Efficient Deep Reinforcement Learning via Policy-Extended Successor Feature Approximator.
DAI(2022)
摘要
Successor Features (SFs) improve the generalization of Reinforcement Learning across unseen tasks by decoupling the dynamics of the environment from the rewards. However, the decomposition highly depends on the policy learned on the task, which may not be optimal in other tasks. To improve the generalization of SFs, in this paper, we propose a novel SFs learning paradigm, Policy-extended Successor Feature Approximator (PeSFA) which decouples the SFs from the policy by learning a policy representation module and inputting the policy representation to SFs. In this way, when we fit SFs well in the policy representation space, we can directly obtain a better SFs corresponding to any task by searching the policy representation space. Experimental results show that PeSFA significantly improves the generalizability of SFs and accelerates the learning process in two representative environments.
更多查看译文
关键词
Reinforcement learning, Transfer learning, Successor features, Policy representation
AI 理解论文
溯源树
样例
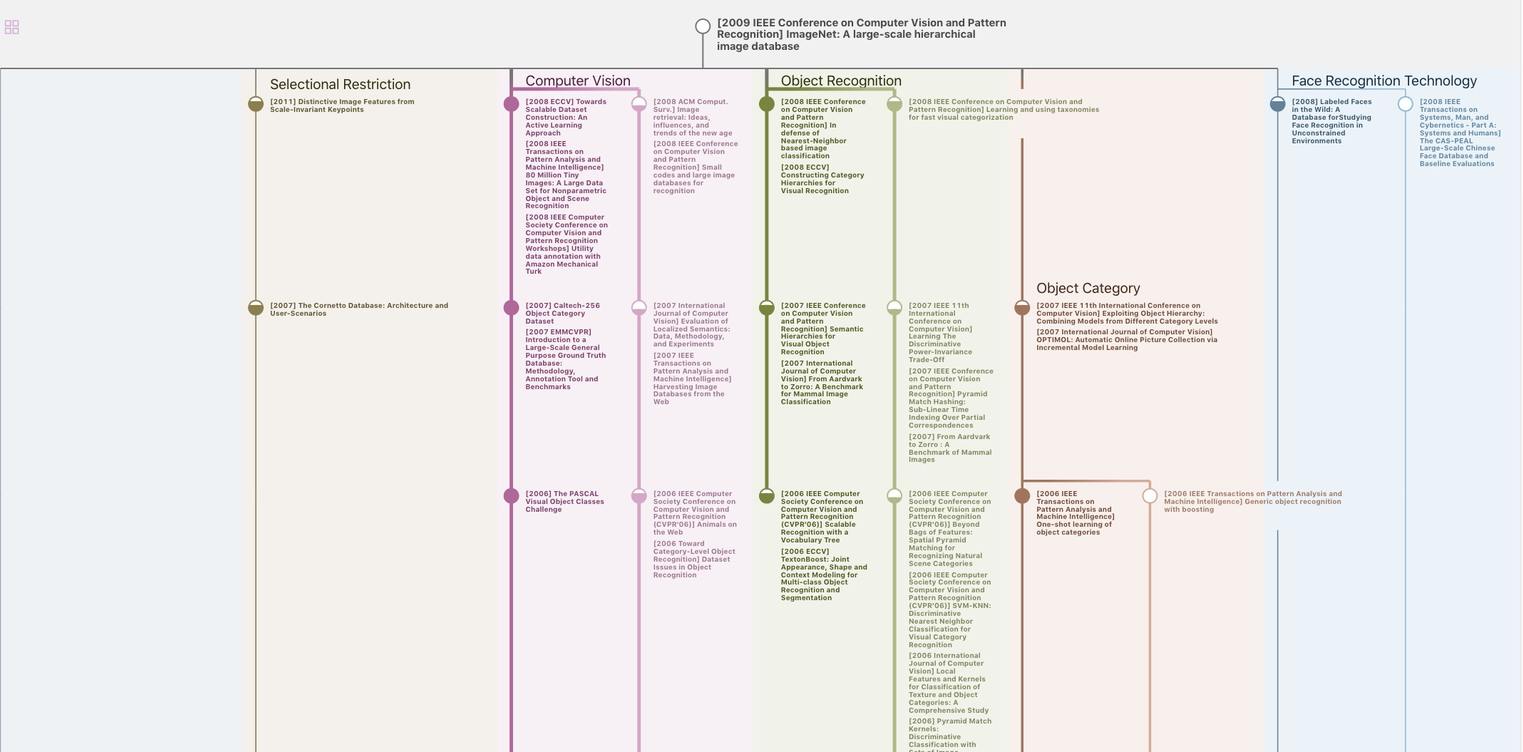
生成溯源树,研究论文发展脉络
Chat Paper
正在生成论文摘要