Missing Value Replacement for PMU Data via Deep Learning Model With Magnitude Trend Decoupling.
IEEE Access(2023)
Abstract
This paper develops a forecasting-based missing value replacement model for Phasor Measurement Unit (PMU) data during power system events. The proposed forecasting model leverages a sequence-to-sequence (Seq2Seq) long short-term memory (LSTM) network with an attention mechanism, which is trained with both pre-event and post-event data. The trained forecasting model is utilized to accurately estimate and recover missing measurements in PMU data. To improve the accuracy of the proposed model, we introduced two novel techniques: 1) integrating a prior knowledge matrix into the attention mechanism that effectively preserves correlations within PMU data, and 2) decoupling the magnitude and trend components of the residual forecast so that such forecasting models are separately trained, which boosts the resistance to the noisy signal. Numerical studies on real-world PMU data collected from the North American electric power grid demonstrate that our proposed model achieves 5% to 30% error reduction compare to the baseline models for all key power grid measurements in both root mean square error and mean absolute error metrics. Furthermore, our model exhibits robust missing data recovery performance even when nearly all of the grid event data is missing.
MoreTranslated text
Key words
Phasor measurement units,Predictive models,Data models,Forecasting,Power systems,Current measurement,Power measurement,machine learning,deep learning,missing value replacement,phasor measurement unit,grid disturbance,multivariate time series
AI Read Science
Must-Reading Tree
Example
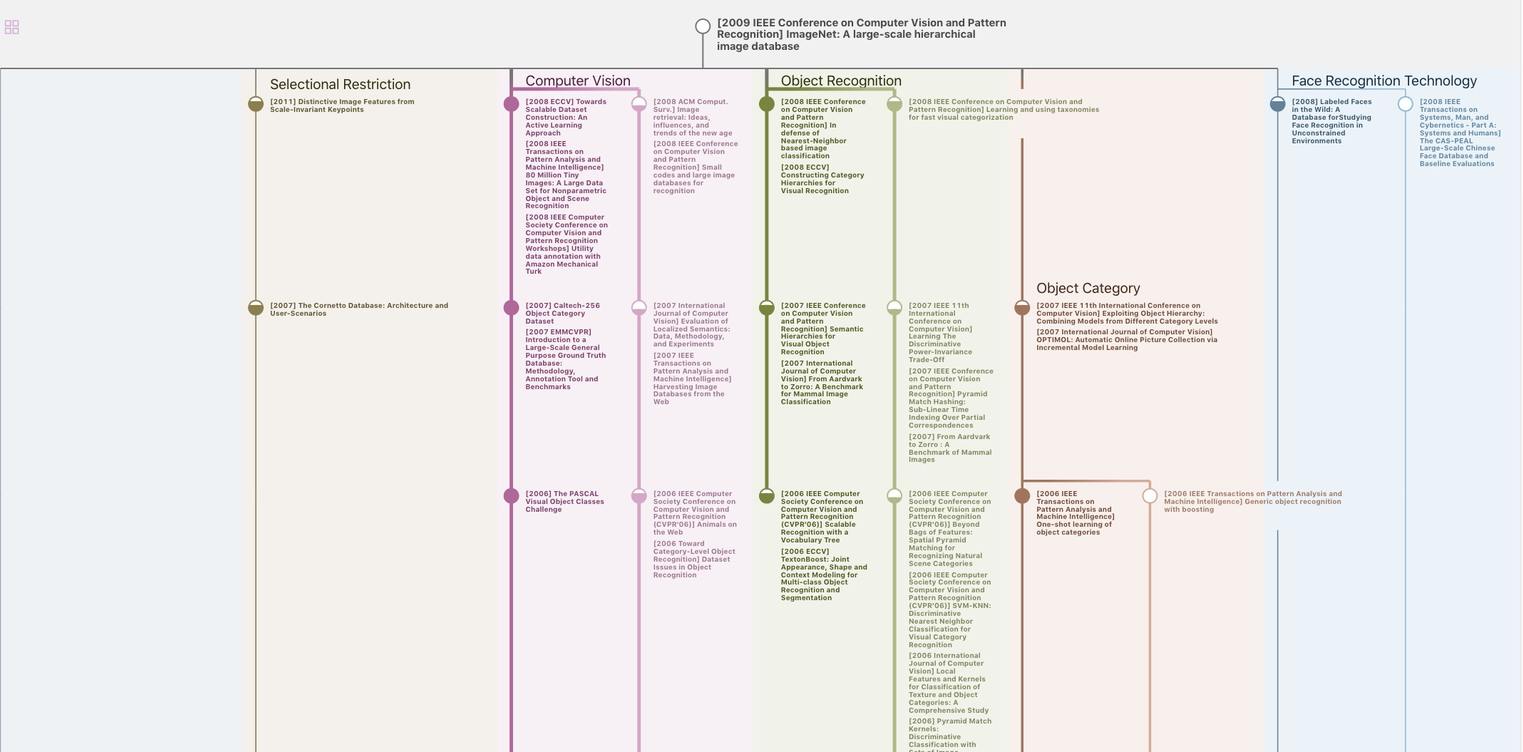
Generate MRT to find the research sequence of this paper
Chat Paper
Summary is being generated by the instructions you defined