Time-Domain Elastic Full Waveform Inversion with Frequency Normalization.
IEEE TRANSACTIONS ON GEOSCIENCE AND REMOTE SENSING(2023)
关键词
Mathematical models,Time-frequency analysis,Time-domain analysis,Numerical models,Synthetic data,Propagation,Linear programming,Amplitude spectrum,elastic wave,full waveform inversion (FWI),normalization,time domain
AI 理解论文
溯源树
样例
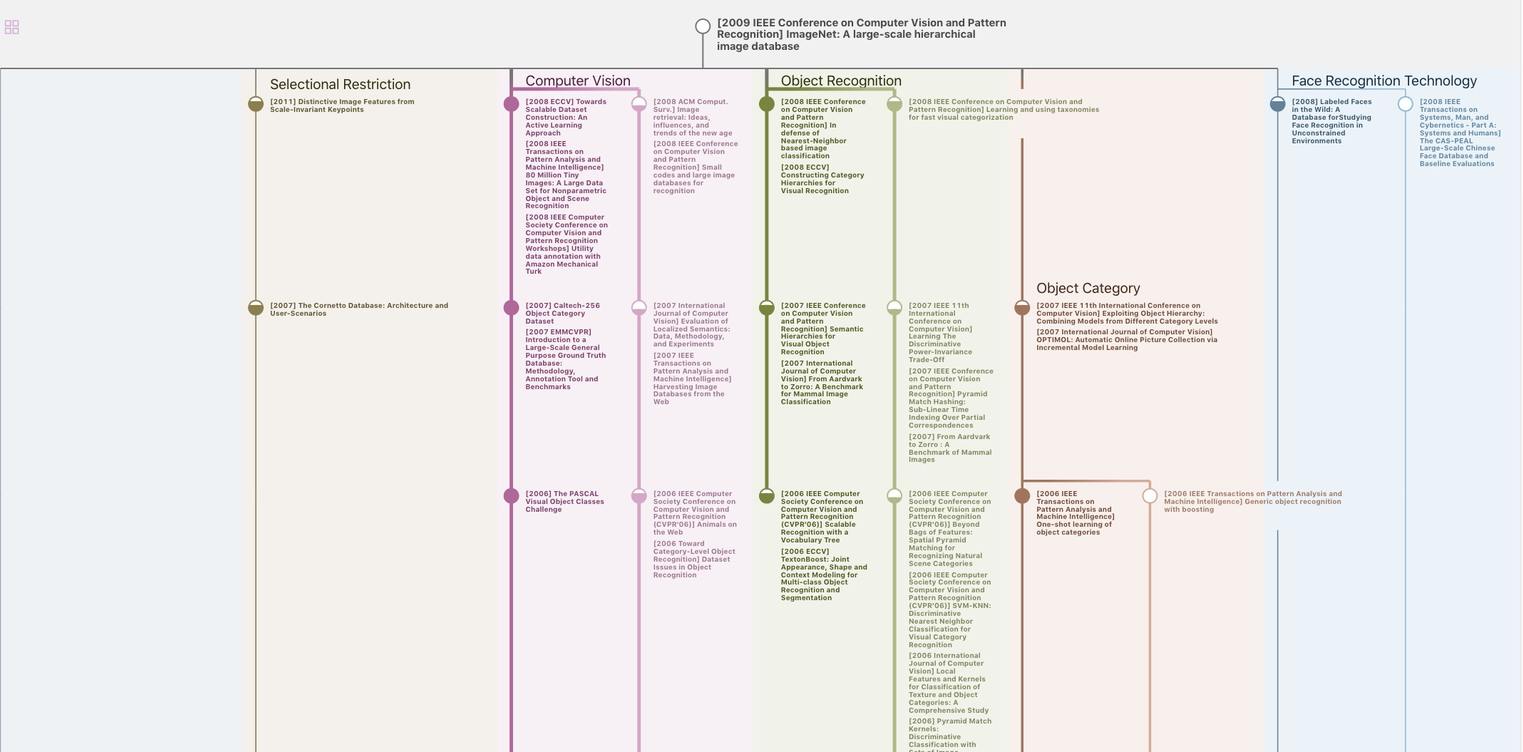
生成溯源树,研究论文发展脉络
Chat Paper
正在生成论文摘要