Action-Manipulation Attack and Defense to X-Armed Bandits.
International Conference on Trust, Security and Privacy in Computing and Communications(2022)
摘要
As a continuous variant of Multi-armed bandits (MAB), $\mathcal{X}$-armed bandits have enriched many applications of online machine learning like personalized recommendation system. However, the attack and defense to the $\mathcal{X}$-armed bandits remain largely unexplored, though the MAB has proved to be vulnerable. In this paper, we aim to bridge this gap and investigate the robustness analysis for the $\mathcal{X}$-armed bandits. Specifically, we consider action-manipulation attack, which is practical but harder than the existing reward-manipulation attack. We propose an attack algorithm based on a lower bound tree (LBT), which can continuously hijack the learner’s action by perturbing $\mathcal{X}$-armed bandits’ high confidence tree (HCT) construction. As a result, the nodes including the arm targeted by the attacker is selected frequently with a sublinear attack cost. To defend against the LBT attack, we propose a robust version of the HCT algorithm, called RoHCT. We theoretically analyze that the regret of RoHCT is related to the upper bound of the total cost Q and still sublinear to total number of rounds T. We carry out experiments to evaluate the effectiveness of LBT and RoHCT.
更多查看译文
关键词
χ-armed bandits,action-manipulation attack,defense,robustness
AI 理解论文
溯源树
样例
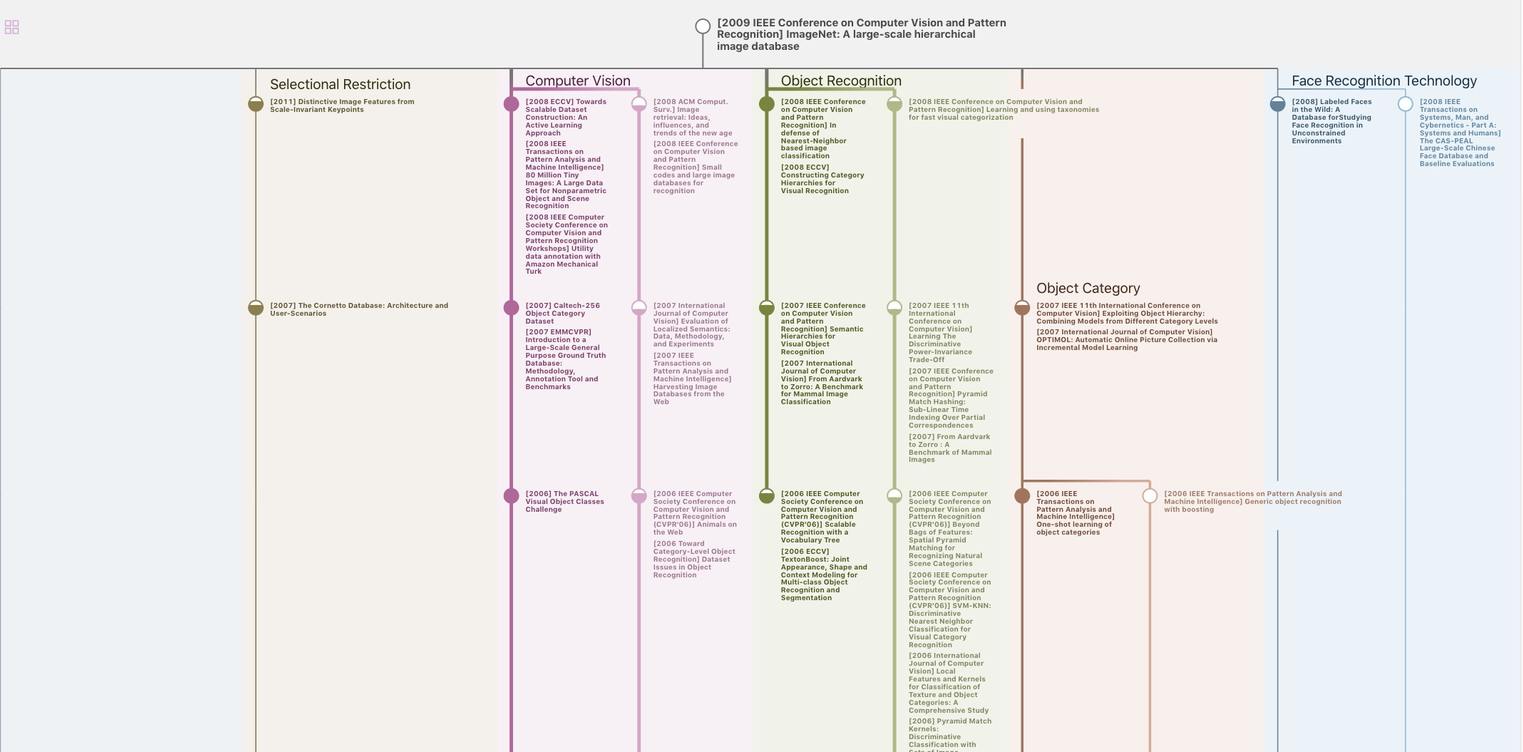
生成溯源树,研究论文发展脉络
Chat Paper
正在生成论文摘要