Online Federated Learning based Object Detection across Autonomous Vehicles in a Virtual World.
CCNC(2023)
摘要
Federated Learning (FL) enables collaborative training of machine learning models for edge devices (e.g., mobile phones) over a network without revealing raw data of the participants. The existing FL benchmarks mostly assume static distribution of local data over time failing to capture the behavior of real-world applications with space-time varying data (e.g., autonomous cars). Our framework addresses this limitation by leveraging popular open source physics simulator (CARLA) and FL framework (OpenFL) to allow collection of streaming data from the mobile agents and feeding them to a practically deployable FL engine for online collaborative training. It also provides the FL researchers with the ability to model data heterogeneity, annotate data with practically zero cost, and perform reproducible continual FL experiments. We believe that this is one of the first attempts to demonstrate online FL on realistic streaming datasets from a virtual world. The demo showcases this framework using a popular object detection use case.
更多查看译文
关键词
Federated Learning,realistic dataset,streaming data,automatic annotation,object detection
AI 理解论文
溯源树
样例
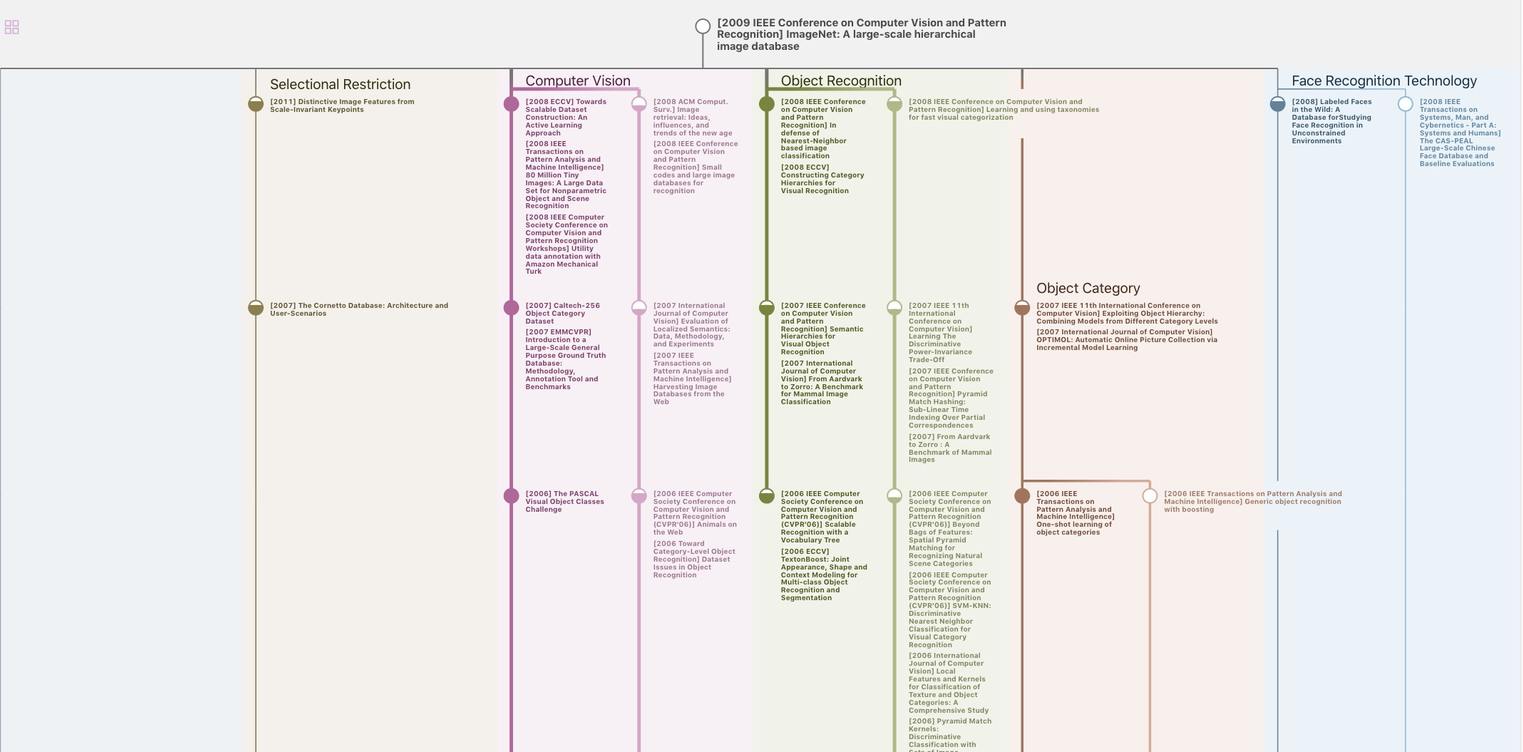
生成溯源树,研究论文发展脉络
Chat Paper
正在生成论文摘要