Online Learning and Control for Data-Augmented Quadrotor Model
CoRR(2023)
摘要
The ability to adapt to changing conditions is a key feature of a successful autonomous system. In this work, we use the Recursive Gaussian Processes (RGP) for identification of the quadrotor air drag model online, without the need of training data. The identified drag model then augments a physics-based model of the quadrotor dynamics, which allows more accurate quadrotor state prediction with increased ability to adapt to changing conditions. This data-augmented physics-based model is utilized for precise quadrotor trajectory tracking using the suitably modified Model Predictive Control (MPC) algorithm. The proposed modelling and control approach is evaluated using the Gazebo simulator and it is shown that the proposed approach tracks a desired trajectory with a higher accuracy compared to the MPC with the non-augmented (purely physics-based) model.
更多查看译文
关键词
online learning,control,data-augmented
AI 理解论文
溯源树
样例
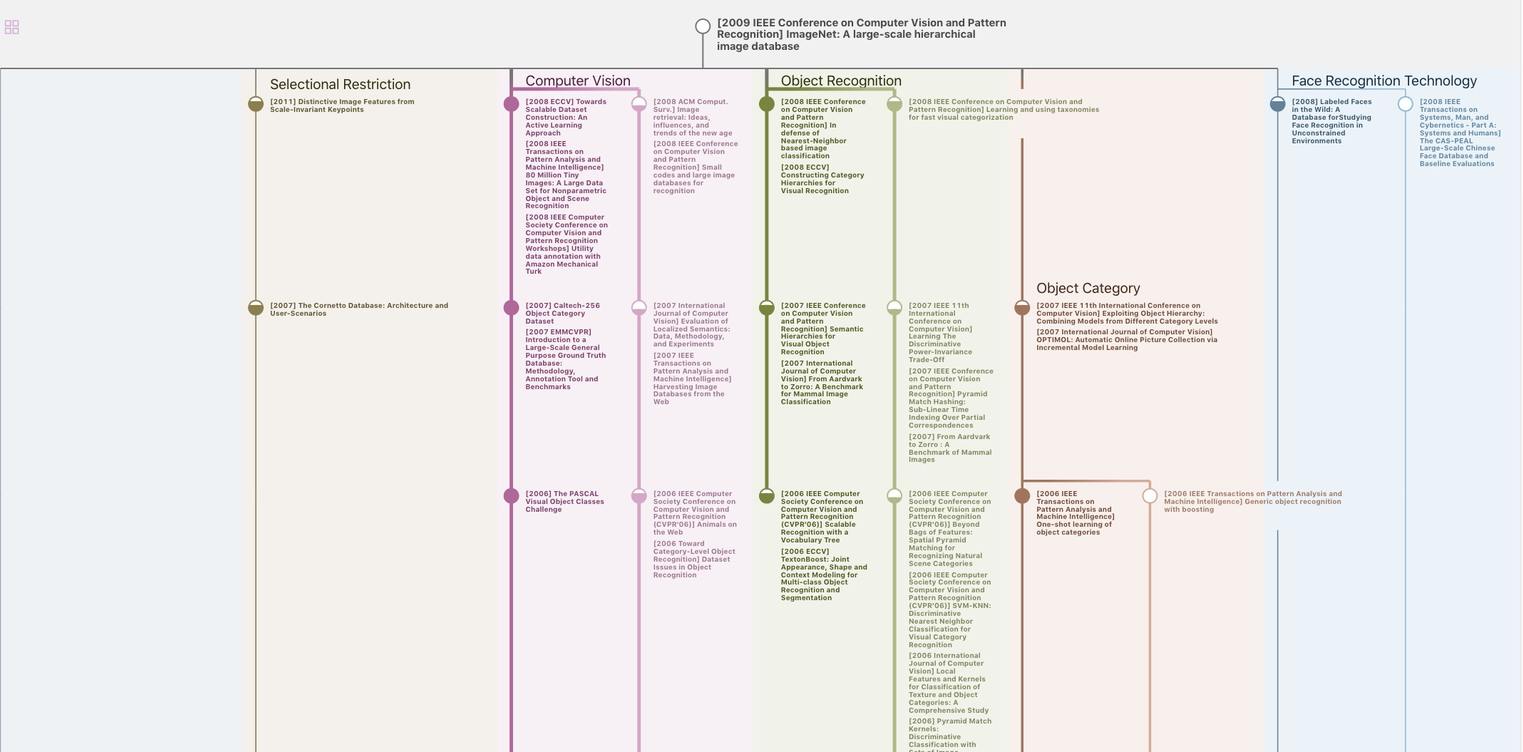
生成溯源树,研究论文发展脉络
Chat Paper
正在生成论文摘要