Deep representation learning of scientific paper reveals its potential scholarly impact.
J. Informetrics(2023)
摘要
Citation and citation-based metrics are traditionally used to quantify the scholarly impact of scientific papers. However, for documents without citation data, i.e., newly published papers, the citation-based metrics are not available. By leveraging deep representation techniques, we propose a text-content based approach that may reveal the scholarly impact of papers without human domain-specific knowledge. Specifically, a large-scale Pre-Trained Model (PTM) with 110 million parameters is utilized to automatically encode the paper into the vector representation. Two indicators, tau (Topicality) and sigma (Originality), are then proposed based on the learned representations. These two indicators leverage the spatial relations of paper representations in the semantic space to capture the impact-related characteristics of a scientific paper. Extensive experiments have been conducted on a COVID-19 open research dataset with 1,056,660 papers. The experimental results demonstrate that the deep representation learning method can better capture the scientific content in the published literature; and the proposed indicators are positively and significantly associated with a paper's potential scholarly impact. In the multivariate regression analysis for the potential impact of a paper, the coefficients of sigma and tau are 5.4915 (P < 0.001) and 6.6879 (P < 0.001) for next 6 months prediction, 12.9964 (P < 0.001) and 13.8678 (P < 0.001) for next 12 months prediction. The proposed framework may facilitate the study of how scholarly impact is generated, from a textual representation perspective.
更多查看译文
关键词
Scholarly impact,Deep representation learning,Topicality,Originality
AI 理解论文
溯源树
样例
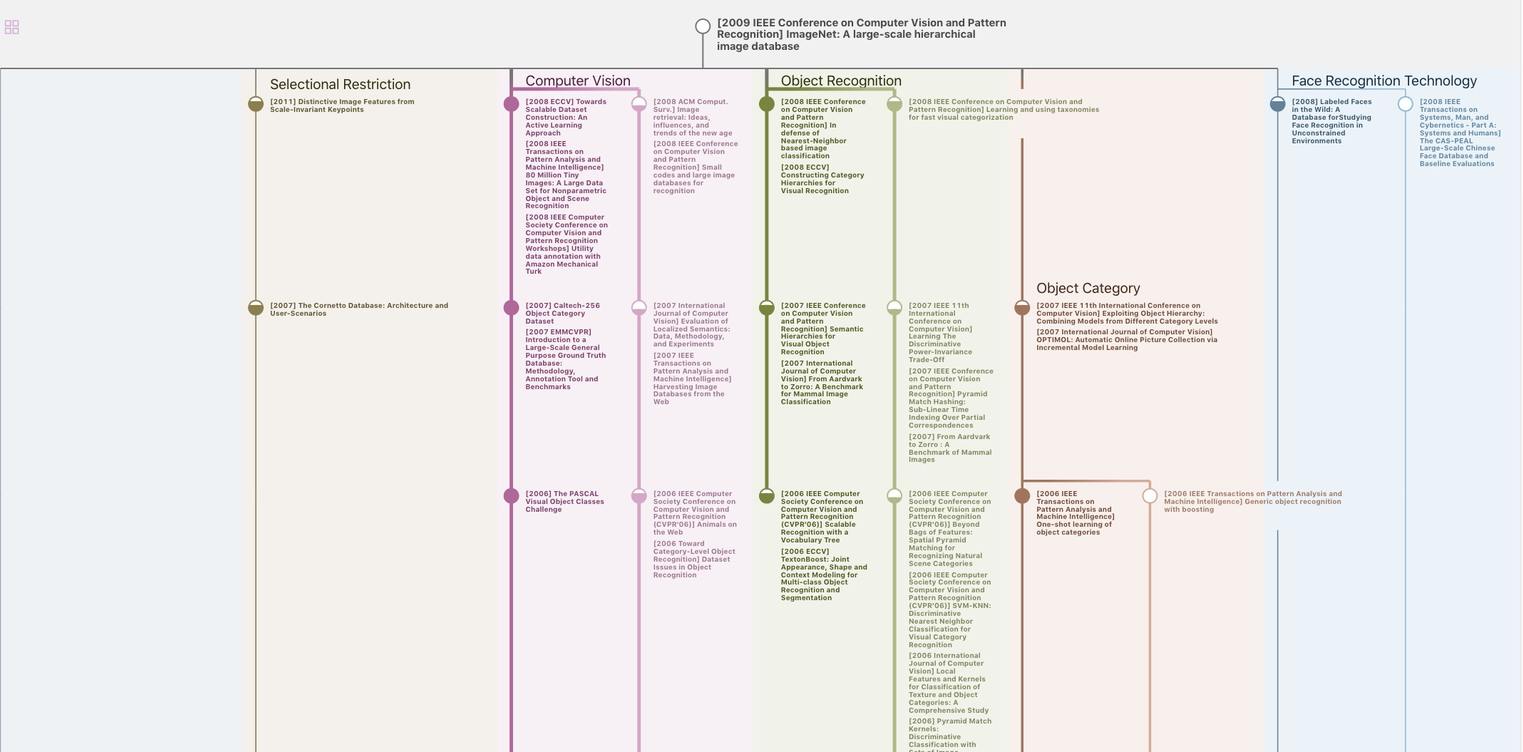
生成溯源树,研究论文发展脉络
Chat Paper
正在生成论文摘要