Improving Zero-Shot Inference with Unsupervised Key-Sentences Extraction.
ICSC(2023)
摘要
In many text applications, low availability of labeled data is common, which makes training machine learning models unfeasible. Many approaches have been proposed to try to circumvent this limitation, with emphasis on zero-shot models. Zero-shot models can perform inference without training. However, they tend to have lower performance in long texts. In this work, we propose to apply an unsupervised technique to rank the most relevant sentences of the long text and apply zero-shot models to these most relevant sentences. Tests on the text classification task demonstrate a performance gain in different types of zero-shot models and different languages.
更多查看译文
关键词
Zero-Shot,Text Labelling,Unsupervised Keyword,Long Text,Embedding Ranking
AI 理解论文
溯源树
样例
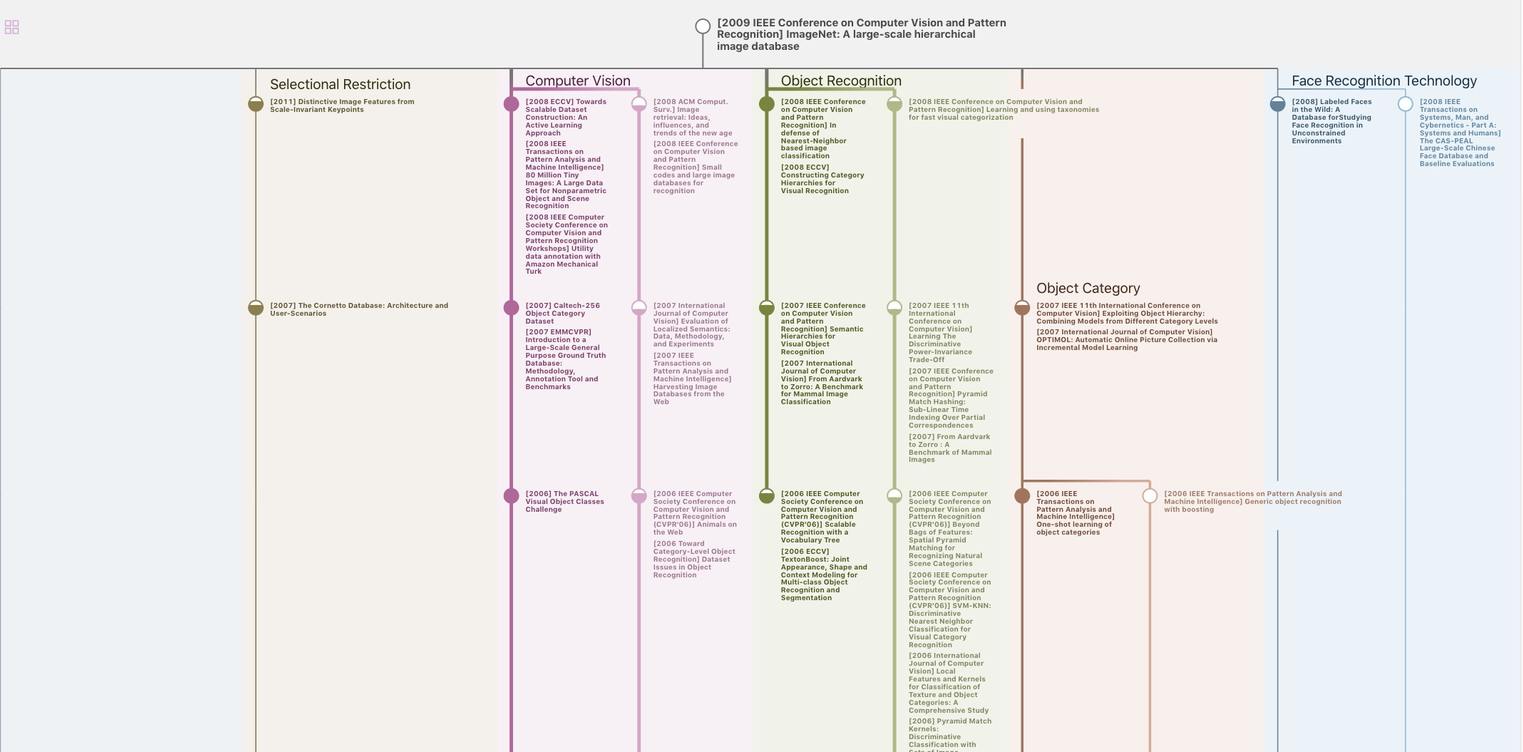
生成溯源树,研究论文发展脉络
Chat Paper
正在生成论文摘要