A Hybrid Optimization and Deep Learning Algorithm for Cyber-resilient DER Control.
ISGT(2023)
摘要
With the proliferation of distributed energy resources (DERs) in the distribution grid, it is a challenge to effectively control a large number of DERs resilient to the communication and security disruptions, as well as to provide the online grid services, such as voltage regulation and virtual power plant (VPP) dispatch. To this end, a hybrid feedback-based optimization algorithm along with deep learning forecasting technique is proposed to specifically address the cyber-related issues. The online decentralized feedback-based DER optimization control requires timely, accurate voltage measurement from the grid. However, in practice such information may not be received by the control center or even be corrupted. Therefore, the long short-term memory (LSTM) deep learning algorithm is employed to forecast delayed/missed/attacked messages with high accuracy. The IEEE 37-node feeder with high penetration of PV systems is used to validate the efficiency of the proposed hybrid algorithm. The results show that 1) the LSTM-forecasted lost voltage can effectively improve the performance of the DER control algorithm in the practical cyber-physical architecture; and 2) the LSTM forecasting strategy outperforms other strategies of using previous message and skipping dual parameter update.
更多查看译文
关键词
cyber-resilient algorithm, distributed energy resources (DERs), DER control, LSTM, Deep learning
AI 理解论文
溯源树
样例
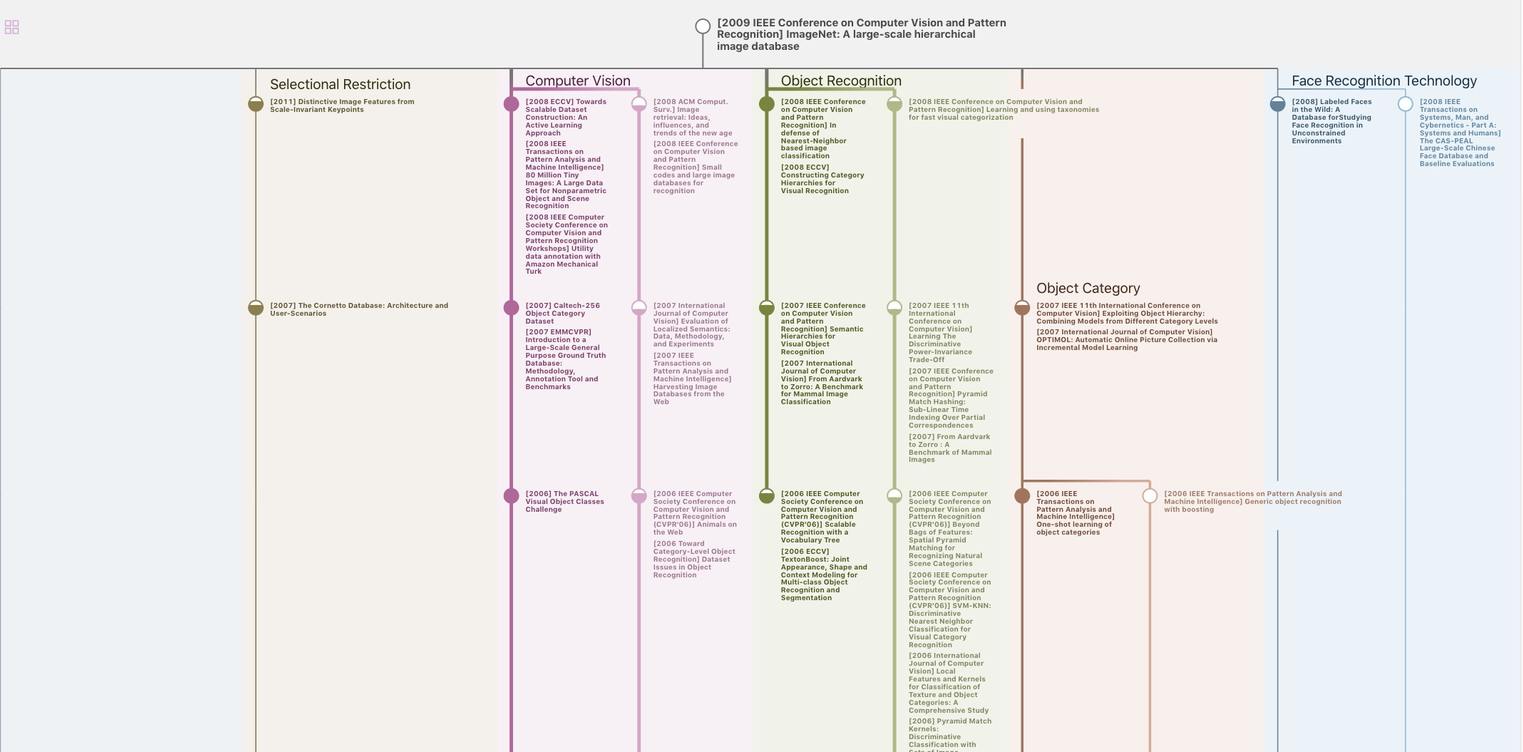
生成溯源树,研究论文发展脉络
Chat Paper
正在生成论文摘要