A 28nm 11.2TOPS/W Hardware-Utilization-Aware Neural-Network Accelerator with Dynamic Dataflow.
ISSCC(2023)
摘要
With the rapid evolution of AI technology, various neural network structures have been developed for diverse applications. As a typical ease, Fig. 22.4.1 shows that the convolution (Conv) layer used in the convolutional neural networks (CNNs) features distinct shapes and types. Neural network accelerators with high peak energy efficiency have been demonstrated [1–4]. However, they usually suffer decreased hardware (mainly multiply-accumulate (MAC) units) utilization for various network structures, which reduces the attainable energy efficiency accordingly. To improve the MAC utilization, the Nvidia deep learning accelerator (NVDLA) [5] applies hardware parallelism along the channel direction, but the MAC utilization is still low for the shallow layers. According to our experiments, NVDLA achieves 23% MAC utilization in the worst case. A Scatter-Gather scheme [4] is utilized to mitigate the utilization drop for shallow layers by rearranging the input features (IF), but the improvement is limited. As depthwise convolution (Dwcv) has been widely used, the accompanying low MAC utilization also needs to be considered. Taking MobileNetV2 as an example, NVDLA only achieves 0.4% utilization for Dwcv. To address these critical issues, this work presents a utilization-aware neural network accelerator, which can dynamically change the level of parallelism along multiple dimensions to maximize the MAC utilization. The chip achieves
$> 97.3{\%}$
MAC utilization on benchmark networks while delivering
$4.7\times$
higher attainable energy efficiency than state-of-the-art designs [1–4].
更多查看译文
关键词
attainable energy efficiency,benchmark networks,convolution layer,convolutional neural networks,depthwise convolution,dynamic dataflow,hardware-utilization-aware neural-network accelerator,high peak energy efficiency,low MAC utilization,MAC utilization,MobileNetV2,multiply-accumulate units,neural network structures,Nvidia deep learning accelerator,Scatter-Gather scheme,size 28.0 nm,utilization drop,utilization-aware neural network accelerator
AI 理解论文
溯源树
样例
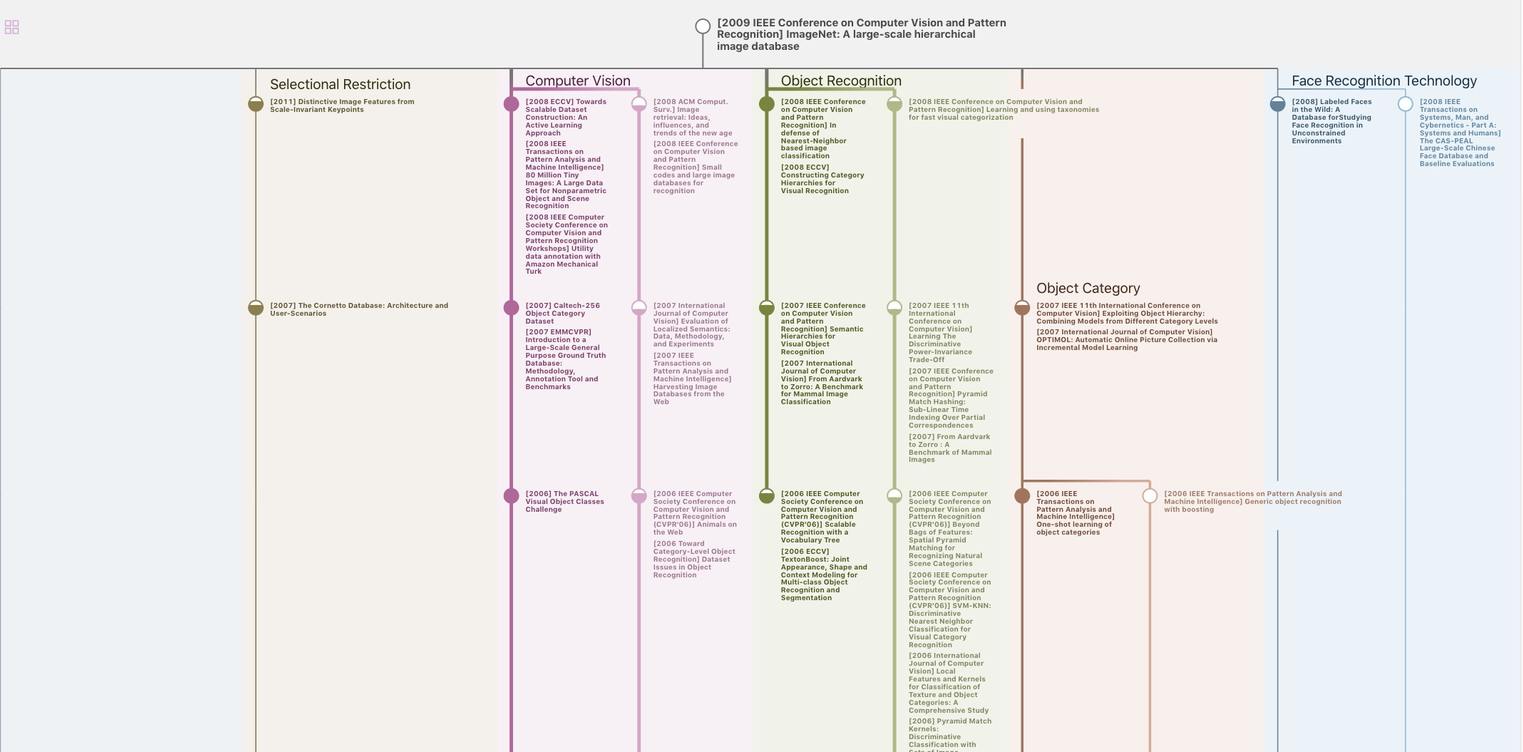
生成溯源树,研究论文发展脉络
Chat Paper
正在生成论文摘要