Radiomic tumor phenotypes augment molecular profiling in predicting recurrence free survival after breast neoadjuvant chemotherapy
COMMUNICATIONS MEDICINE(2023)
摘要
BackgroundEarly changes in breast intratumor heterogeneity during neoadjuvant chemotherapy may reflect the tumor's ability to adapt and evade treatment. We investigated the combination of precision medicine predictors of genomic and MRI data towards improved prediction of recurrence free survival (RFS).MethodsA total of 100 women from the ACRIN 6657/I-SPY 1 trial were retrospectively analyzed. We estimated MammaPrint, PAM50 ROR-S, and p53 mutation scores from publicly available gene expression data and generated four, voxel-wise 3-D radiomic kinetic maps from DCE-MR images at both pre- and early-treatment time points. Within the primary lesion from each kinetic map, features of change in radiomic heterogeneity were summarized into 6 principal components.ResultsWe identify two imaging phenotypes of change in intratumor heterogeneity (p < 0.01) demonstrating significant Kaplan-Meier curve separation (p < 0.001). Adding phenotypes to established prognostic factors, functional tumor volume (FTV), MammaPrint, PAM50, and p53 scores in a Cox regression model improves the concordance statistic for predicting RFS from 0.73 to 0.79 (p = 0.002).ConclusionsThese results demonstrate an important step in combining personalized molecular signatures and longitudinal imaging data towards improved prognosis. Chitalia, Miliotis et al. evaluate radiomic predictors of outcome in patients with breast cancer treated with neoadjuvant chemotherapy. The authors identify radiomic phenotypes related to changes in tumor heterogeneity that improve progression-free survival prediction when added to clinicopathological and molecular factors. Plain language summaryEarly changes in tumor properties during treatment may tell us whether or not a patient's tumor is responding to treatment. Such changes may be seen on imaging. Here, changes in breast cancer properties are identified on imaging and are used in combination with gene markers to investigate whether response to treatment can be predicted using mathematical models. We demonstrate that tumor properties seen on imaging early on in treatment can help to predict patient outcomes. Our approach may allow clinicians to better inform patients about their prognosis and choose appropriate and effective therapies.
更多查看译文
关键词
Predictive markers,Tumour biomarkers,Medicine/Public Health,general
AI 理解论文
溯源树
样例
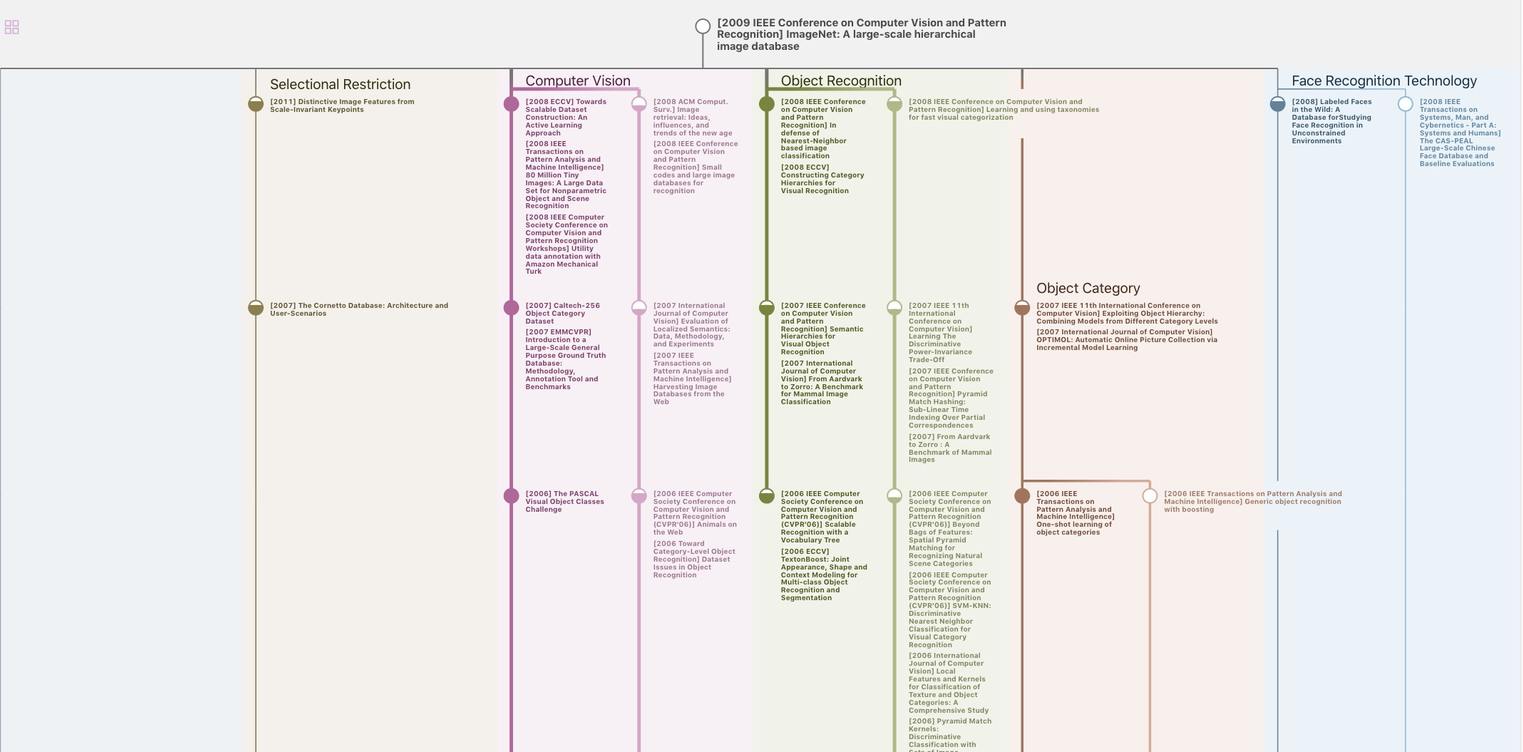
生成溯源树,研究论文发展脉络
Chat Paper
正在生成论文摘要