Deep Learning Techniques for Visual SLAM: A Survey.
IEEE Access(2023)
摘要
Visual Simultaneous Localization and Mapping (VSLAM) has attracted considerable attention in recent years. This task involves using visual sensors to localize a robot while simultaneously constructing an internal representation of its environment. Traditional VSLAM methods involve the laborious hand-crafted design of visual features and complex geometric models. As a result, they are generally limited to simple environments with easily identifiable textures. Recent years, however, have witnessed the development of deep learning techniques for VSLAM. This is primarily due to their capability of modeling complex features of the environment in a completely data-driven manner. In this paper, we present a survey of relevant deep learning-based VSLAM methods and suggest a new taxonomy for the subject. We also discuss some of the current challenges and possible directions for this field of study.
更多查看译文
关键词
Deep learning,Simultaneous localization and mapping,Estimation,Visualization,Visual odometry,Feature extraction,Learning systems,Location awareness,Robots,Taxonomies,Robot sensing systems,Visual SLAM,deep learning,joint learning,active learning,survey
AI 理解论文
溯源树
样例
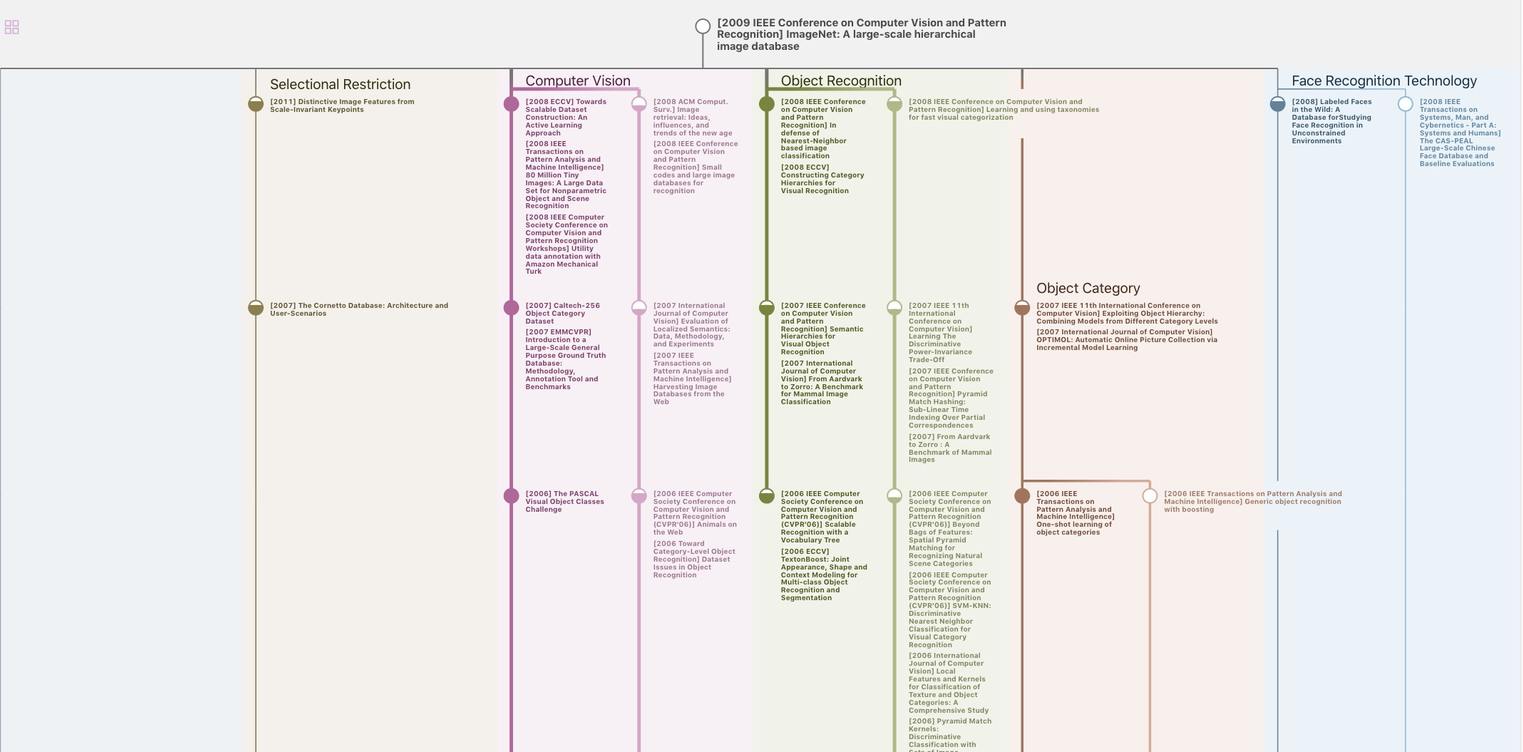
生成溯源树,研究论文发展脉络
Chat Paper
正在生成论文摘要