Local Differential Privacy in Federated Optimization
CoRR(2023)
摘要
Federated optimization, wherein several agents in a network collaborate with a central server to achieve optimal social cost over the network with no requirement for exchanging information among agents, has attracted significant interest from the research community. In this context, agents demand resources based on their local computation. Due to the exchange of optimization parameters such as states, constraints, or objective functions with a central server, an adversary may infer sensitive information of agents. We develop LDP-AIMD, a local differentially-private additive-increase and multiplicative-decrease (AIMD) algorithm, to allocate multiple divisible shared resources to agents in a network. The LDP-AIMD algorithm provides a differential privacy guarantee to agents in the network. No inter-agent communication is required; however, the central server keeps track of the aggregate consumption of resources. We present experimental results to check the efficacy of the algorithm. Moreover, we present empirical analyses for the trade-off between privacy and the efficiency of the algorithm.
更多查看译文
关键词
federated optimization,privacy
AI 理解论文
溯源树
样例
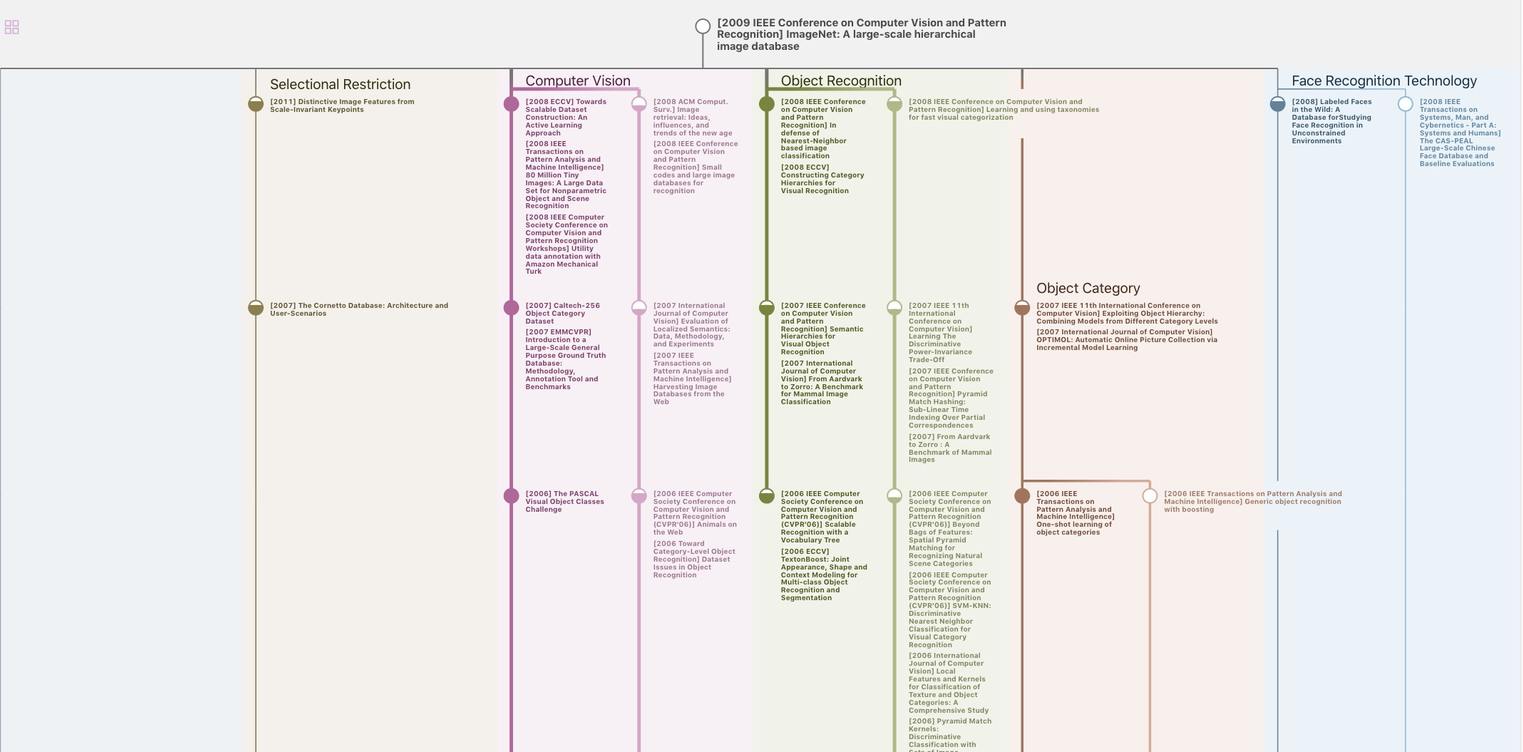
生成溯源树,研究论文发展脉络
Chat Paper
正在生成论文摘要