APT Attribution for Malware Based on Time Series Shapelets.
TrustCom(2022)
摘要
To discover and defend against APT attacks more efficiently, we need to conduct binary analysis and source tracing research on APT malicious codes. This paper attributes APT groups for malicious codes from the perspective of binary similarity. First, we innovatively select the local features of the binary functions for classification and apply time series mining techniques to the mining of sequences of basic blocks (called paths). The Shapelet model selects path shapelets, which are path fragments that can best represent paths and are used to distinguish paths. Path shapelets can provide path-level interpretability for classification. Second, we use API calls to filter functions and generate paths of interest to reduce resource consumption. To evaluate the proposed method, we collect APT malicious codes based on publicly available threat intelligence reports. Our method filters 92.82% of functions and generates an average of 1.37 paths per function. The classification effect has obvious advantages over other methods.
更多查看译文
关键词
n/a
AI 理解论文
溯源树
样例
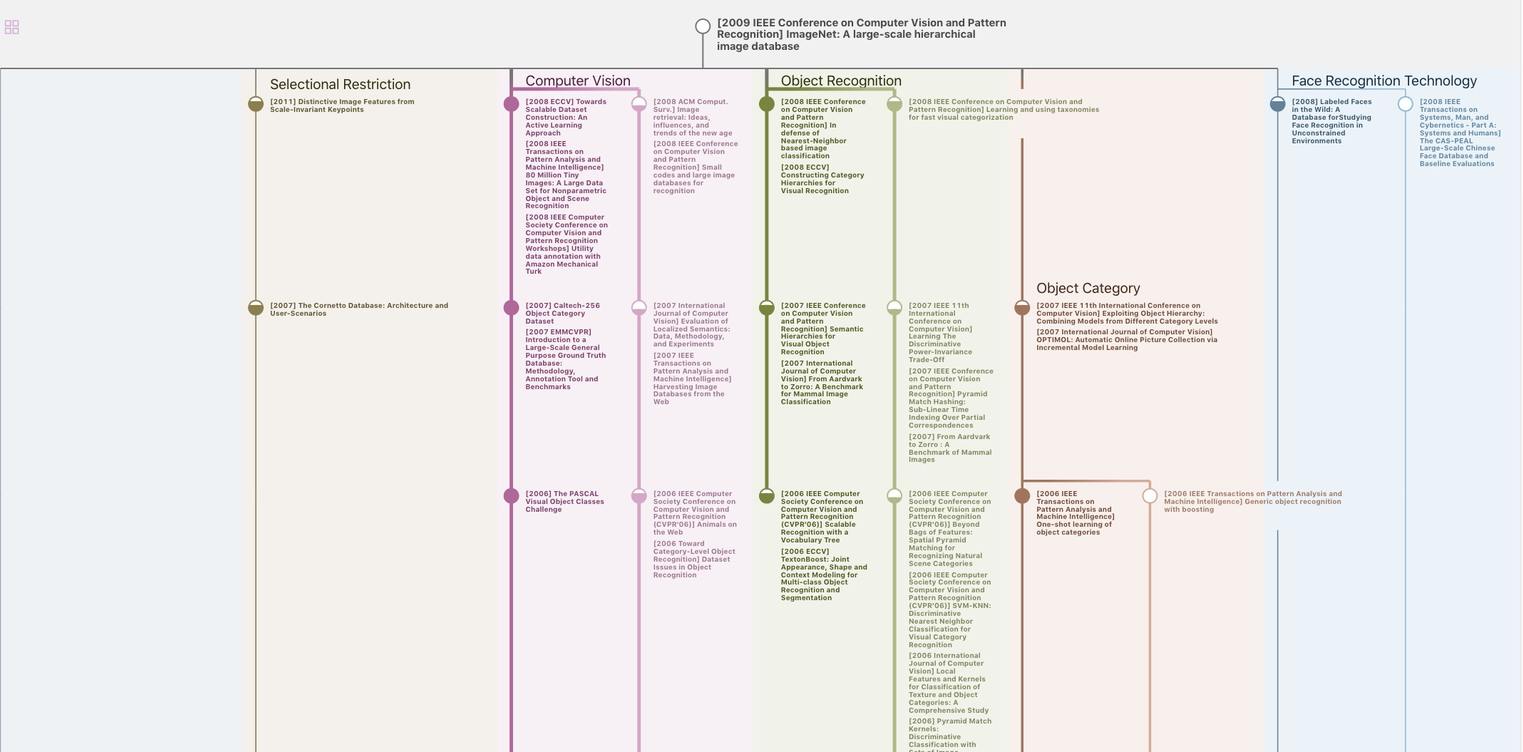
生成溯源树,研究论文发展脉络
Chat Paper
正在生成论文摘要