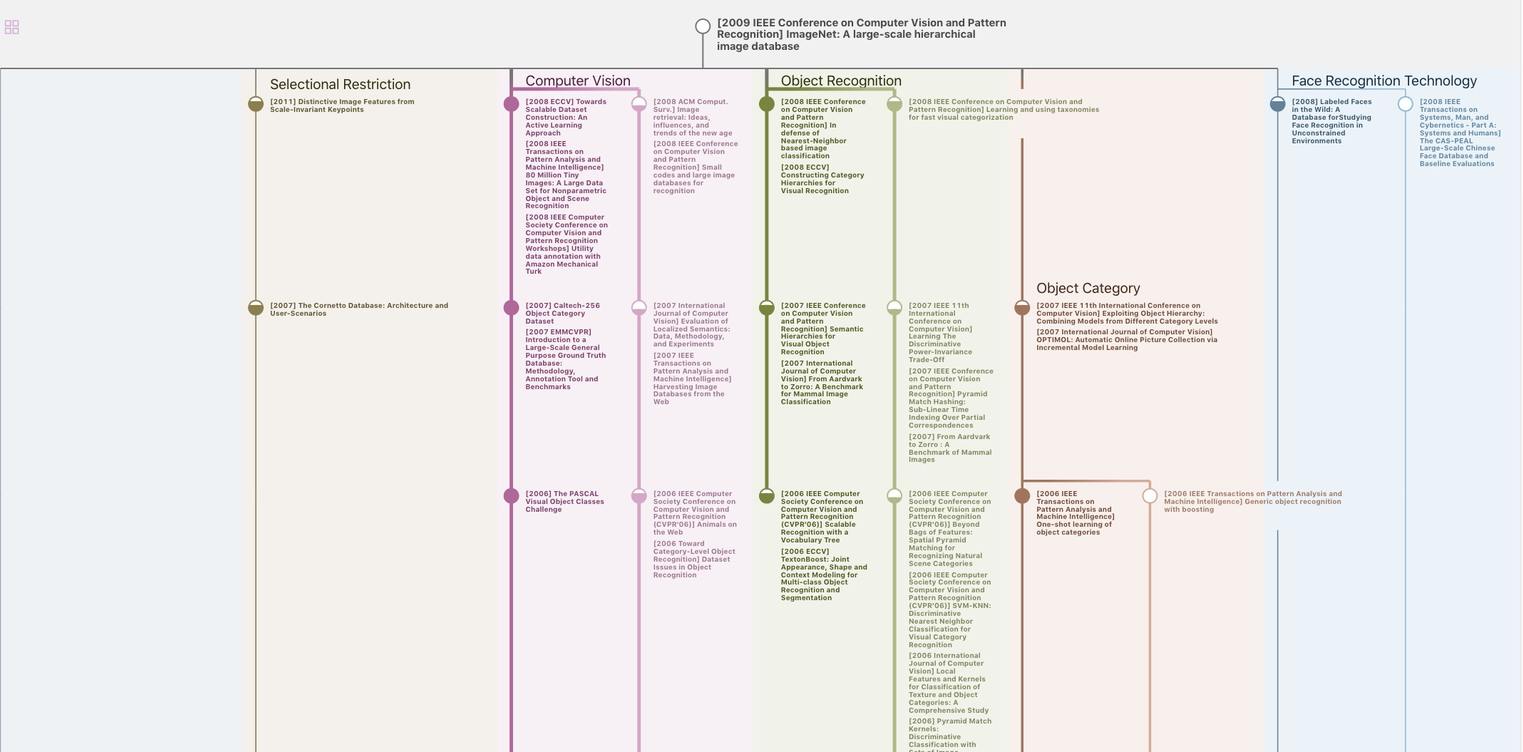
生成溯源树,研究论文发展脉络
Chat Paper
正在生成论文摘要