Light: joint individual building extraction and height estimation from satellite images through a unified multitask learning network
IGARSS 2023 - 2023 IEEE INTERNATIONAL GEOSCIENCE AND REMOTE SENSING SYMPOSIUM(2023)
摘要
Building extraction and height estimation are two important basic tasks in remote sensing image interpretation, which are widely used in urban planning, real-world 3D construction, and other fields. Most of the existing research regards the two tasks as independent studies. Therefore the height information cannot be fully used to improve the accuracy of building extraction and vice versa. In this work, we combine the individuaL buIlding extraction and heiGHt estimation through a unified multiTask learning network (LIGHT) for the first time, which simultaneously outputs a height map, bounding boxes, and a segmentation mask map of buildings. Specifically, LIGHT consists of an instance segmentation branch and a height estimation branch. In particular, so as to effectively unify multi-scale feature branches and alleviate feature spans between branches, we propose a Gated Cross Task Interaction (GCTI) module that can efficiently perform feature interaction between branches. Experiments on the DFC2023 dataset show that our LIGHT can achieve superior performance, and our GCTI module with ResNet 101 as the backbone can significantly improve the performance of multitask learning by 2.8% AP50 and 6.5% d1, respectively.
更多查看译文
关键词
Building Extraction,Instance Segmentation,Height Estimation,Multitask Learning,Cross Task Interaction
AI 理解论文
溯源树
样例
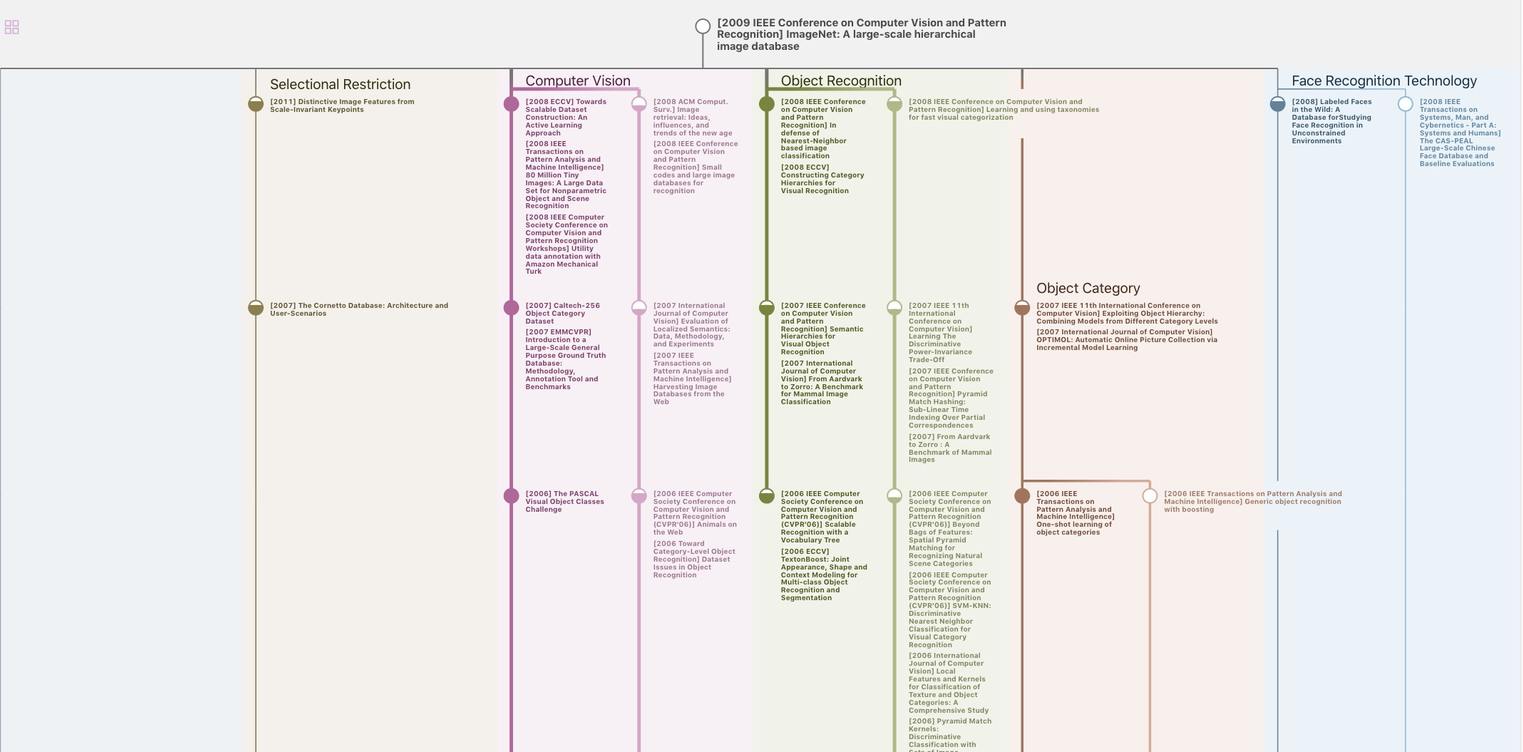
生成溯源树,研究论文发展脉络
Chat Paper
正在生成论文摘要