Adversarial composite prediction of normal video dynamics for anomaly detection
Computer Vision and Image Understanding(2023)
摘要
Dynamic modeling of normal videos is the key problem for unsupervised video anomaly detection. In this paper, we propose a novel adversarial composite prediction framework for unsupervised video anomaly detection, namely ACP-VAD. It consists of an adversarial composite prediction (ACP) module and a foreground motion metric learning (FMML) module. Given a video sequence, ACP module aims to learn reasonable normal video dynamics by using a generator for the sequential and composite predicting of video frames, and two discriminators for the frame-level and sequence-level adversarial learning. Moreover, to learn a more compact representation of normal video dynamics and improve the detection sensibility of motion anomalies, FMML module utilizes the sophisticated metric learning scheme over the latent space distributions of large foreground motion amplitude sequence, real normal sequence as well as real normal sequence containing previously sequential or composite prediction frames. Through these two modules, our framework can learn reasonable and compact spatial-temporal dynamics for normal videos, and the final anomaly detection task can be solved by computing the error between the predicted frame and its corresponding real frame. Comprehensive experimental results on UCSD Ped2, Avenue, and ShanghaiTech datasets demonstrate the effectiveness of both ACP module and FMML module, and our final ACP-VAD framework achieves impressive performance, surpassing most of the state-of-the-art methods.
更多查看译文
关键词
adversarial composite prediction,normal video dynamics,anomaly,detection
AI 理解论文
溯源树
样例
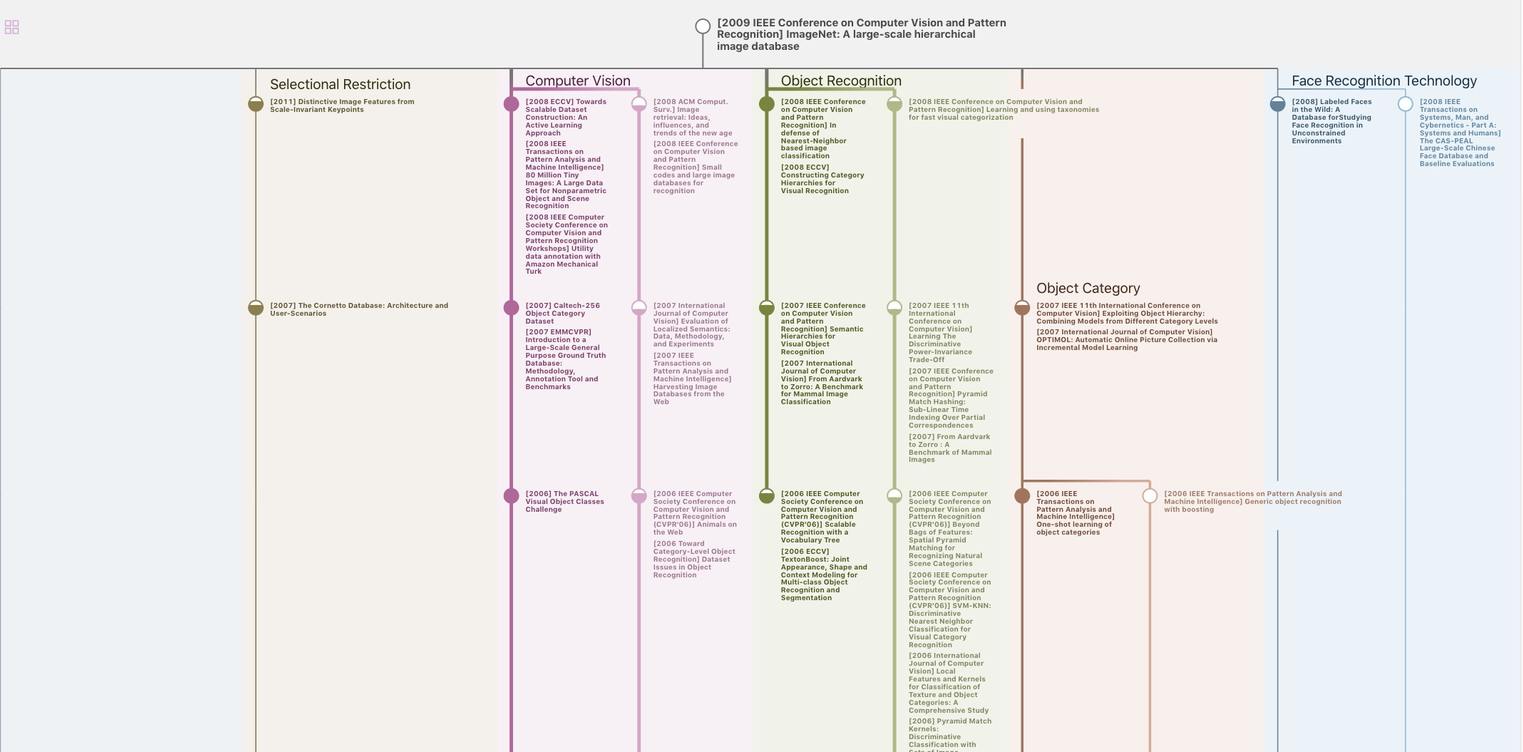
生成溯源树,研究论文发展脉络
Chat Paper
正在生成论文摘要