Can listening to more neighbours help CAVs be faster and safer?
CoRR(2023)
摘要
Connected Autonomous Vehicles (CAVs) are widely expected to improve traffic safety and efficiency by exploiting information from surrounding vehicles via V2V communication. A CAV typically adapts its speed based on information from the vehicle it follows. CAVs can also use information from vehicles further ahead within their communication range, and this results in improved traffic safety and efficiency. In mixed traffic scenarios, however, this may not always be possible due to the presence of human-driven vehicles that do not have communication capabilities. Furthermore, as wireless vehicular networks are unreliable, information from other vehicles can be delayed or lost, which brings more challenges for CAVs in utilizing information from multiple leading vehicles. A few studies have investigated the impact of CAVs where they use information from multiple leading vehicles on traffic safety and efficiency, but only in very limited scenarios (i.e., with a very small number of vehicles). In contrast, this paper investigates the impact of CAV car-following control based on multiple leading vehicles information on both mixed traffic safety and efficiency in realistic scenarios in terms of imperfect communication, vehicle modelling, and traffic scenario. Results show that exploiting information from multiple, rather than a single, leading vehicles in CAV controller design further improves both traffic safety and efficiency especially at high penetration rates. In addition to proper tuning of CAV controller parameters (control gains and time headways), the scale of the improvement depends on both market penetration rate (MPR) and communication reliability. A packet error rate (PER) of 70% leads to an increase in traffic efficiency by 4.18% (at 40% MPR) and 12.19% (at 70% MPR), compared to the simple single leading vehicle information based controller.
更多查看译文
关键词
Connected autonomous vehicles,car-following control,multiple-predecessor-following information flow topology,mixed traffic,unreliable communication
AI 理解论文
溯源树
样例
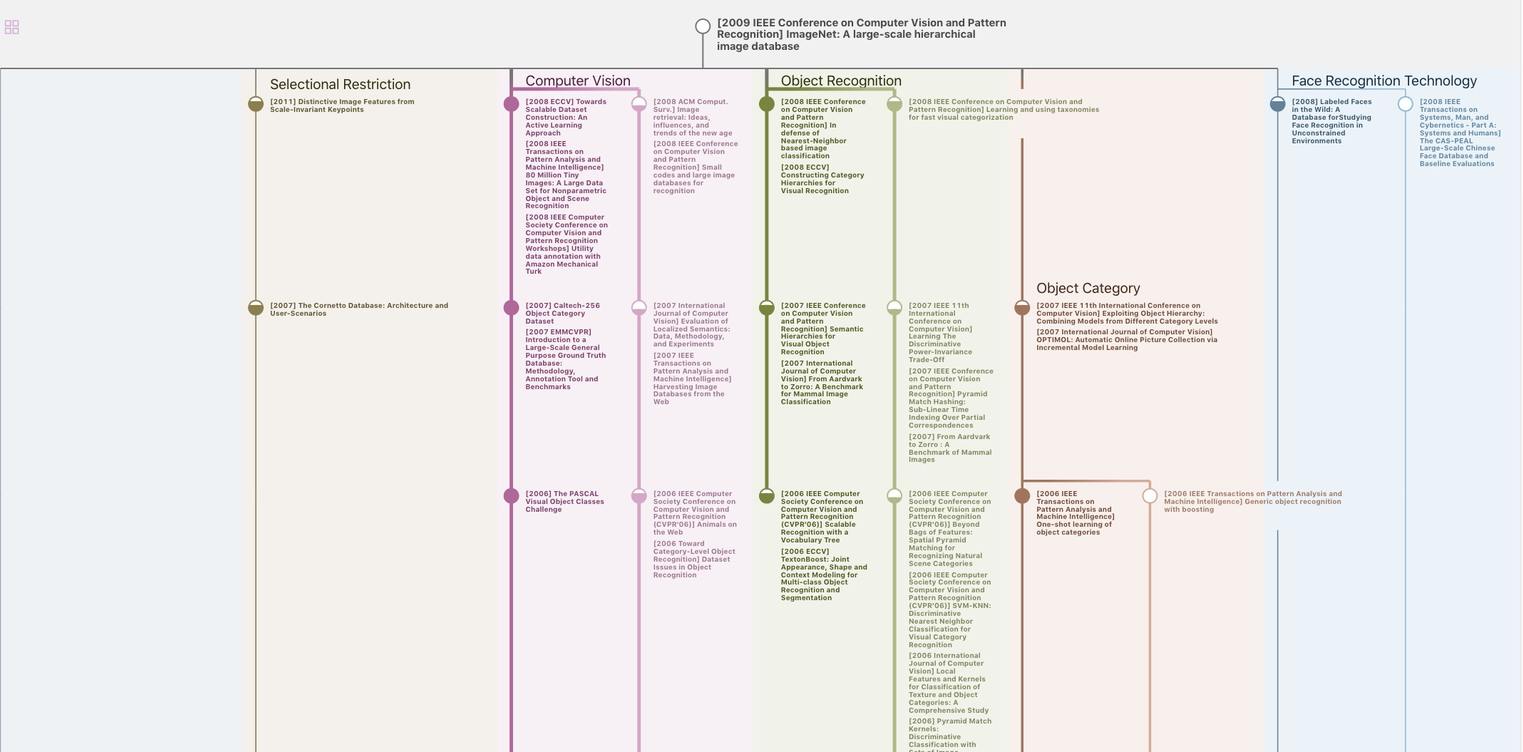
生成溯源树,研究论文发展脉络
Chat Paper
正在生成论文摘要