Safety Under Uncertainty: Tight Bounds with Risk-Aware Control Barrier Functions
CoRR(2023)
摘要
We propose a novel class of risk-aware control barrier functions (RA-CBFs) for the control of stochastic safety-critical systems. Leveraging a result from the stochastic level-crossing literature, we deviate from the martingale theory that is currently used in stochastic CBF techniques and prove that a RA-CBF based control synthesis confers a tighter upper bound on the probability of the system becoming unsafe within a finite time interval than existing approaches. We highlight the advantages of our proposed approach over the state-of-the-art via a comparative study on an mobile-robot example, and further demonstrate its viability on an autonomous vehicle highway merging problem in dense traffic.
更多查看译文
关键词
safety,barrier
AI 理解论文
溯源树
样例
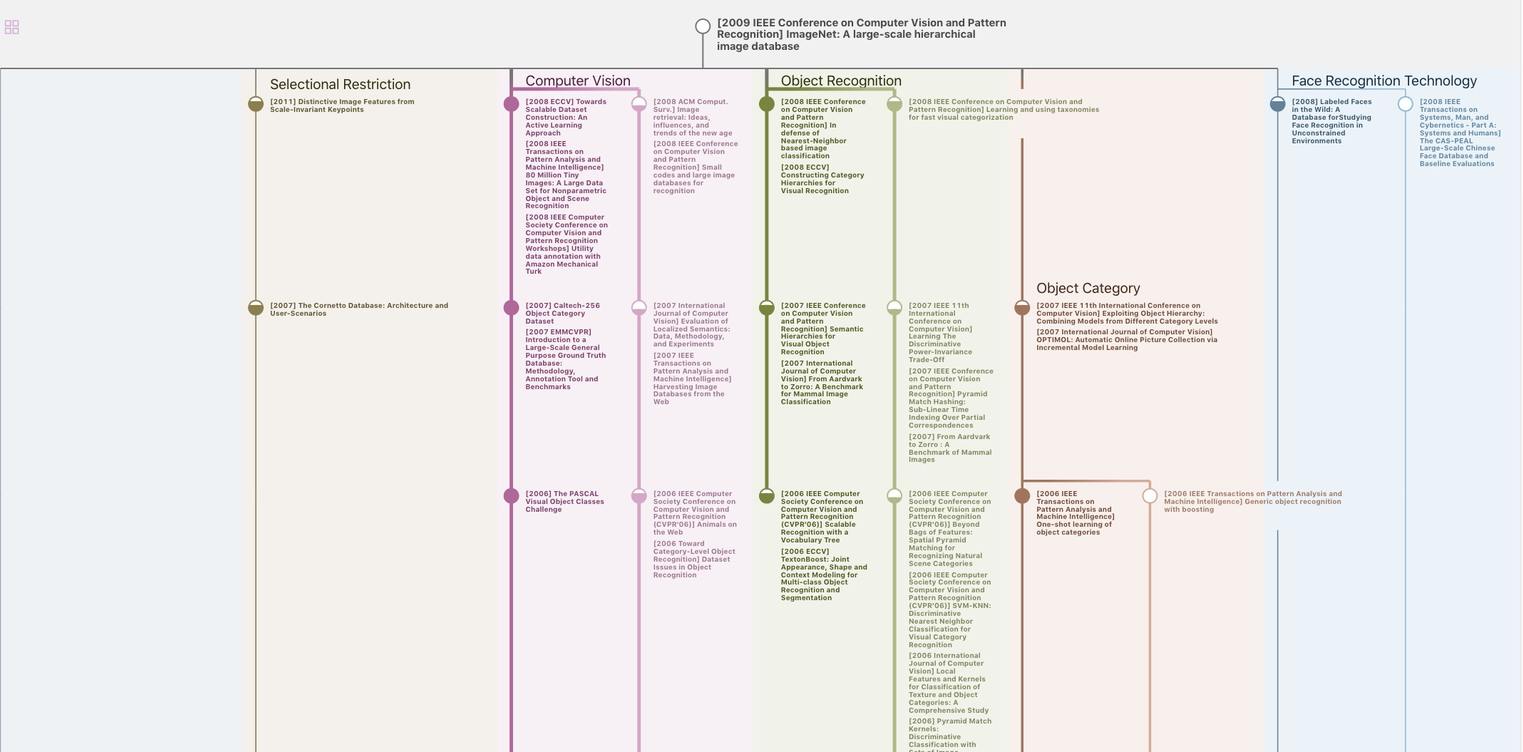
生成溯源树,研究论文发展脉络
Chat Paper
正在生成论文摘要