Self-Supervised Multimodal Learning: A Survey
CoRR(2023)
摘要
Multimodal learning, which aims to understand and analyze information from multiple modalities, has achieved substantial progress in the supervised regime in recent years. However, the heavy dependence on data paired with expensive human annotations impedes scaling up models. Meanwhile, given the availability of large-scale unannotated data in the wild, self-supervised learning has become an attractive strategy to alleviate the annotation bottleneck. Building on these two directions, self-supervised multimodal learning (SSML) provides ways to leverage supervision from raw multimodal data. In this survey, we provide a comprehensive review of the state-of-the-art in SSML, which we categorize along three orthogonal axes: objective functions, data alignment, and model architectures. These axes correspond to the inherent characteristics of self-supervised learning methods and multimodal data. Specifically, we classify training objectives into instance discrimination, clustering, and masked prediction categories. We also discuss multimodal input data pairing and alignment strategies during training. Finally, we review model architectures including the design of encoders, fusion modules, and decoders, which are essential components of SSML methods. We review downstream multimodal application tasks, reporting the concrete performance of the state-of-the-art image-text models and multimodal video models, and also review real-world applications of SSML algorithms in diverse fields such as healthcare, remote sensing, and machine translation. Finally, we discuss challenges and future directions for SSML. A collection of related resources can be found at: https://github.com/ys-zong/awesome-self-supervised-multimodal-learning.
更多查看译文
关键词
multimodal learning,survey,self-supervised
AI 理解论文
溯源树
样例
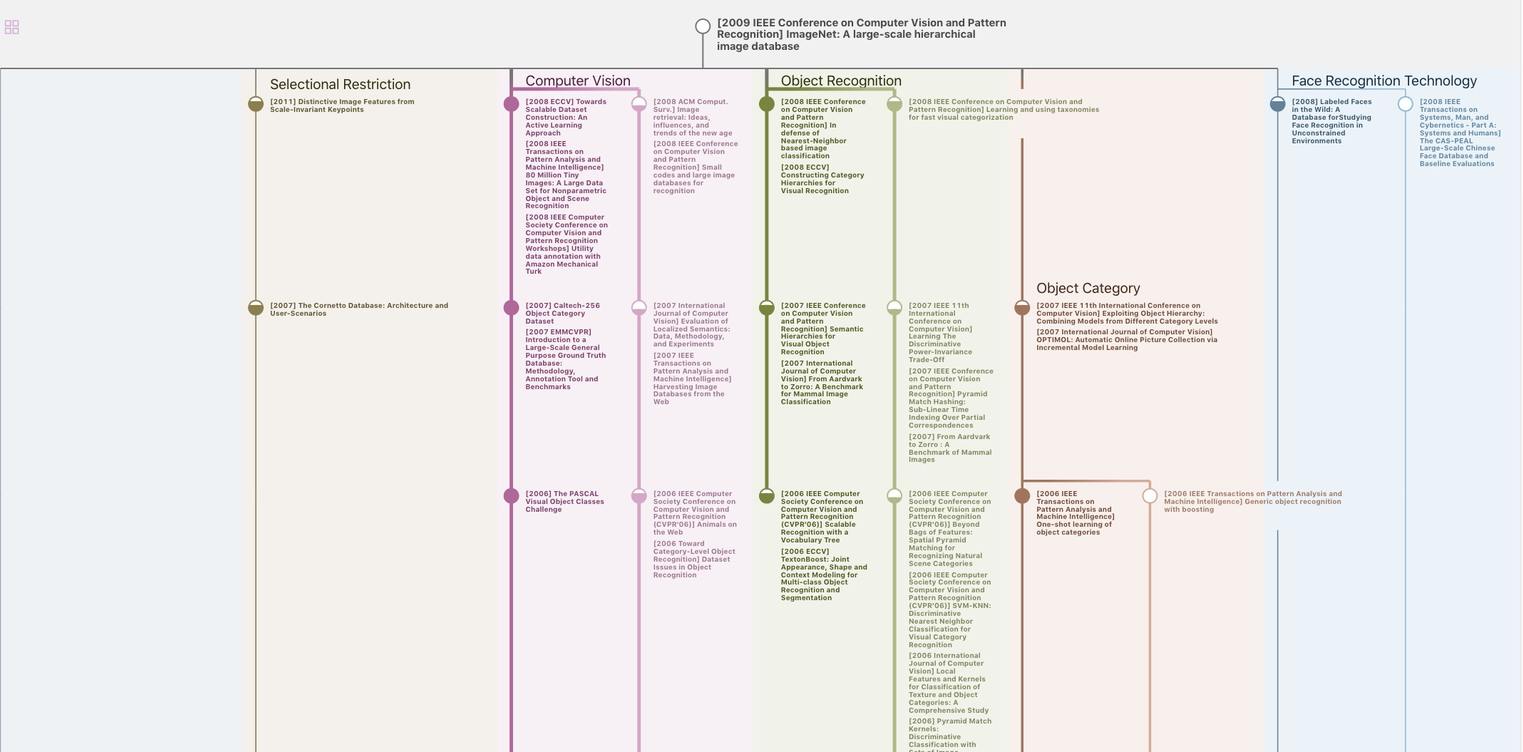
生成溯源树,研究论文发展脉络
Chat Paper
正在生成论文摘要