Communication-Efficient Distributed Learning: An Overview.
IEEE J. Sel. Areas Commun.(2023)
摘要
Distributed learning is envisioned as the bedrock of next-generation intelligent networks, where intelligent agents, such as mobile devices, robots, and sensors, exchange information with each other or a parameter server to train machine learning models collaboratively without uploading raw data to a central entity for centralized processing. By utilizing the computation/communication capability of individual agents, the distributed learning paradigm can mitigate the burden at central processors and help preserve data privacy of users. Despite its promising applications, a downside of distributed learning is its need for iterative information exchange over wireless channels, which may lead to high communication overhead unaffordable in many practical systems with limited radio resources such as energy and bandwidth. To overcome this communication bottleneck, there is an urgent need for the development of communication-efficient distributed learning algorithms capable of reducing the communication cost and achieving satisfactory learning/optimization performance simultaneously. In this paper, we present a comprehensive survey of prevailing methodologies for communication-efficient distributed learning, including reduction of the number of communications, compression and quantization of the exchanged information, radio resource management for efficient learning, and game-theoretic mechanisms incentivizing user participation. We also point out potential directions for future research to further enhance the communication efficiency of distributed learning in various scenarios.
更多查看译文
关键词
Distributed learning,communication efficiency,event-triggering,quantization,compression,sparsification,resource allocation,incentive mechanisms,single-task learning,multitask learning,meta-learning,online learning
AI 理解论文
溯源树
样例
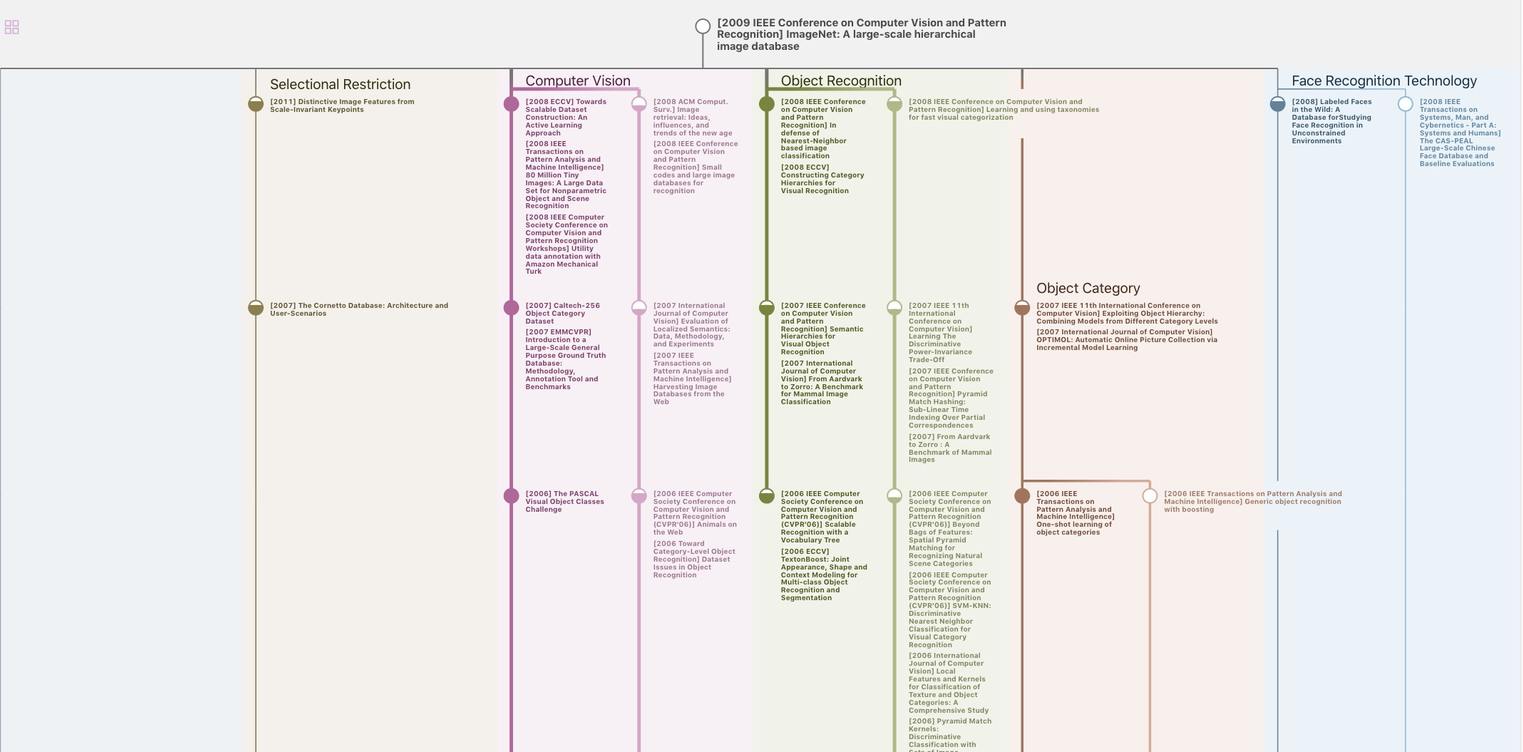
生成溯源树,研究论文发展脉络
Chat Paper
正在生成论文摘要