Fair-CDA: Continuous and Directional Augmentation for Group Fairness
CoRR(2023)
摘要
In this work, we propose {\it Fair-CDA}, a fine-grained data augmentation strategy for imposing fairness constraints. We use a feature disentanglement method to extract the features highly related to the sensitive attributes. Then we show that group fairness can be achieved by regularizing the models on transition paths of sensitive features between groups. By adjusting the perturbation strength in the direction of the paths, our proposed augmentation is controllable and auditable. To alleviate the accuracy degradation caused by fairness constraints, we further introduce a calibrated model to impute labels for the augmented data. Our proposed method does not assume any data generative model and ensures good generalization for both accuracy and fairness. Experimental results show that Fair-CDA consistently outperforms state-of-the-art methods on widely-used benchmarks, e.g., Adult, CelebA and MovieLens. Especially, Fair-CDA obtains an 86.3\% relative improvement for fairness while maintaining the accuracy on the Adult dataset. Moreover, we evaluate Fair-CDA in an online recommendation system to demonstrate the effectiveness of our method in terms of accuracy and fairness.
更多查看译文
关键词
group fairness,directional augmentation,fair-cda
AI 理解论文
溯源树
样例
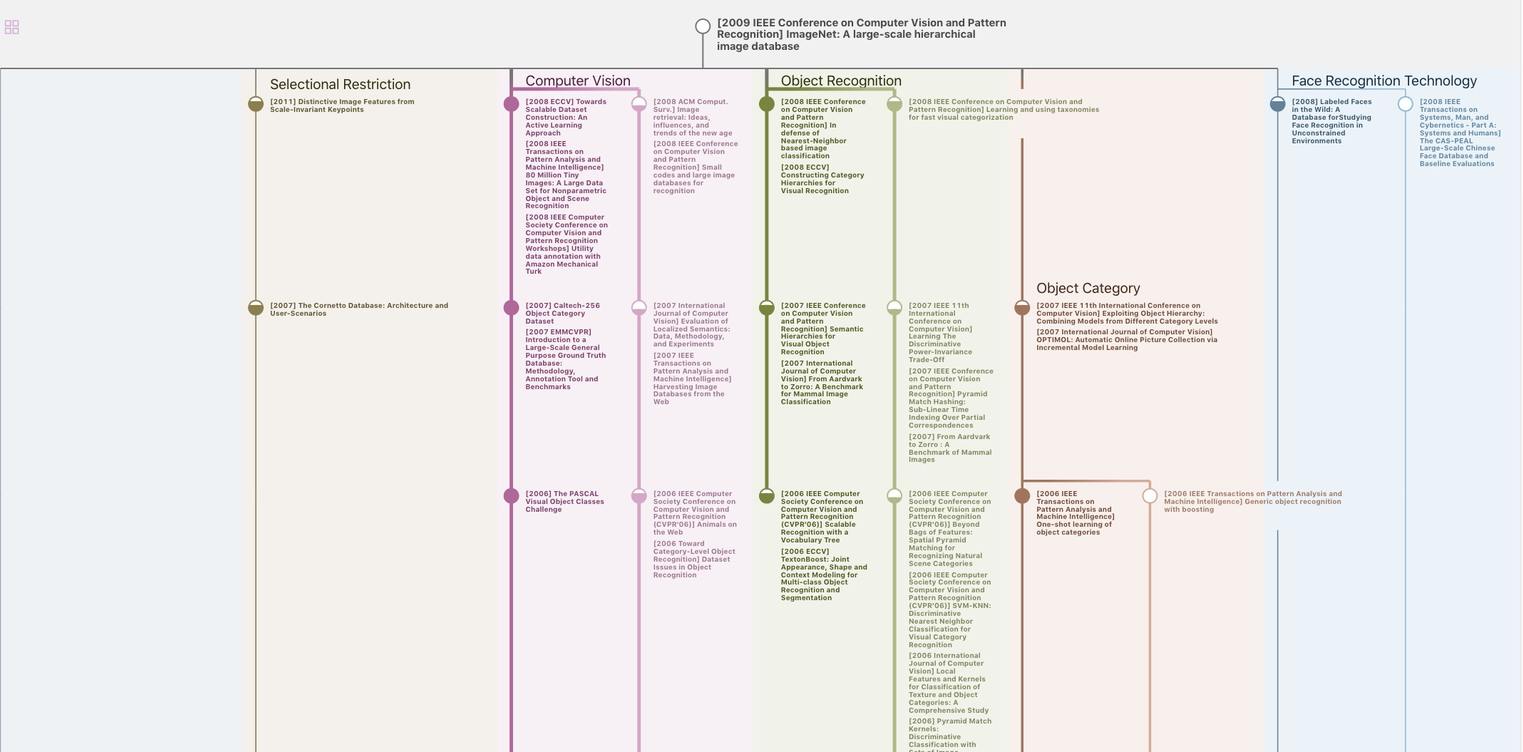
生成溯源树,研究论文发展脉络
Chat Paper
正在生成论文摘要