Brain-machine interface based on transfer-learning for detecting the appearance of obstacles during exoskeleton-assisted walking.
Frontiers in neuroscience(2023)
摘要
The results were superior when using the methodology of the two consecutive networks vs. only the first one in a cross-validation pseudo-online analysis. The false positives per min (FP/min) decreased from 31.8 to 3.9 FP/min and the number of repetitions in which there were no false positives and true positives (TP) improved from 34.9% to 60.3% NOFP/TP. This methodology was tested in a closed-loop experiment with an exoskeleton, in which the brain-machine interface (BMI) detected an obstacle and sent the command to the exoskeleton to stop. This methodology was tested with three healthy subjects, and the online results were 3.8 FP/min and 49.3% NOFP/TP. To make this model feasible for non-able bodied patients with a reduced and manageable time frame, transfer-learning techniques were applied and validated in the previous tests, and were then applied to patients. The results for two incomplete Spinal Cord Injury (iSCI) patients were 37.9% NOFP/TP and 7.7 FP/min.
更多查看译文
关键词
BMI,EEG,closed-loop,exoskeleton,stopping intention,transfer-learning
AI 理解论文
溯源树
样例
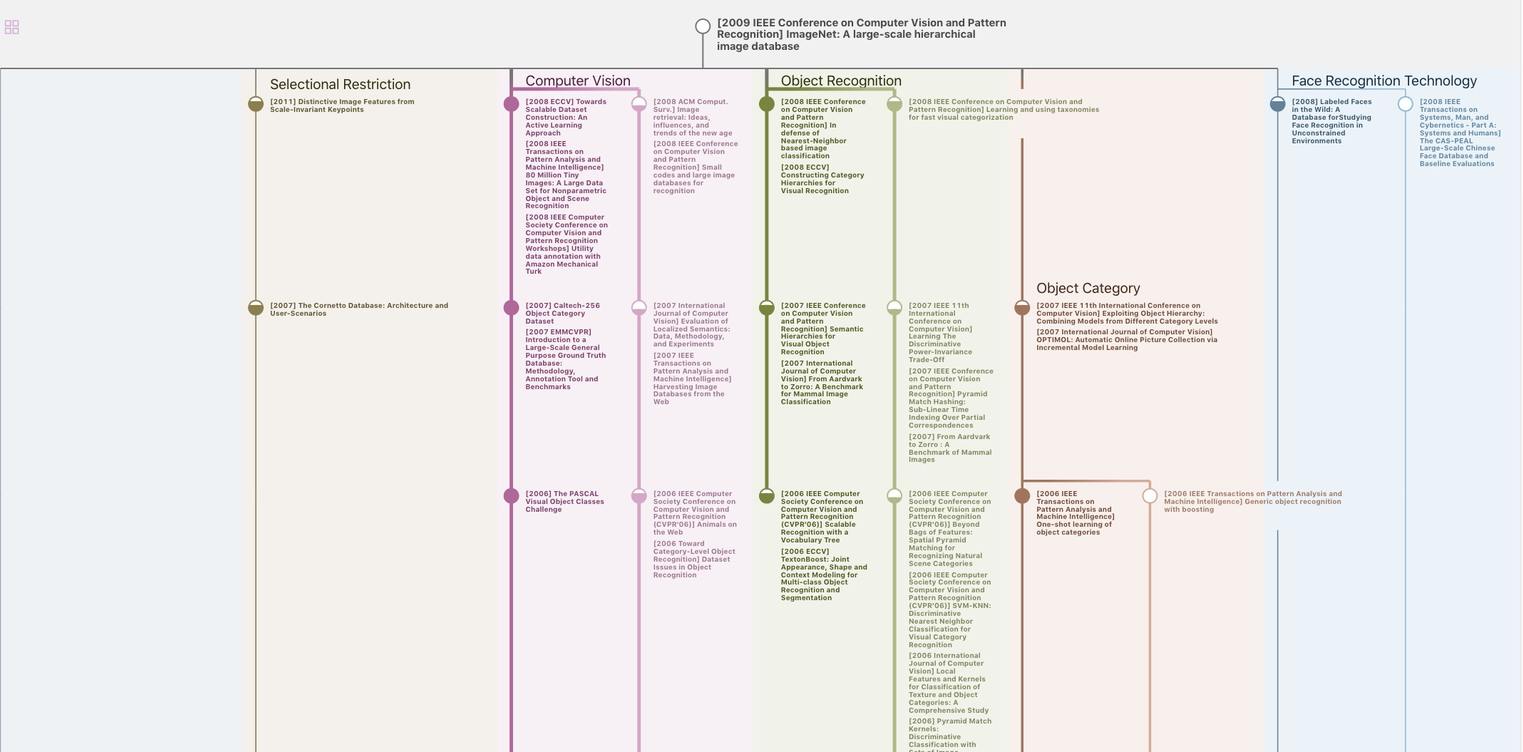
生成溯源树,研究论文发展脉络
Chat Paper
正在生成论文摘要