A Comprehensive Study on Efficient and Accurate Machine Learning-Based Malicious PE Detection.
CCNC(2023)
Abstract
For safe and trustworthy digital services, fast and accurate malware detection is critical. Because of the financial rewards, ransomware assaults are one of the most commonly employed malware variants by cyber criminals. Because of the dynamic environment in which new malware variants arise on a regular basis, it is critical to maintain databases up-to-date in order to protect the digital world from ransomware threats. In this study, we curated the Ransomary dataset containing 2871 ransomware and 4208 benign PE files to allow researchers to use their own algorithms to accomplish fast and precise detection. We examined the Ransomary dataset and compared feature extraction and raw data techniques of static malware analysis. In the EMBER, DeepDetectNet, and Ransomary datasets, we found that effective feature selection with the LightGBM model can yield more than 0.99 AUC. Finally, we demonstrate that using raw data from the first 1KB of PE files may result in an accurate and extremely rapid response time. We intend to continuously expand Ransomary dataset and encourage more researchers to use static, dynamic, or hybrid analysis to identify ransomware more quickly and accurately.
MoreTranslated text
Key words
Malware Analysis,Ransomware Detection,Machine Learning,Feature Engineering
AI Read Science
Must-Reading Tree
Example
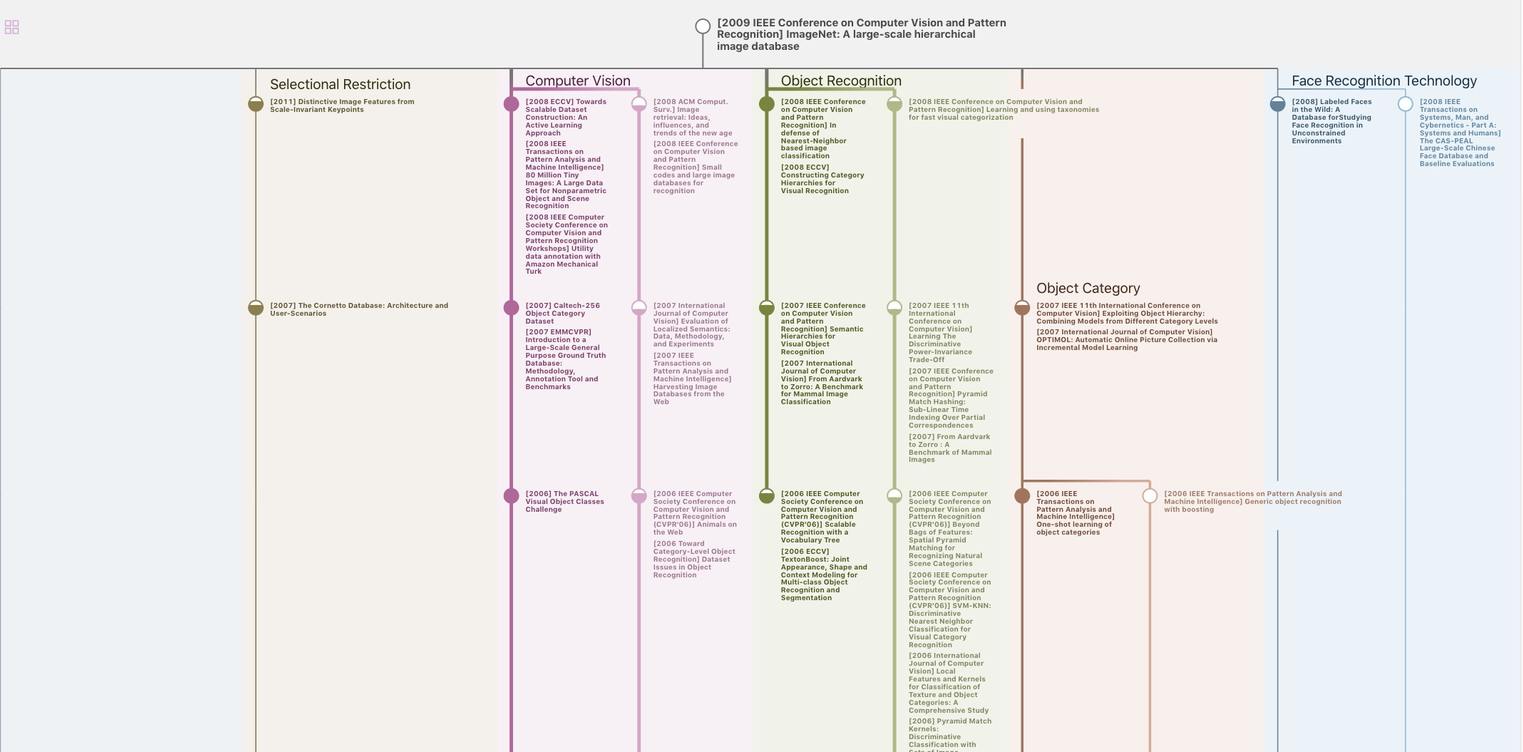
Generate MRT to find the research sequence of this paper
Chat Paper
Summary is being generated by the instructions you defined