Towards Fairness and Interpretability: Clinical Decision Support for Acute Coronary Syndrome.
ICMLA(2022)
摘要
Machine learning based prediction of acute coronary syndrome positive patients is becoming increasingly common. However, there is little to no research in terms of assessing fairness and interpretability of these methods. This study discusses methodologies to reduce fairness related disparities in acute coronary syndrome predictions made by machine learning algorithms for different demographics sections of the population. Additionally, existing interpretability technique called LIME is used to understand predictions made using fairness methodologies. Our analysis show that discussed methods have: (1) potential to reduce treatment disparities; (2) generalize well to different healthcare setting in the United States; and (3) reduce overall false negative rates by 0.34 and improve AUROC score by 0.11 when compared to algorithms like XGBoost.
更多查看译文
关键词
machine learning,fairness,interpretability,acute coronary syndrome,healthcare
AI 理解论文
溯源树
样例
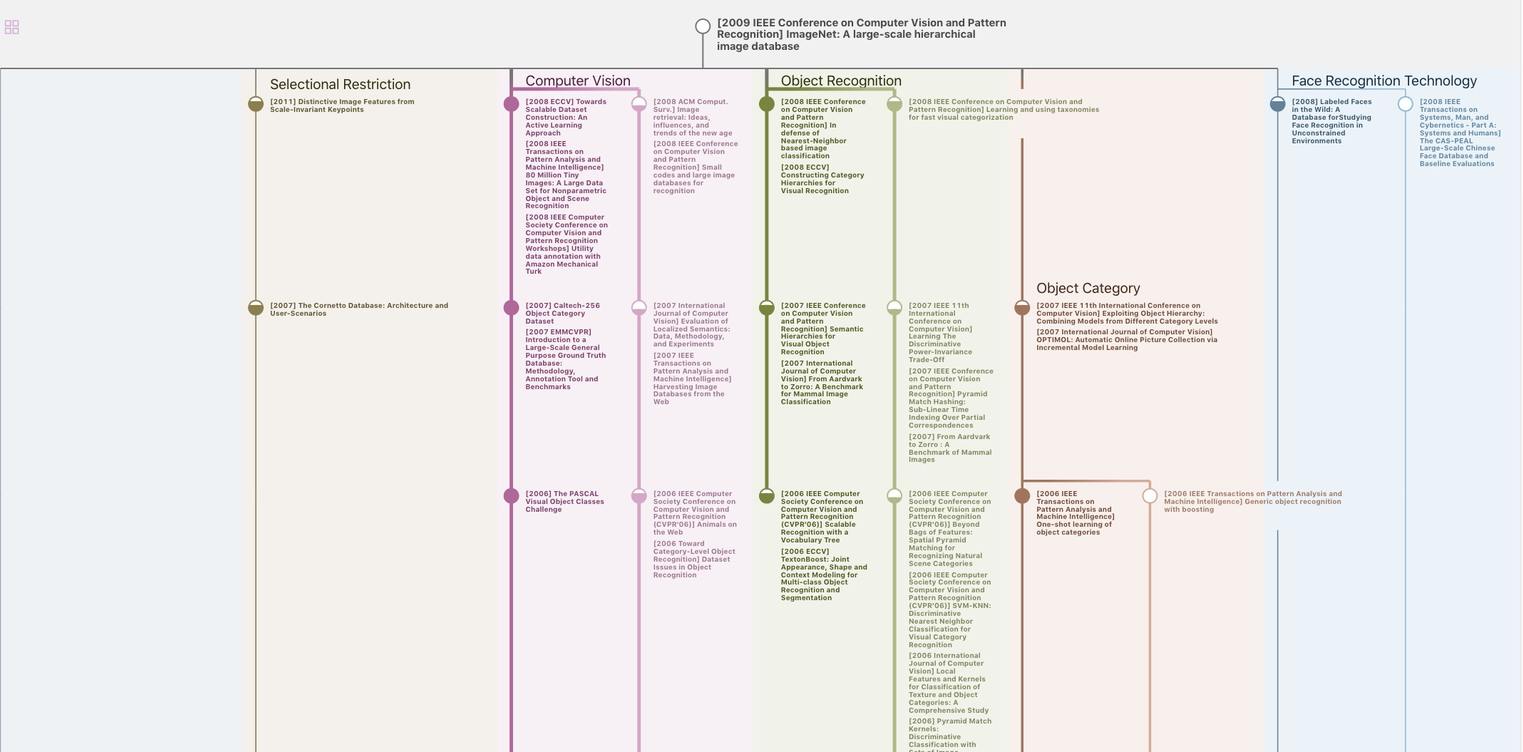
生成溯源树,研究论文发展脉络
Chat Paper
正在生成论文摘要