Does Noise Really Matter? Investigation into the Influence of Noisy Labels on BERT-Based Question Answering System
INTERNATIONAL JOURNAL OF SEMANTIC COMPUTING(2024)
摘要
Recent works with the BERT-based models demonstrate their generalization ability and high performance on the new domain tasks. However, this kind of model requires a large amount of data. Collecting this data can be error-prone, and it is important to know: how the errors in data affect the quality of the model. In this work, we study the impact of data with different errors- noisy data on the training of the question answering-over-text BERT-model. We use the concept of random, structural and irrelevant question noises. We study the robustness of QAT models during the training process with different settings, datasets and noise types and discuss possible reasons. We also propose a real-world domain dataset to probe our findings in a real-world scenario. The results of an experimental study showed that following developed recommendations allowed performance improvement up to 3.6% in a real-world setting.
更多查看译文
关键词
Question-answering system,BERT,noisy data,noise simulation
AI 理解论文
溯源树
样例
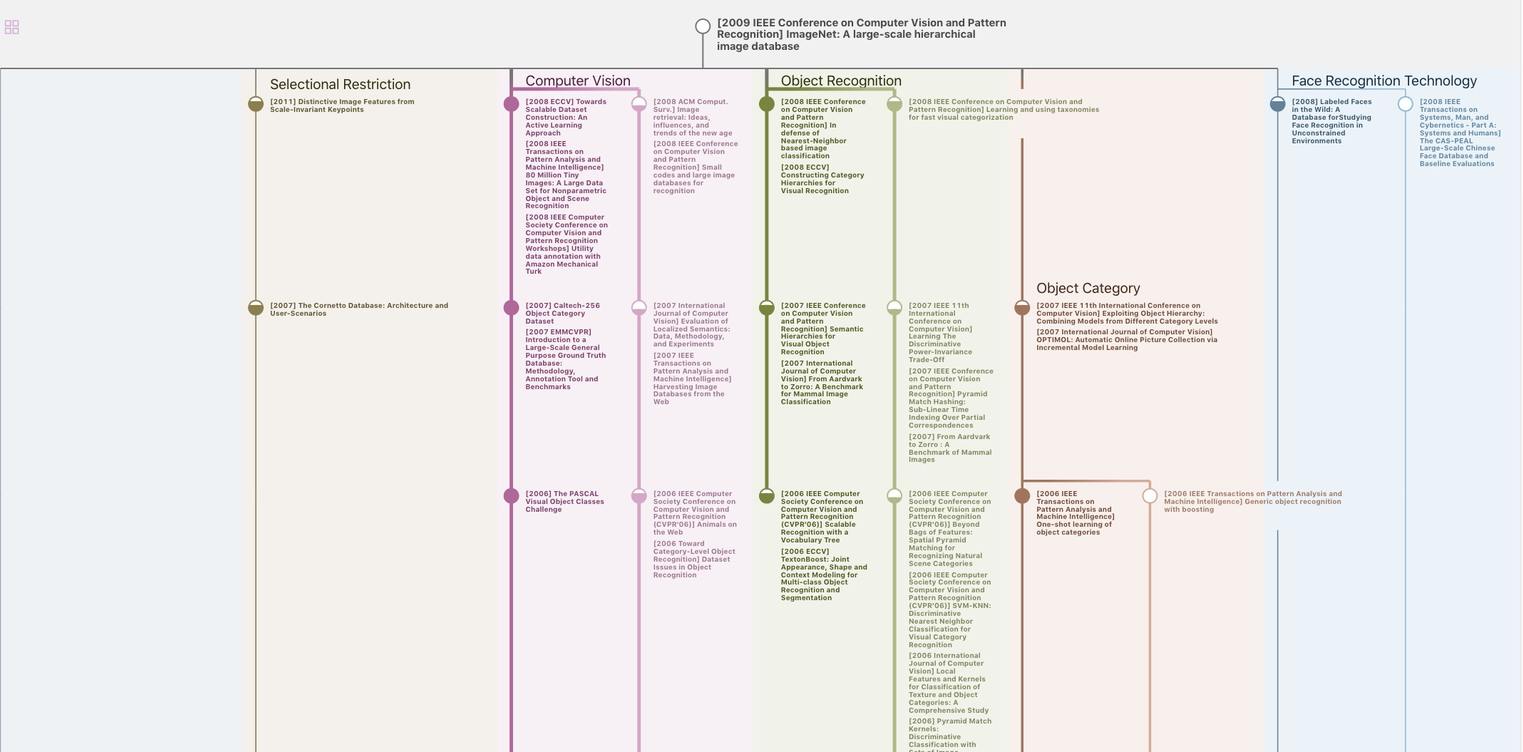
生成溯源树,研究论文发展脉络
Chat Paper
正在生成论文摘要