Deep Neural Network Piration without Accuracy Loss.
ICMLA(2022)
摘要
A deep neural network (DNN) classifier is often viewed as the intellectual property of a model owner due to the huge resources required to train it. To protect intellectual property, the model owner can embed a watermark into the DNN classifier (called target classifier) such that it outputs pre-determined labels (called trigger labels) for pre-determined inputs (called trigger inputs). Given the black-box access to a suspect classifier, the model owner can verify whether the suspect classifier is pirated version of its classifier by first querying the suspect classifier for trigger inputs and then checking whether the predicted labels match with the trigger labels. Many studies showed that an attacker can pirate the target classifier (called pirated classifier) via retraining or fine-tuning the target classifier to remove its watermark. However, they sacrifice the accuracy of the pirated classifier, which is undesired for critical applications such as finance and healthcare. In our work, we propose a new attack without sacrificing the accuracy of the pirated classifier for in-distribution testing inputs while preventing the detection from the model owner. Our idea is that an attacker can detect the trigger inputs in the inference stage of the pirated classifier. In particular, given a testing input, we let the pirated classifier return a random label if the input is detected as a trigger input. Otherwise, the pirated classifier predicts the same label as the target classifier. We evaluate our attack on benchmark datasets and find that our attack can effectively identify the trigger inputs. Our attack reveals that the intellectual property of a model owner can be violated with existing watermarking techniques, highlighting the need for new techniques.
更多查看译文
关键词
Deep Neural Networks, Intellectual Property, Watermarking
AI 理解论文
溯源树
样例
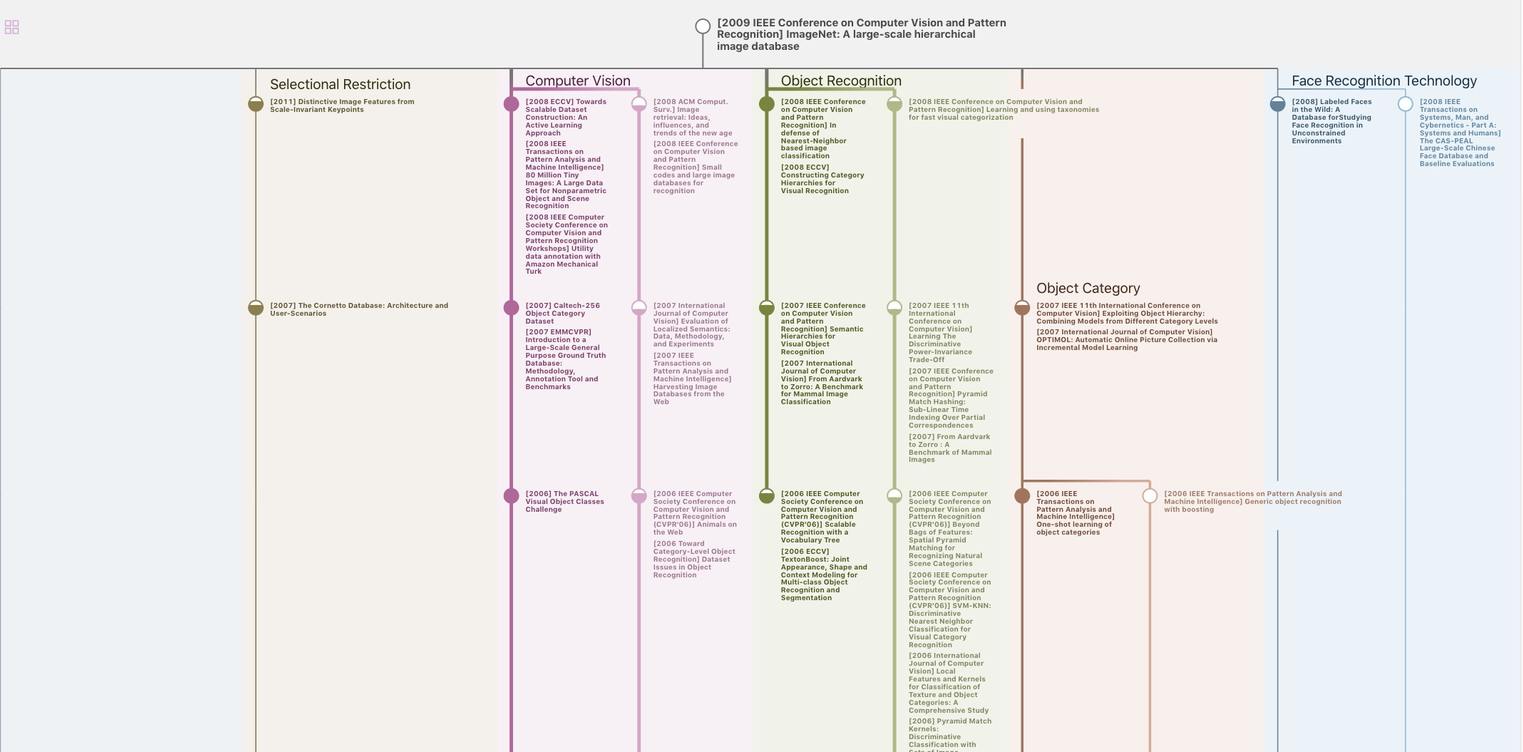
生成溯源树,研究论文发展脉络
Chat Paper
正在生成论文摘要