Measurement of Malware Family Classification on a Large-Scale Real-World Dataset.
TrustCom(2022)
Abstract
There are many review articles on malware analysis, which provide a comprehensive summary of the features, methods, and challenges of malware analysis. But these are limited to a theoretical overview. The purpose of this paper is to take malware family classification as an example, to restore and display the malware analysis in real scenarios.In this paper, the measurement of malware family classification is carried out on a large-scale dataset in the real world. We use the BODMAS dataset, which contains a total of 57,293 malware samples, with carefully curated family information (581 families). Referring to the common features (including static features and dynamic features) and machine learning methods mentioned in the review articles, we conduct feature extraction and classification experiments. Then, we summarize the classification results. Static features can efficiently classify a large number of samples, even with 10% packed samples. In real scenarios, the family distribution is extremely unbalanced, and the family classification results with a large number of samples are better. Different evaluation methods have different interpretations of the classification results. These measurement results provide a basis for further malware analysis in real applications.
MoreTranslated text
AI Read Science
Must-Reading Tree
Example
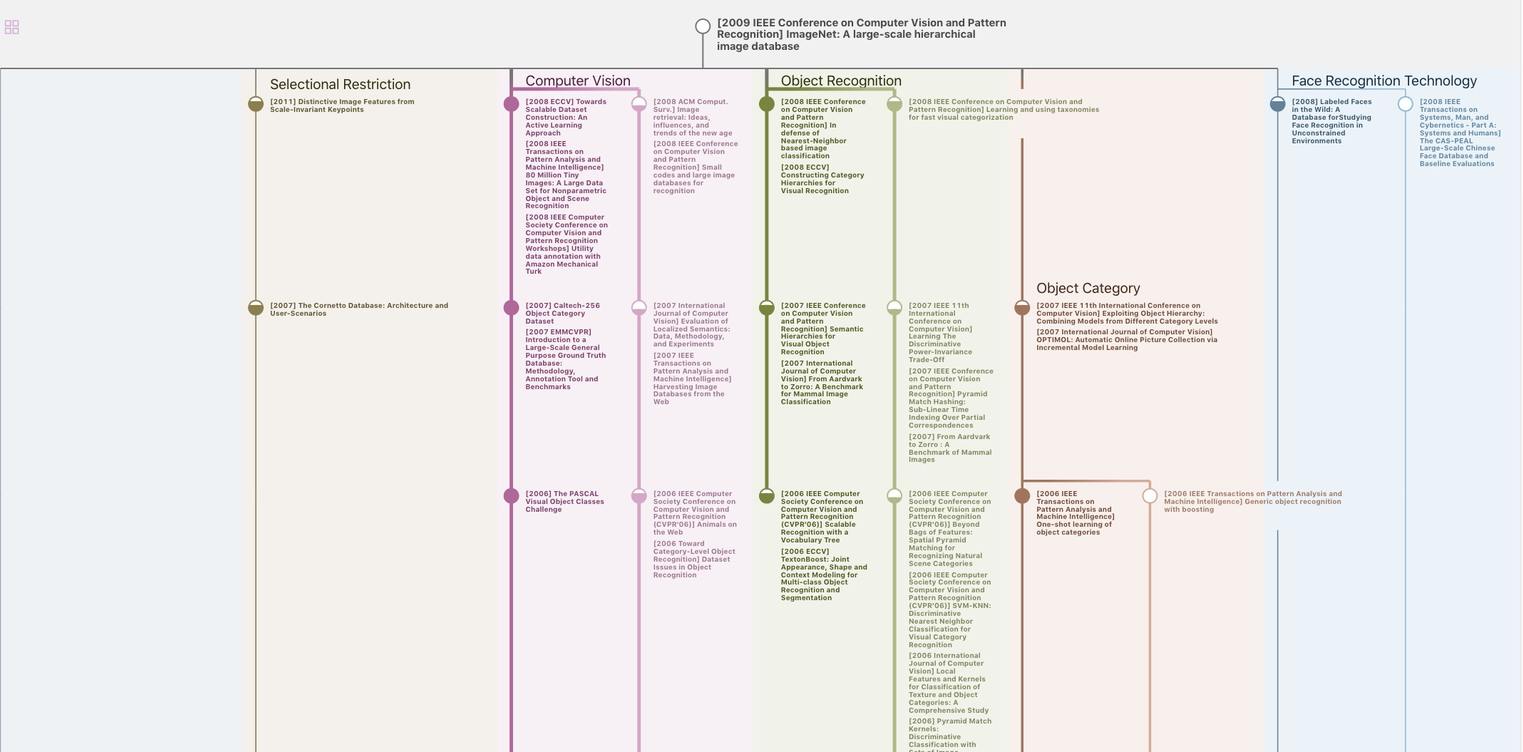
Generate MRT to find the research sequence of this paper
Chat Paper
Summary is being generated by the instructions you defined