Infinite-dimensional Reservoir Computing.
Neural Networks(2024)
Abstract
Reservoir computing approximation and generalization bounds are proved for a new concept class of input/output systems that extends the so-called generalized Barron functionals to a dynamic context. This new class is characterized by the readouts with a certain integral representation built on infinite-dimensional state-space systems. It is shown that this class is very rich and possesses useful features and universal approximation properties. The reservoir architectures used for the approximation and estimation of elements in the new class are randomly generated echo state networks with either linear or ReLU activation functions. Their readouts are built using randomly generated neural networks in which only the output layer is trained (extreme learning machines or random feature neural networks). The results in the paper yield a fully implementable recurrent neural network-based learning algorithm with provable convergence guarantees that do not suffer from the curse of dimensionality.
MoreTranslated text
Key words
Recurrent neural network,Reservoir computing,Echo state network,ESN,Extreme learning machine,ELM,Recurrent linear network,Machine learning,Barron functional,Recurrent barron functional,Universality,Finite memory functional,Approximation bound,Convolutional filter
AI Read Science
Must-Reading Tree
Example
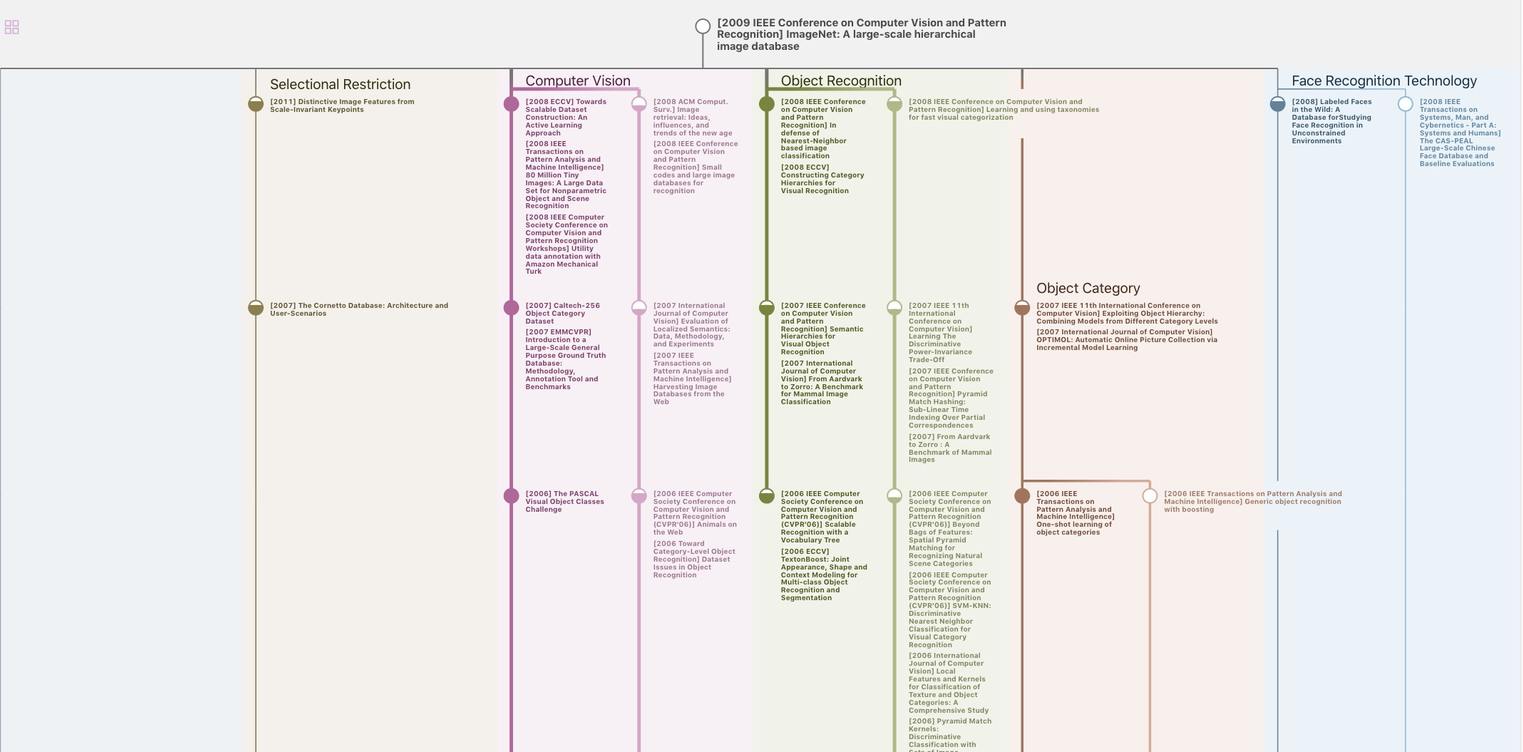
Generate MRT to find the research sequence of this paper
Chat Paper
Summary is being generated by the instructions you defined