Client Tuned Federated Learning for RSSI-based Indoor Localisation.
CCNC(2023)
摘要
We apply Federated Learning (FL) to the problem of indoor localisation in a real-world multiple residential house scenario. Fingerprinting of the Received Signal Strength Indicator (RSSI) was used as the localisation method. We show that, given the minimal amount of fine-tuning allowed by constraint on the size of the gradient step in the fit round of FL, a shared model learned this way has strong performance on all houses and is stable with respect to randomness in weight initialisation. Not unexpectedly, the performance is inferior to an individual learning approach. We developed a tuned FL approach - a finetuning step in every round of FL that only affects a subset of the clients' parameters while leaving a common ‘backbone’ unchanged. The FL clients were able to accept the model weights post-tuning or revert to the weights in the previous evaluation round based on their local validation set. Through our extensive evaluation, our results indicate a significant reduction in the performance gap between a completely individual ML and a benchmark traditional FL approach.
更多查看译文
关键词
federated learning,localisation,fingerprinting,e-health
AI 理解论文
溯源树
样例
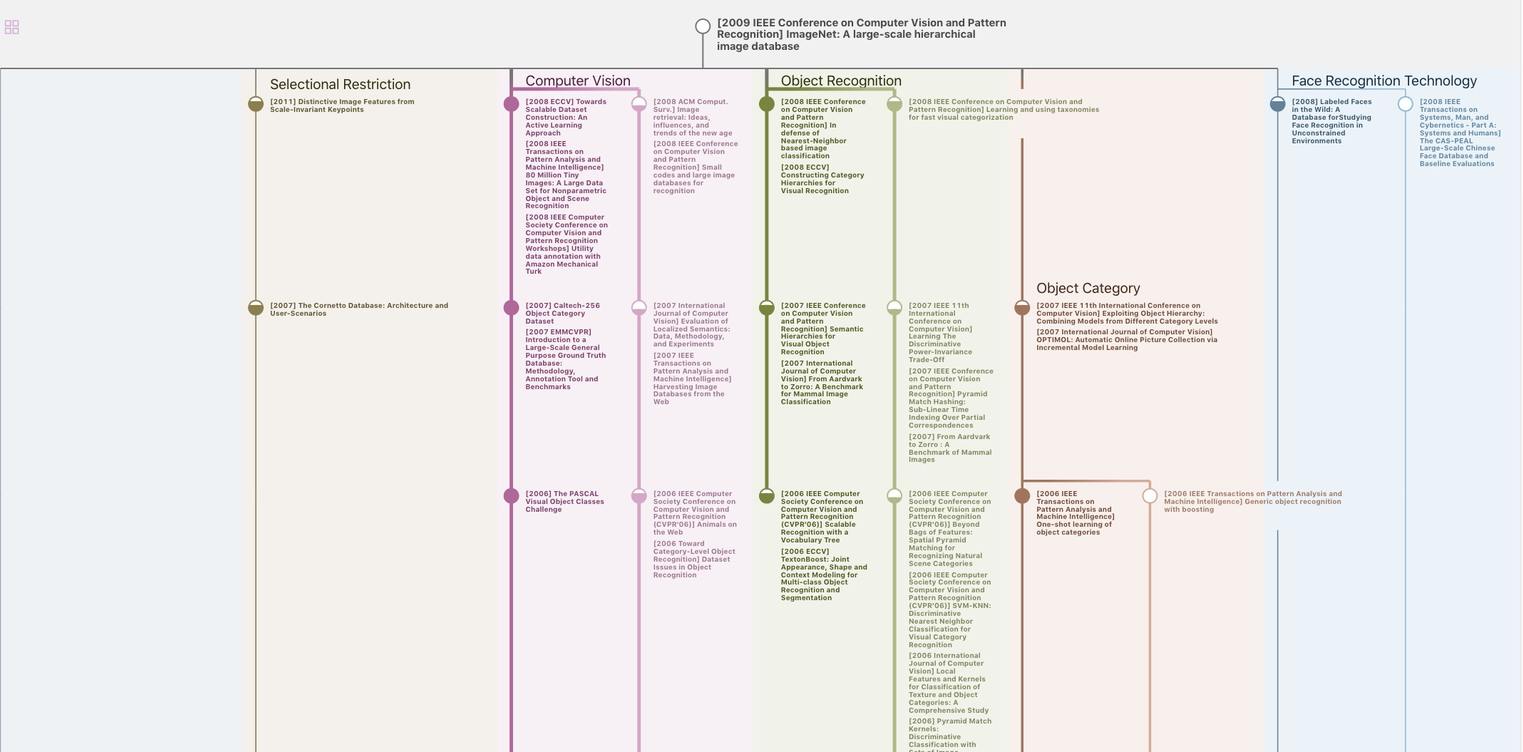
生成溯源树,研究论文发展脉络
Chat Paper
正在生成论文摘要