Boolean network sketches: a unifying framework for logical model inference.
Bioinform.(2023)
摘要
Motivation The problem of model inference is of fundamental importance to systems biology. Logical models (e.g. Boolean networks; BNs) represent a computationally attractive approach capable of handling large biological networks. The models are typically inferred from experimental data. However, even with a substantial amount of experimental data supported by some prior knowledge, existing inference methods often focus on a small sample of admissible candidate models only. Results We propose Boolean network sketches as a new formal instrument for the inference of Boolean networks. A sketch integrates (typically partial) knowledge about the network ' s topology and the update logic (obtained through, e.g. a biological knowledge base or a literature search), as well as further assumptions about the properties of the network ' s transitions (e.g. the form of its attractor landscape), and additional restrictions on the model dynamics given by the measured experimental data. Our new BNs inference algorithm starts with an ' initial ' sketch, which is extended by adding restrictions representing experimental data to a ' data-informed ' sketch and subsequently computes all BNs consistent with the data-informed sketch. Our algorithm is based on a symbolic representation and coloured model-checking. Our approach is unique in its ability to cover a broad spectrum of knowledge and efficiently produce a compact representation of all inferred BNs. We evaluate the method on a non-trivial collection of real-world and simulated data. Availability and implementation: All software and data are freely available as a reproducible artefact at https://doi. org/10.5281/zenodo.7688740.
更多查看译文
关键词
boolean network,inference,model
AI 理解论文
溯源树
样例
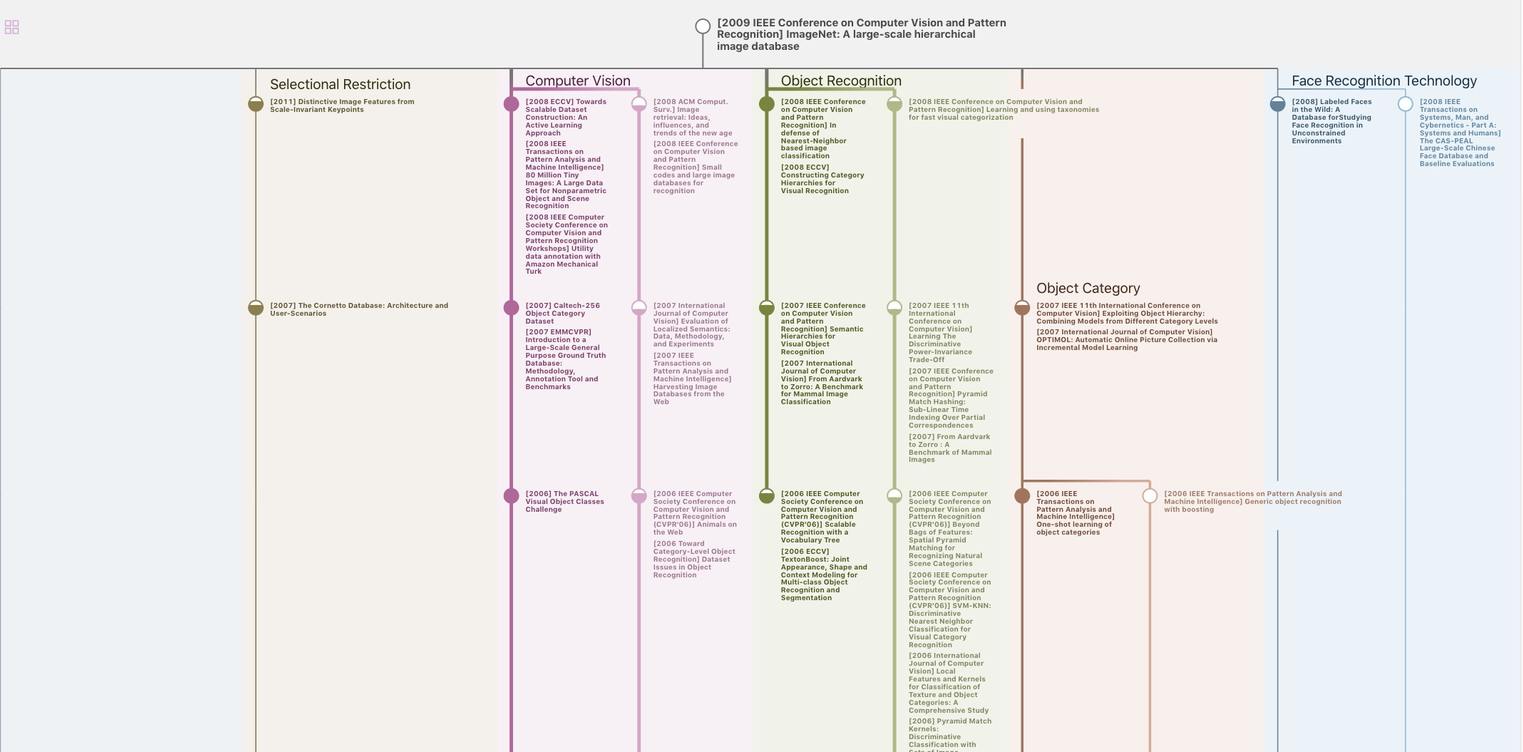
生成溯源树,研究论文发展脉络
Chat Paper
正在生成论文摘要