Accelerating Training of MLIPs Through Small-Cell Training
arXiv (Cornell University)(2023)
Abstract
While machine-learned interatomic potentials have become a mainstay for modeling materials, designing training sets that lead to robust potentials is challenging. Automated methods, such as active learning and on-the-fly learning, allow for the construction of reliable training sets, but these processes can be very resource-intensive when training a potential for use in large-scale simulations. Current training approaches often use density functional theory (DFT) calculations that have the same cell size as the simulations that use the potential. Here, we demonstrate an easy-to-implement small-cell structures training protocol and use it to train a potential for zirconium and hydrides. This training leads to a convex hull in good agreement with DFT when applied to known stable phases. Compared to traditional active learning, small-cell training decreased the training time of a potential able to capture the {\alpha}-\b{eta} zirconium phase transition by approximately 20 times. The potential describes the phase transition with a degree of accuracy similar to that of the large-cell training method.
MoreTranslated text
Key words
mlips,training,small-cell
AI Read Science
Must-Reading Tree
Example
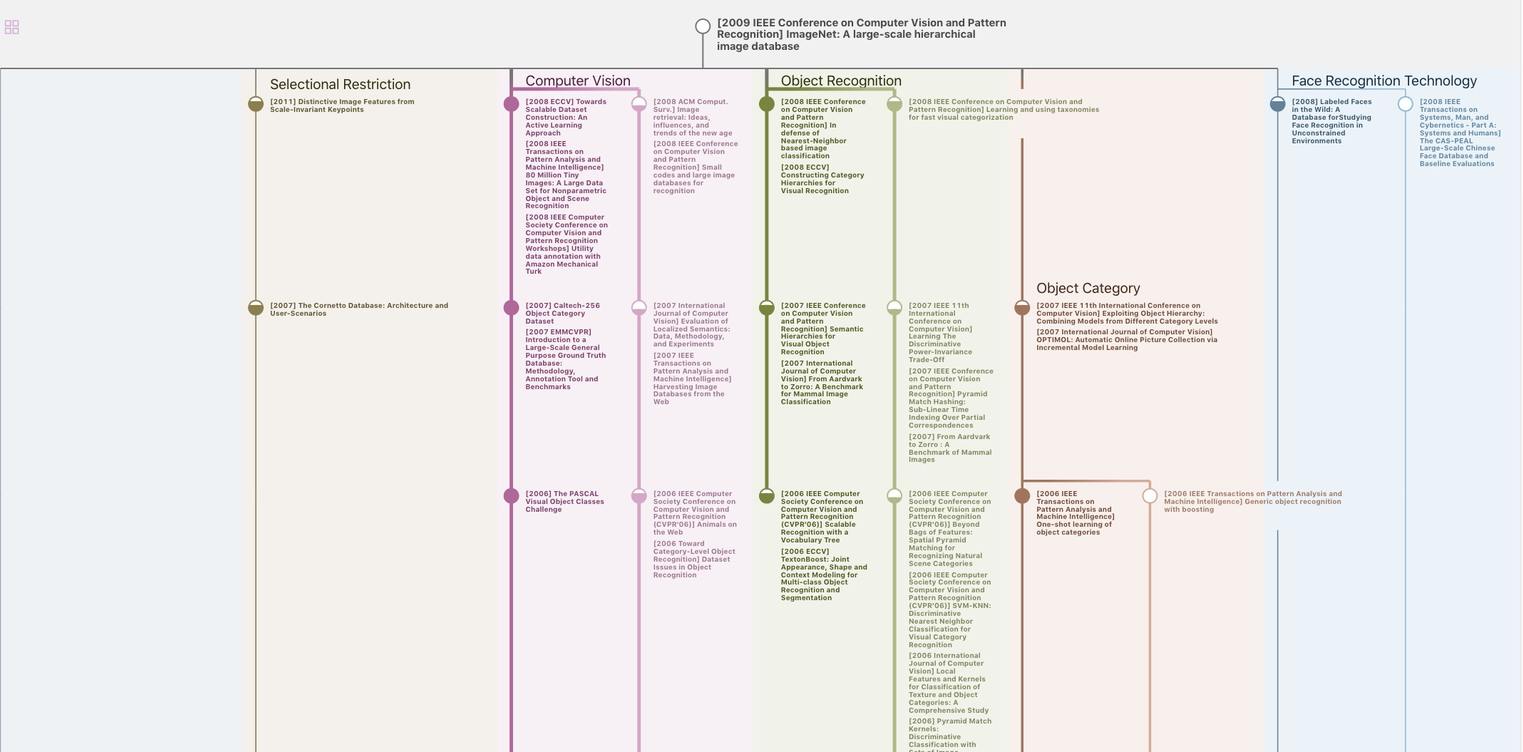
Generate MRT to find the research sequence of this paper
Chat Paper
Summary is being generated by the instructions you defined