Tree-Based Credit Card Fraud Detection Using Isolation Forest, Spectral Residual, and Knowledge Graph.
LOD (2)(2022)
摘要
Credit card frauds have constantly been a significant threat to the worldwide economy since the advent of non-cash payments, leading to high demand for fraud detection research. Almost existing studies on supervised credit card fraud classifiers cannot leverage domain characteristics such as fraud rings and sudden changes in payment traffic. They also often fail to detect unseen fraud patterns. In this paper, we introduce a set of features, targeting both traditional and modern fraud patterns, generated from Isolation Forest, Spectral Residual, and Knowledge Graph to boost the performance of tree-based models. We evaluate different tree-based models on the Kaggle public dataset and demonstrate the enhancement of our feature scores in the enterprise payment context. The proposed feature set increases the AUC-ROC and AUC-PR of XGBoost by up to 4.5% and 47.89%, respectively, on the enterprise dataset with only 0.0028% of fraud samples. Our results suggest a method for further improvement on a wide range of fraud detection problems based on domain analysis and feature extractors, especially Knowledge Graph.
更多查看译文
关键词
Credit card fraud detection, Cash-out, Supervised learning, Unsupervised learning, Knowledge graph, Feature construction
AI 理解论文
溯源树
样例
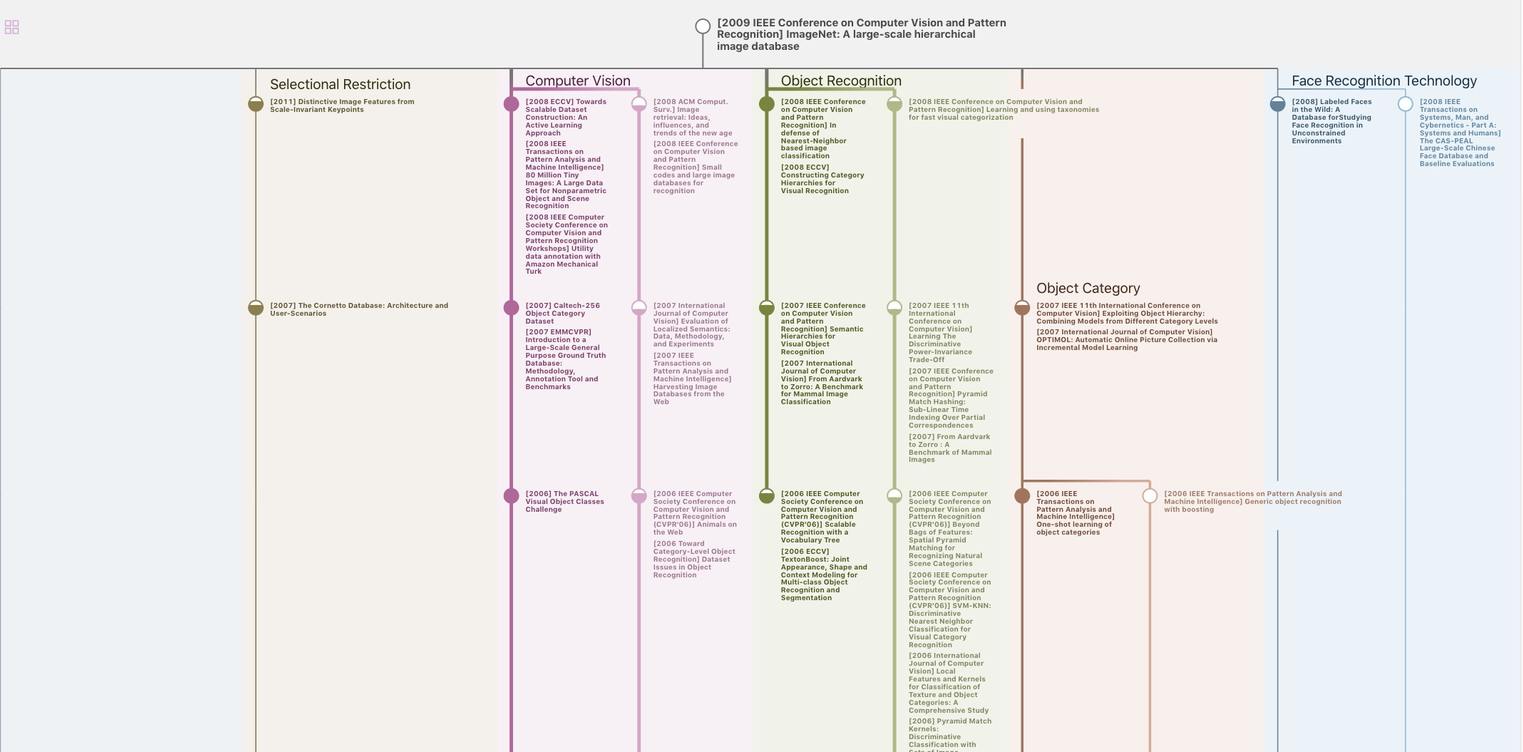
生成溯源树,研究论文发展脉络
Chat Paper
正在生成论文摘要