Robust Perception for Autonomous Vehicles using Dimensionality Reduction
TrustCom(2022)
Abstract
Adversarial attacks on machine learning models have proven to be a major contributor for the lack of actual deployment and adoption of ML in many practical use-cases. They have been found to be equally lethal in video streams as they are in images and texts. This finds particular relevance in the domain of autonomous driving tasks, which make use of object recognition neural architectures. In this paper, we take a step back from the cat and mouse chase of novel attacks and ad-hoc defenses and try to explain adversarial attacks from the perspective of the geometry of the high-dimensional spaces that the models operate in. Additionally, we make use of our idea of relating adversarial attacks to dimensionality to propose a counter-measure that uses dimension reduction. We have tested our proposition on state-of-the-art object detection and classification models on video streams including Faster-RCNN and YOLO and corresponding adversarial attacks on these models. Having optimally tuned the hyper-parameter associated with variability preservation upon dimension reduction using simple Singular Value Decomposition, we have shown that the performance of the robust version of the object detector models is within 2- 3% of that on the clean samples, despite the presence of adversarial perturbation.
MoreTranslated text
Key words
Deep learning,Neural networks,Adversarial Attacks,video streams,object recognition
AI Read Science
Must-Reading Tree
Example
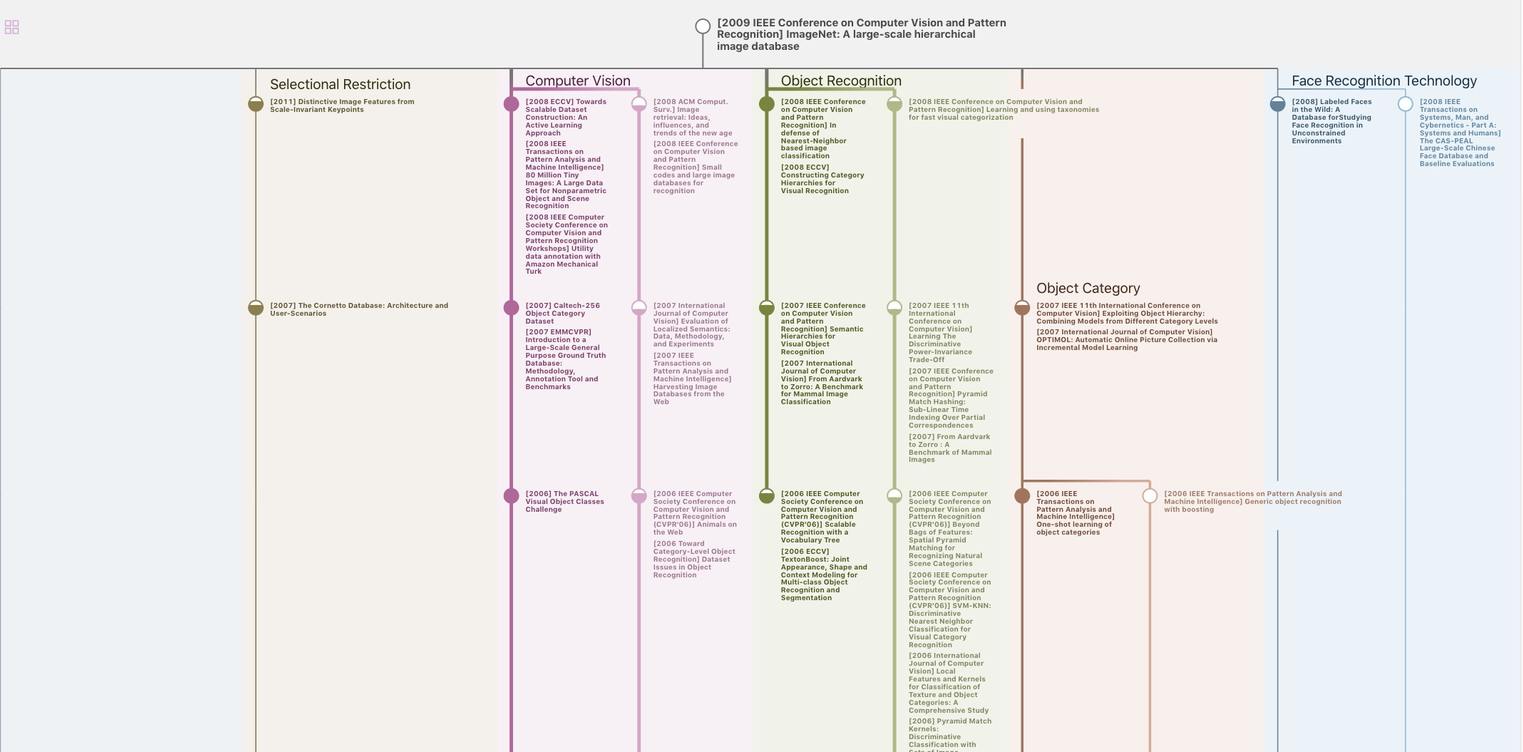
Generate MRT to find the research sequence of this paper
Chat Paper
Summary is being generated by the instructions you defined