A Robust Deep Learning-Based Damage Identification Approach for SHM Considering Missing Data
CoRR(2023)
摘要
Data-driven methods have shown promising results in structural health monitoring (SHM) applications. However, most of these approaches rely on the ideal dataset assumption and do not account for missing data, which can significantly impact their real-world performance. Missing data is a frequently encountered issue in time series data, which hinders standardized data mining and downstream tasks such as damage identification and condition assessment. While imputation approaches based on spatiotemporal relations among monitoring data have been proposed to handle this issue, they do not provide additional helpful information for downstream tasks. This paper proposes a robust deep learning-based method that unifies missing data imputation and damage identification tasks into a single framework. The proposed approach is based on a long short-term memory (LSTM) structured autoencoder (AE) framework, and missing data is simulated using the dropout mechanism by randomly dropping the input channels. Reconstruction errors serve as the loss function and damage indicator. The proposed method is validated using the quasi-static response (cable tension) of a cable-stayed bridge released in the 1st IPC-SHM, and results show that missing data imputation and damage identification can be effectively integrated into the proposed unified framework.
更多查看译文
关键词
structural health monitoring,missing data,damage identification,deep learning
AI 理解论文
溯源树
样例
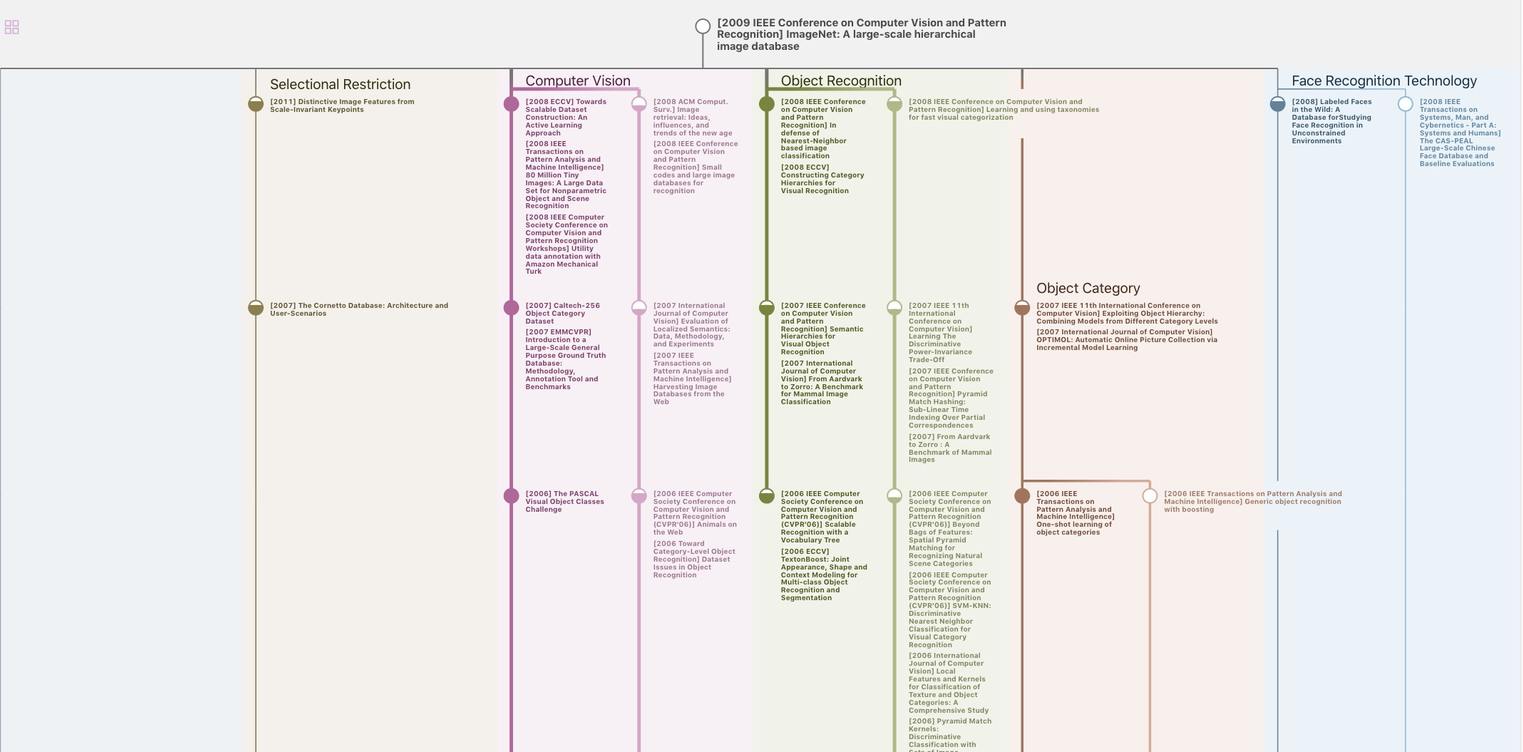
生成溯源树,研究论文发展脉络
Chat Paper
正在生成论文摘要